Reframing Continuous Input Attributes
Tools with Artificial Intelligence(2014)
摘要
Reuse of learnt knowledge is of critical importance in the majority of knowledge-intensive application areas, particularly because the operating context can be expected to vary from training to deployment. Dataset shift is a crucial example of this where training and testing datasets follow different distributions. However, most of the existing dataset shift solving algorithms need costly retraining operation and are not suitable to use the existing model. In this paper, we propose a new approach called reframing to handle dataset shift. The main objective of reframing is to build a model once and make it workable without retraining. We propose two efficient reframing algorithms to learn the optimal shift parameter values using only a small amount of labelled data available in the deployment. Thus, they can transform the shifted input attributes with the optimal parameter values and use the same existing model in several deployment environments without retraining. We have addressed supervised learning tasks both for classification and regression. Extensive experimental results demonstrate the efficiency and effectiveness of our approach compared to the existing solutions. In particular, we report the existence of dataset shift in two real-life datasets. These real-life unknown shifts can also be accurately modeled by our algorithms.
更多查看译文
关键词
data handling,learning (artificial intelligence),pattern classification,classification,dataset shift solving algorithms,knowledge-intensive application,learnt knowledge,optimal shift parameter values,reframing algorithms,reframing continuous input attributes,regression,supervised learning tasks,adaptation,classification,continuous attributes,dataset shift,machine learning,regression
AI 理解论文
溯源树
样例
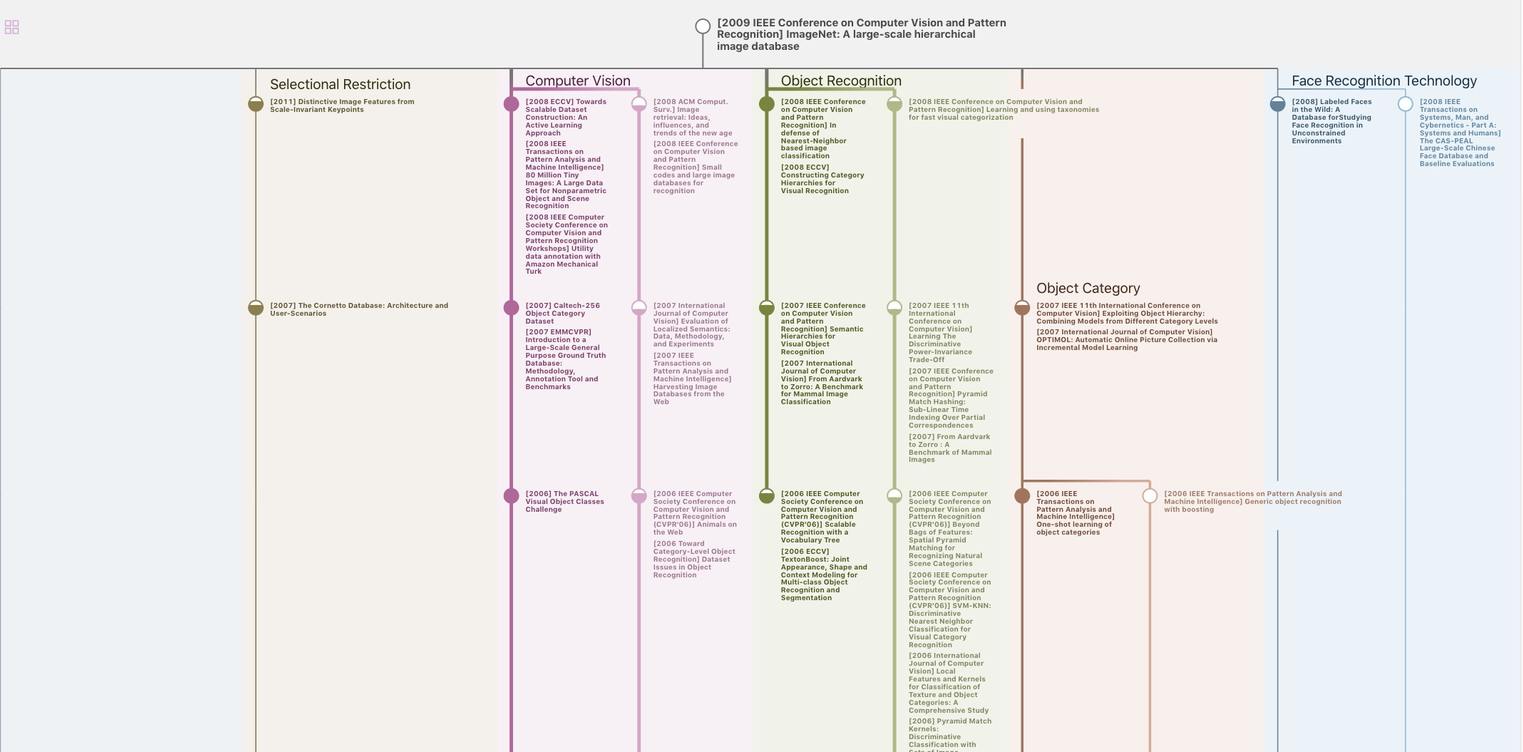
生成溯源树,研究论文发展脉络
Chat Paper
正在生成论文摘要