Multimodal Learning in Loosely-Organized Web Images
Computer Vision and Pattern Recognition(2014)
摘要
Photo-sharing websites have become very popular in the last few years, leading to huge collections of online images. In addition to image data, these websites collect a variety of multimodal metadata about photos including text tags, captions, GPS coordinates, camera metadata, user profiles, etc. However, this metadata is not well constrained and is often noisy, sparse, or missing altogether. In this paper, we propose a framework to model these \"loosely organized\" multimodal datasets, and show how to perform loosely-supervised learning using a novel latent Conditional Random Field framework. We learn parameters of the LCRF automatically from a small set of validation data, using Information Theoretic Metric Learning (ITML) to learn distance functions and a structural SVM formulation to learn the potential functions. We apply our framework on four datasets of images from Flickr, evaluating both qualitatively and quantitatively against several baselines.
更多查看译文
关键词
Web sites,learning (artificial intelligence),meta data,multimedia computing,random processes,support vector machines,Flickr,ITML,LCRF,distance function,information theoretic metric learning,latent conditional random field,loosely organized Web Images,loosely organized multimodal dataset,loosely supervised learning,multimodal learning,multimodal metadata collection,online image collection,photo sharing Website,potential function,structural SVM,graphical models,multimodal image modeling,object recognition,semi-supervised learning
AI 理解论文
溯源树
样例
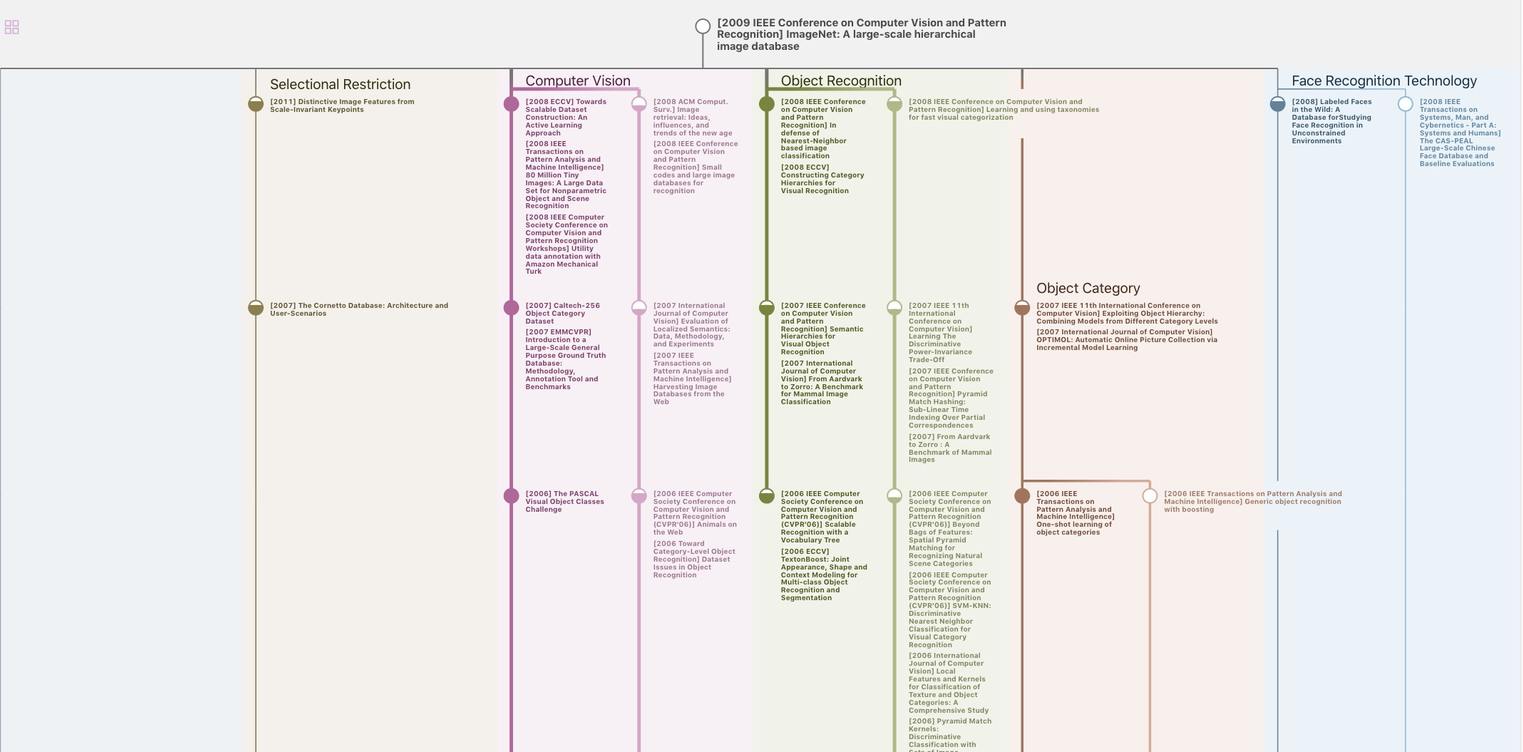
生成溯源树,研究论文发展脉络
Chat Paper
正在生成论文摘要