Crowdsourced intersection parameters: A generic approach for extraction and confidence estimation
Intelligent Vehicles Symposium(2014)
摘要
Digital maps within cars are not only the basis for navigation but also for advanced driver assistance systems. Therefore more and more up-to-date details about the environment of the vehicle are required which means that they have to be enriched with further attributes such as detailed representations of intersections. In the future we will be able to extract details of the environment out of the sensory data of connected cars. We present a generic approach for extracting multiple intersection parameters with the same method by analyzing logged data from a test fleet. Based on that a method for a feature based estimation of the confidence is introduced. The proposed approaches are applied in a completely automated process to estimate stop line positions and traffic flows at intersections with traffic lights. Altogether 203.701 traces of the test fleet were used for developing and testing. The performance of the method and the confidence estimation were analyzed using a ground truth, consisting of 108 stop line positions, which was derived from satellite images. The results show that the approach is fast and predictions with an absolute accuracy of 3.5m can be achieved. Hence the method is able to deliver valuable inputs for driver assistance systems.
更多查看译文
关键词
automobiles,data loggers,driver information systems,geographic information systems,parameter estimation,road traffic,automated stop line position estimation process,car navigation,crowdsourced intersection parameters,digital maps,driver assistance systems,environment detail extraction,feature-based confidence estimation,generic approach,ground truth,intersection representation,logged data analysis,multiple intersection parameter extraction,performance analysis,satellite images,sensory data,test fleet,traffic flow estimation,traffic light intersections,vehicle environment
AI 理解论文
溯源树
样例
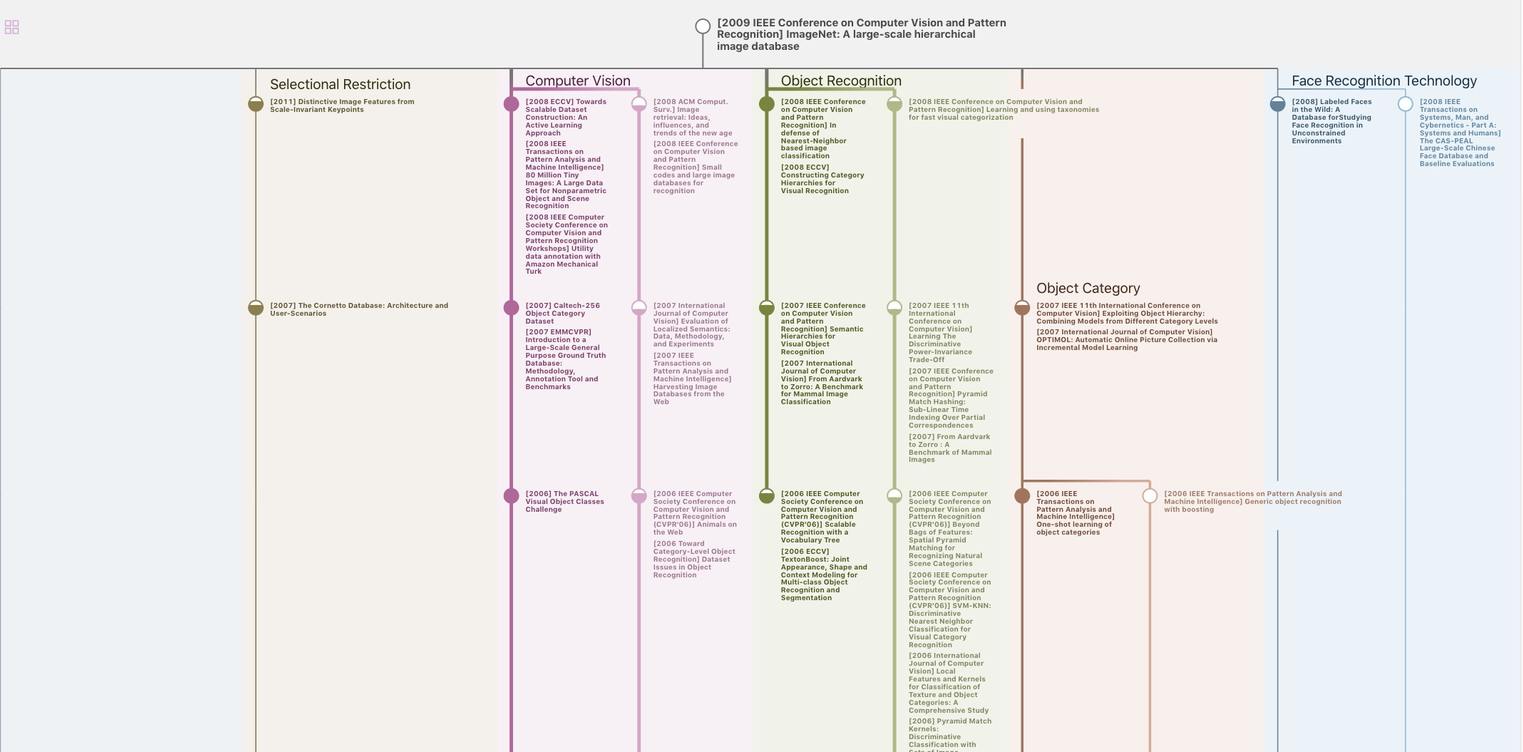
生成溯源树,研究论文发展脉络
Chat Paper
正在生成论文摘要