Learning the Information Divergence
IEEE Transactions on Pattern Analysis and Machine Intelligence(2015)
摘要
Information divergence that measures the difference between two nonnegative matrices or tensors has found its use in a variety of machine learning problems. Examples are Nonnegative Matrix/Tensor Factorization, Stochastic Neighbor Embedding, topic models, and Bayesian network optimization. The success of such a learning task depends heavily on a suitable divergence. A large variety of divergences have been suggested and analyzed, but very few results are available for an objective choice of the optimal divergence for a given task. Here we present a framework that facilitates automatic selection of the best divergence among a given family, based on standard maximum likelihood estimation. We first propose an approximated Tweedie distribution for the -divergence family. Selecting the best then becomes a machine learning problem solved by maximum likelihood. Next, we reformulate -divergence in terms of -divergence, which enables automatic selection of by maximum likelihood with reuse of the learning principle for -\div er\gence. Fur\the\rmore, we show \the con\nections between <\inl\i\ne-for\mula>$\gamma "> - and -divergences as well as Rényi- and -divergences, such that our automatic selection framework is extended to non-separable divergences. Experiments on both synthetic and real-world data demonstrate that our method can quite accurately select information divergence across different learning problems and various divergence families.
更多查看译文
关键词
information divergence,tweedie distribution,maximum likelihood,nonnegative matrix factorization,stochastic neighbor embedding
AI 理解论文
溯源树
样例
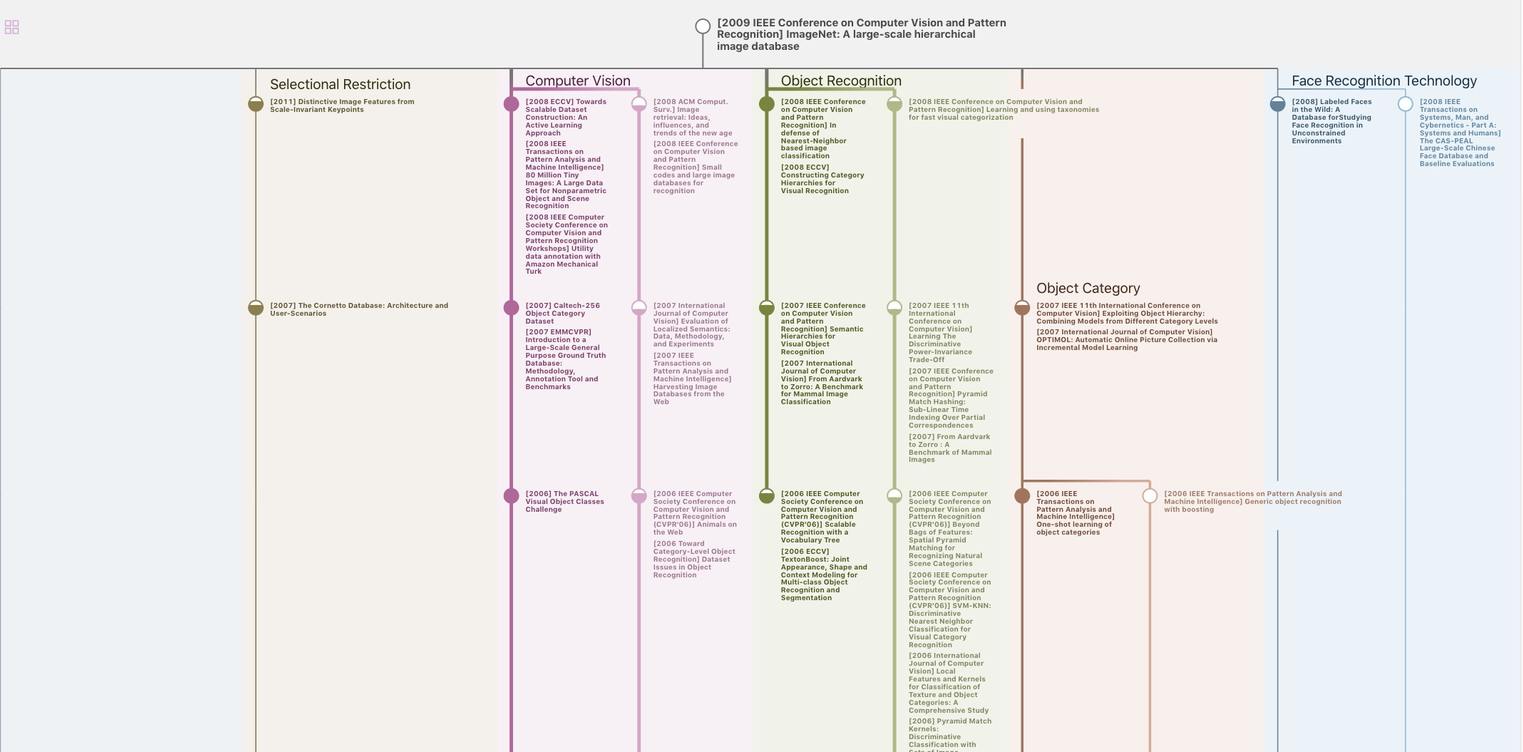
生成溯源树,研究论文发展脉络
Chat Paper
正在生成论文摘要