Maximum Likelihood Training Of Subspaces For Inverse Covariance Modeling
ICASSP '03). 2003 IEEE International Conference(2003)
摘要
Speech recognition systems typically use mixtures of diagonal Gaussians to model the acoustics. Using Gaussians with a more general covariance structure can give improved performance; EMLLT [1] and SPAM [2] models give improvements by restricting the inverse covariance to a linear/affine subspace spanned by rank one and full rank matrices respectively. In this paper we consider training these subspaces to maximize likelihood. For EMLLT ML training the subspace results in significant gains over the scheme proposed in [1]. For SPAM ML training of the subspace slightly improves performance over the method reported in [2]. For the same subspace size an EMLLT model is more efficient computationally than a SPAM model, while the SPAM model is more accurate. This paper proposes a hybrid method of structuring the inverse covariances that both has good accuracy and is computationally efficient.
更多查看译文
关键词
Gaussian processes,acoustic signal processing,covariance analysis,hidden Markov models,inverse problems,matrix algebra,maximum likelihood estimation,optimisation,speech recognition,EMLLT ML training,EMLLT model,ML parameter estimation,SPAM ML training,SPAM model,acoustics modelling,computationally efficient method,diagonal Gaussians,full rank matrix,hybrid method,inverse covariance,inverse covariance modeling,inverse covariances,linear/affine subspace,maximum likelihood linear transform,maximum likelihood training,numerical optimization,rank one matrix,speech recognition systems,subspace size,subspaces training
AI 理解论文
溯源树
样例
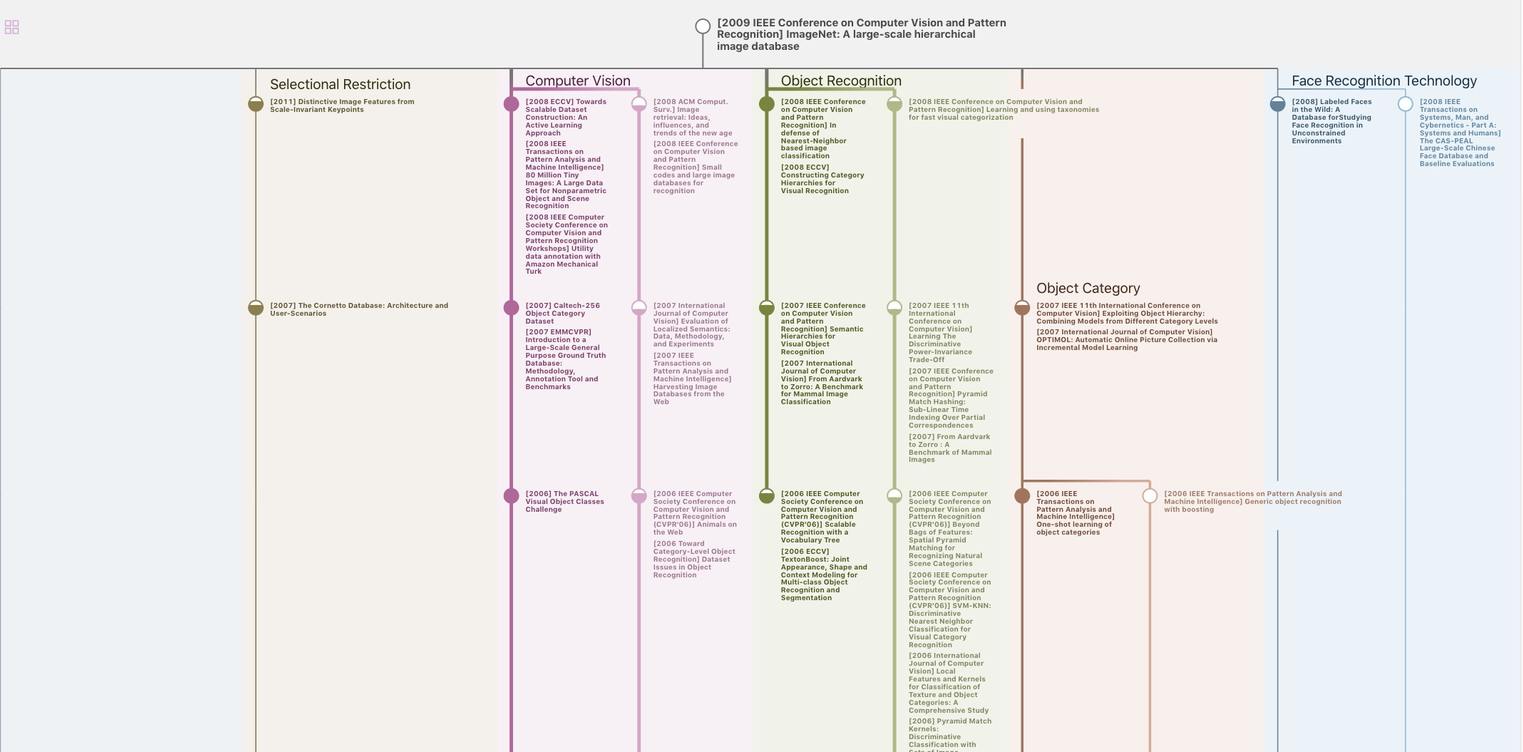
生成溯源树,研究论文发展脉络
Chat Paper
正在生成论文摘要