Detecting deception in Online Social Networks
ASONAM '14: Advances in Social Networks Analysis and Mining 2014 Beijing China August, 2014(2014)
摘要
Over the past decade Online Social Networks (OSNs) have been helping hundreds of millions of people develop reliable computer-mediated relations. However, many user profiles in OSNs contain misleading, inconsistent or false information. Existing studies have shown that lying in OSNs is quite widespread, often for protecting a user's privacy. In order for OSNs to continue expanding their role as a communication medium in our society, it is crucial for information posted on OSNs to be trusted. Here we define a set of analysis methods for detecting deceptive information about user genders in Twitter. In addition, we report empirical results with our stratified data set consisting of 174,600 Twitter profiles with a 50--50 breakdown between male and female users.
Our automated approach compares gender indicators obtained from different profile characteristics including first name, user name, and layout colors. We establish the overall accuracy of each indicator and the strength of all possible values for each indicator through extensive experimentations with our data set. We define male trending users and female trending users based on two factors, namely the overall accuracy of each characteristic and the relative strength of the value of each characteristic for a given user. We apply a Bayesian classifier to the weighted average of characteristics for each user. We flag for possible deception profiles that we classify as male or female in contrast with a self-declared gender that we obtain independently of Twitter profiles. Finally, we use manual inspections on a subset of profiles that we identify as potentially deceptive in order to verify the correctness of our predictions.
更多查看译文
关键词
Bayes methods,data privacy,pattern classification,social networking (online),50-50 breakdown,Bayesian classifier,Twitter profiles,deception detection,female trending users,first name,layout colors,male trending users,online social networks,reliable computer-mediated relations,user genders,user name,user privacy,weighted characteristics average,Deception detection,Twitter,gender classification,profile characteristics
AI 理解论文
溯源树
样例
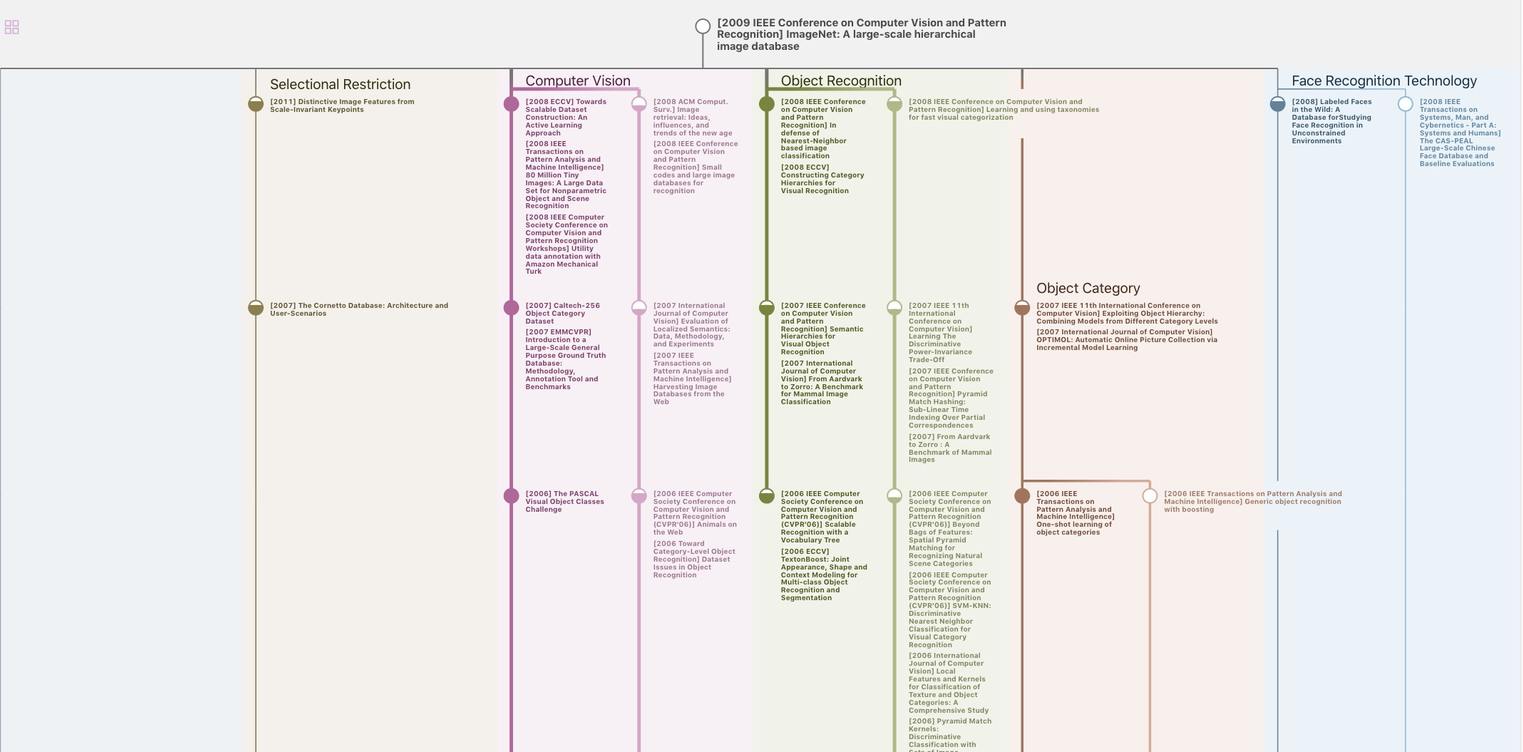
生成溯源树,研究论文发展脉络
Chat Paper
正在生成论文摘要