Audio concept classification with Hierarchical Deep Neural Networks
Signal Processing Conference(2017)
摘要
Audio-based multimedia retrieval tasks may identify semantic information in audio streams, i.e., audio concepts (such as music, laughter, or a revving engine). Conventional Gaussian-Mixture-Models have had some success in classifying a reduced set of audio concepts. However, multi-class classification can benefit from context window analysis and the discriminating power of deeper architectures. Although deep learning has shown promise in various applications such as speech and object recognition, it has not yet met the expectations for other fields such as audio concept classification. This paper explores, for the first time, the potential of deep learning in classifying audio concepts on User-Generated Content videos. The proposed system is comprised of two cascaded neural networks in a hierarchical configuration to analyze the short- and long-term context information. Our system outperforms a GMM approach by a relative 54%, a Neural Network by 33%, and a Deep Neural Network by 12% on the TRECVID-MED database.
更多查看译文
关键词
Gaussian processes,audio signal processing,audio streaming,content-based retrieval,learning (artificial intelligence),mixture models,multimedia databases,neural nets,signal classification,GMM,Gaussian mixture models,TRECVID-MED database,audio concept classification,audio streams,audio-based multimedia retrieval,cascaded neural networks,context information,context window analysis,hierarchical deep neural networks,multiclass classification,object recognition,semantic information,speech recognition,user-generated content videos,TRECVID,audio concepts classification,deep neural networks
AI 理解论文
溯源树
样例
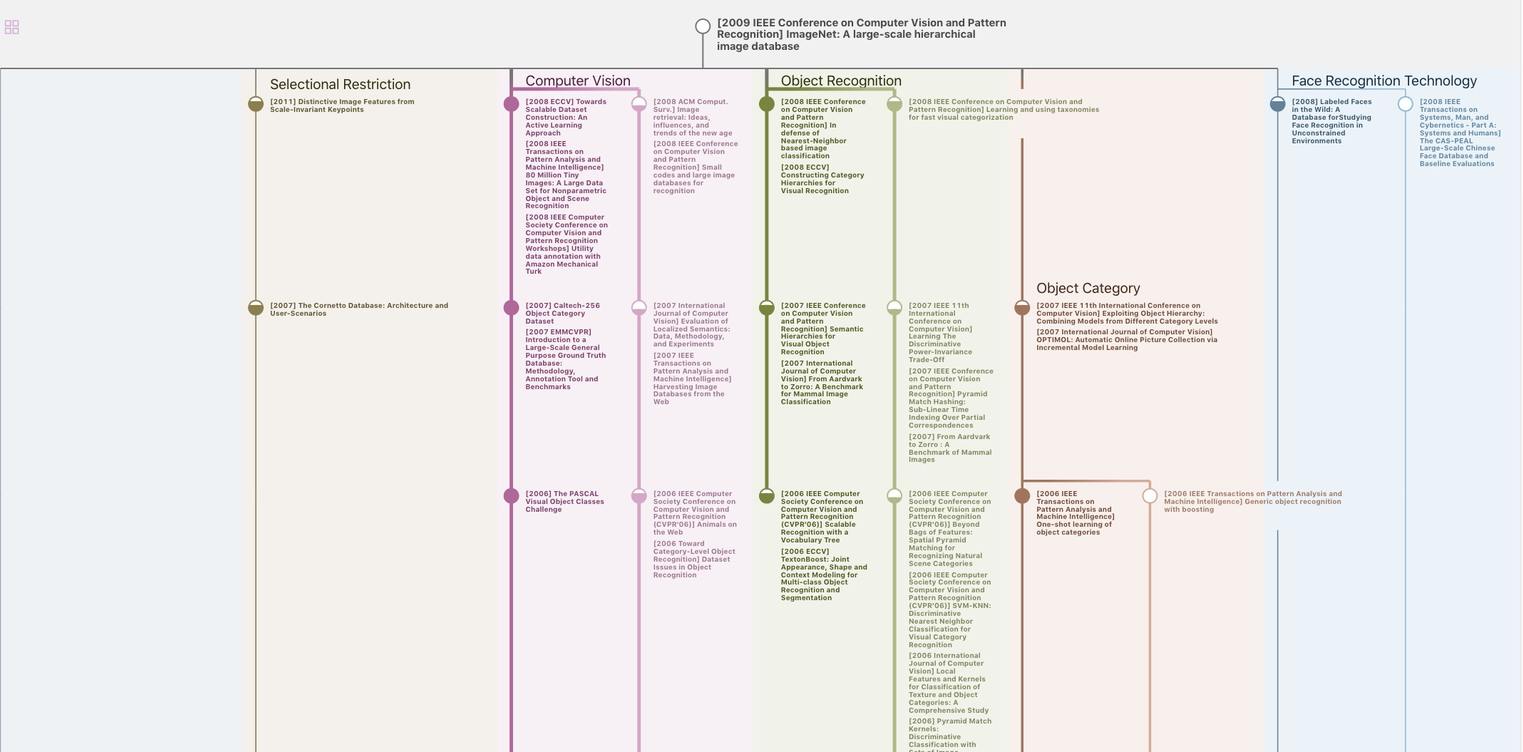
生成溯源树,研究论文发展脉络
Chat Paper
正在生成论文摘要