Fusing an ensemble of diverse prognostic life predictions
Big Sky, MT(2014)
摘要
Prognosis refers to the production of bounded estimates for the remaining useful life (RUL) of a component or system. Robust prognosis within well defined uncertainty bounds promises the ability to lower system sustainment costs and improve maintenance decision making. In ideal scenarios, the degradation of many assets is predictable from measurements taken during operation. These measurements, accompanied by appropriate knowledge, have been successfully used with various prognostic trending and prediction techniques to estimate the remaining useful life. However, there are scenarios where changes in operating environment or emerging fault modes can alter the degradation path an asset takes and thus invalidate previous predictions. One approach to overcoming these challenges is to develop prognostic techniques sensitive to change, however this sensitivity must be traded for robustness to imperfect degradation measurements. As an alternative, we develop multiple predictors, each with differing properties so that multiple estimates can be made based on different possible scenarios. A data fusion architecture is presented that combines multiple prognostic techniques weighted by their individual prediction uncertainty for the remaining useful life of an asset. The architecture is based on the concept of an ensemble Kalman Filter with an adaptive error co-variance parameter. The parameter is updated in real-time with the prediction variances produced by the set of prognostic predictions. Using real civil aerospace data, the technique is demonstrated to provide an amalgamated estimate of RUL to a fleet management operator. The developed architecture provides improved decision support to fleet maintainers in comparison to existing single prognostic technique methods.
更多查看译文
关键词
kalman filters,aerospace engineering,covariance analysis,decision making,maintenance engineering,remaining life assessment,sensor fusion,adaptive error covariance parameter,bounded estimates production,civil aerospace data,data fusion architecture,decision support,diverse prognostic life predictions,ensemble kalman filter,equipment health management,fault modes,fleet maintainers,fleet management operator,imperfect degradation measurements,maintenance decision making,prediction techniques,prognostic predictions,prognostic technique methods,prognostic techniques,prognostic trending,remaining useful life,system sustainment costs,data models,degradation,trajectory,mathematical model,noise,predictive models
AI 理解论文
溯源树
样例
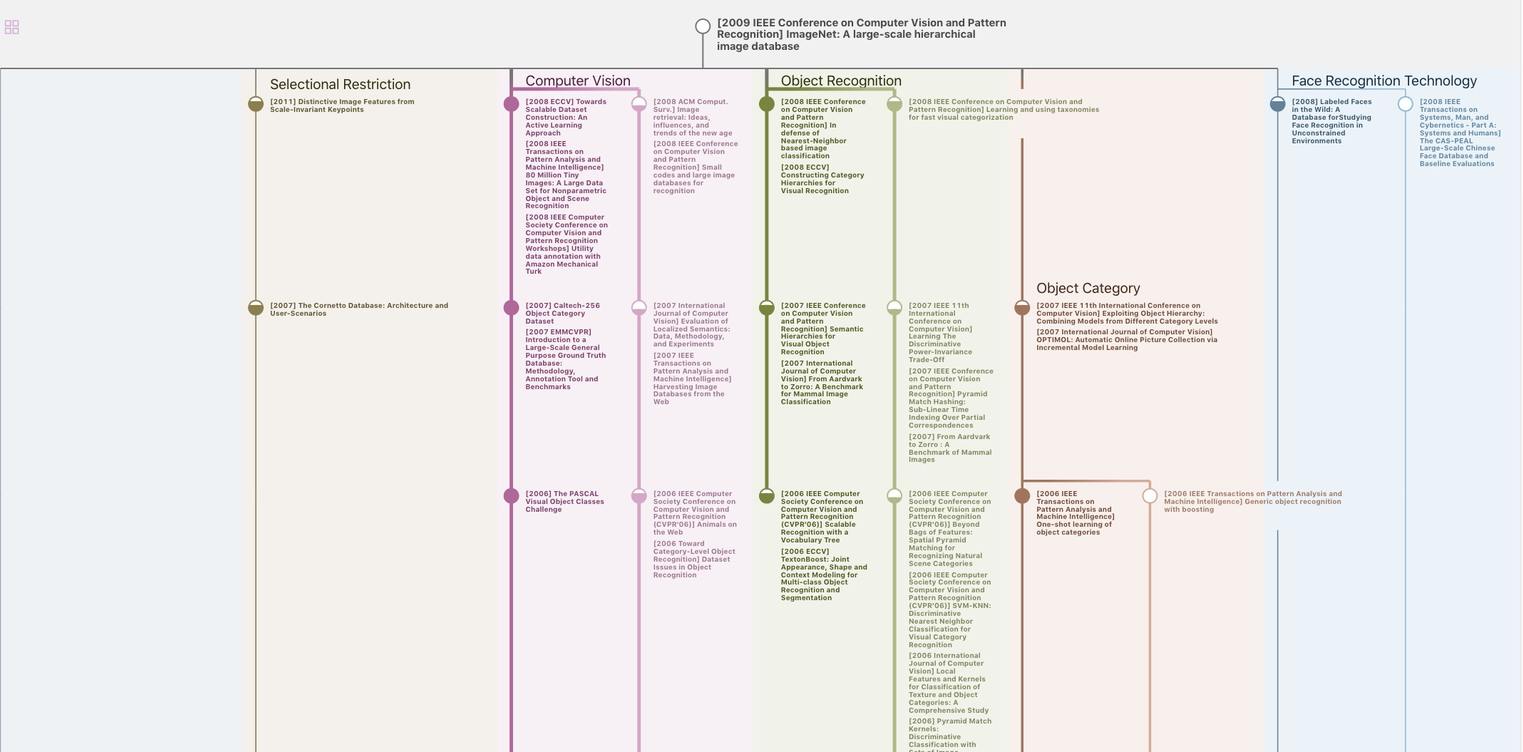
生成溯源树,研究论文发展脉络
Chat Paper
正在生成论文摘要