Autoregressive Models for Statistical Parametric Speech Synthesis
Audio, Speech, and Language Processing, IEEE Transactions(2013)
摘要
We propose using the autoregressive hidden Markov model (HMM) for speech synthesis. The autoregressive HMM uses the same model for parameter estimation and synthesis in a consistent way, in contrast to the standard approach to statistical parametric speech synthesis. It supports easy and efficient parameter estimation using expectation maximization, in contrast to the trajectory HMM. At the same time its similarities to the standard approach allow use of established high quality synthesis algorithms such as speech parameter generation considering global variance. The autoregressive HMM also supports a speech parameter generation algorithm not available for the standard approach or the trajectory HMM and which has particular advantages in the domain of real-time, low latency synthesis. We show how to do efficient parameter estimation and synthesis with the autoregressive HMM and look at some of the similarities and differences between the standard approach, the trajectory HMM and the autoregressive HMM. We compare the three approaches in subjective and objective evaluations. We also systematically investigate which choices of parameters such as autoregressive order and number of states are optimal for the autoregressive HMM.
更多查看译文
关键词
hidden Markov models,speech synthesis,statistical analysis,autoregressive HMM model,autoregressive hidden Markov model,autoregressive order,expectation maximization,global variance,high quality synthesis algorithms,parameter estimation,speech parameter generation algorithm,statistical parametric speech synthesis,Acoustic modeling,autoregressive hidden Markov model,autoregressive processes,hidden Markov models (HMMs),speech,statistical parametric speech synthesis
AI 理解论文
溯源树
样例
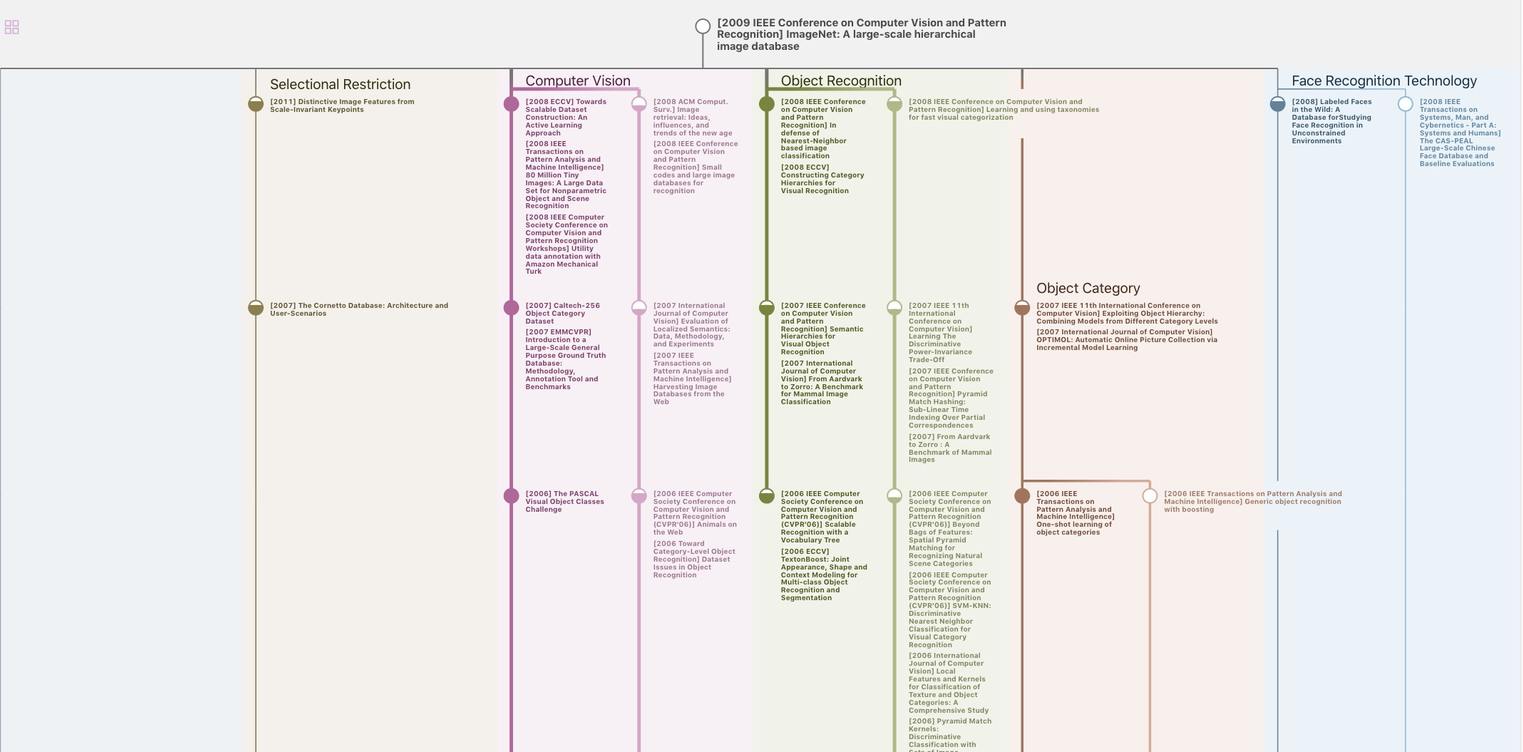
生成溯源树,研究论文发展脉络
Chat Paper
正在生成论文摘要