Learning to Reassemble Shredded Documents
IEEE Transactions on Multimedia(2013)
摘要
In this paper, we address the problem of automatically assembling shredded documents. We propose a two-step algorithmic framework. First, we digitize each fragment of a given document and extract shape- and content-based local features. Based on these multimodal features, we identify pairs of corresponding points on all pairs of fragments using an SVM classifier. Each pair is considered a point of attachment for aligning the respective fragments. In order to restore the layout of the document, we create a document graph in which nodes represent fragments and edges correspond to alignments. We assign weights to the edges by evaluating the alignments using a set of inter-fragment constraints which take into account shape- and content-based information. Finally, we use an iterative algorithm that chooses the edge having the highest weight during each iteration. However, since selecting edges corresponds to combining groups of fragments and thus provides new evidence, we reevaluate the edge weights after each iteration. We quantitatively evaluate the effectiveness of our approach by conducting experiments on a novel dataset. It comprises a total of 120 pages taken from two magazines which have been shredded and annotated manually. We thus provide the means for a quantitative evaluation of assembly algorithms which, to the best of our knowledge, has not been done before.
更多查看译文
关键词
document image processing,feature extraction,graph theory,image classification,iterative methods,learning (artificial intelligence),support vector machines,SVM classifier,assembly algorithms,automatic shredded document assembling,content-based local feature extraction,document fragment digitization,document graph nodes,document layout restoration,edge selection,fragment alignment,graph edge weight assignment,interfragment constraints,iterative algorithm,magazine page annotation,magazine page shredding,multimodal features,quantitative evaluation,shape-based local feature extraction,shredded document reassembling,two-step algorithmic framework,Annotated dataset,document assembly,graph algorithm,supervised learning
AI 理解论文
溯源树
样例
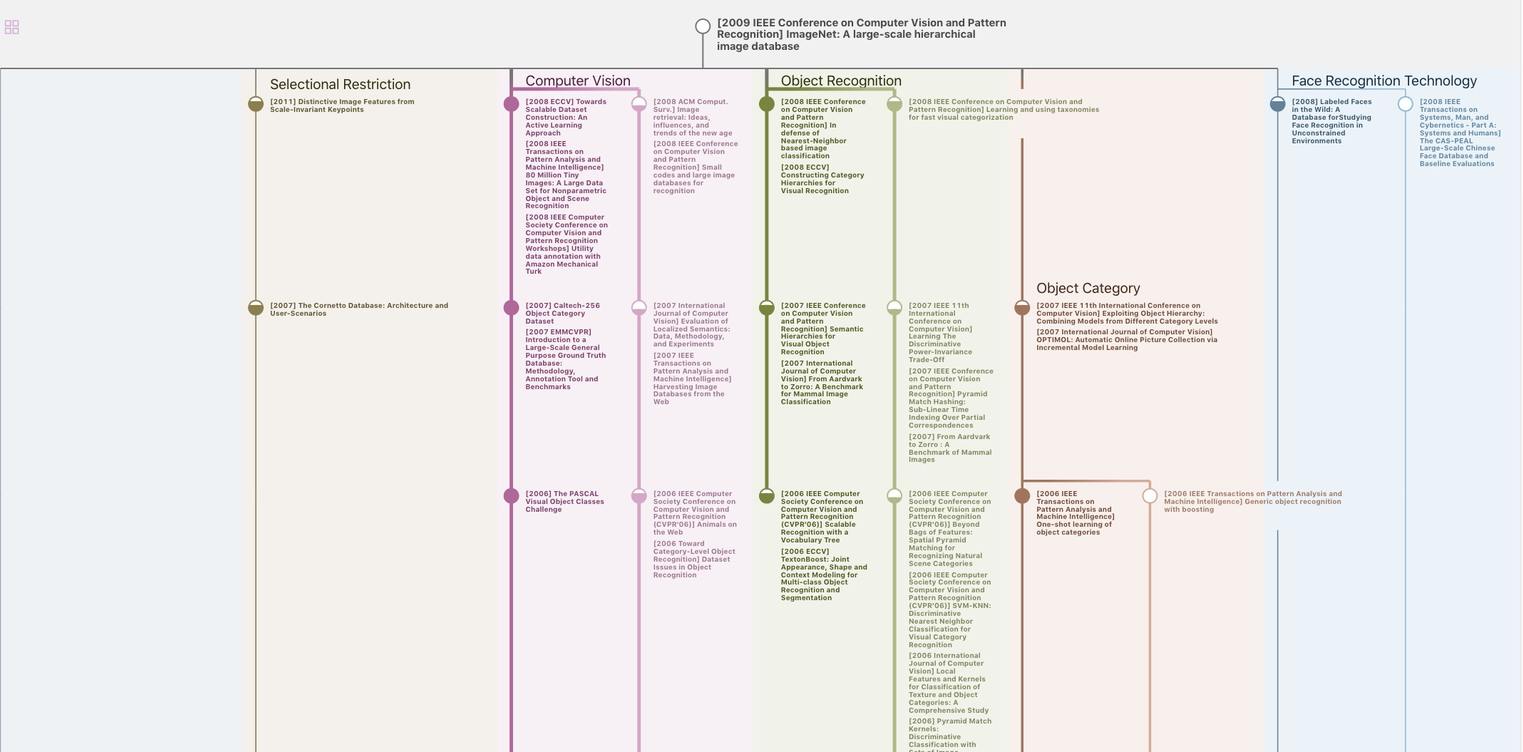
生成溯源树,研究论文发展脉络
Chat Paper
正在生成论文摘要