Improved audio features for large-scale multimedia event detection
Multimedia and Expo(2014)
摘要
In this paper, we present recent experiments on using Artificial Neural Networks (ANNs), a new “delayed” approach to speech vs. non-speech segmentation, and extraction of large-scale pooling feature (LSPF) for detecting “events” within consumer videos, using the audio channel only. A “event” is defined to be a sequence of observations in a video, that can be directly observed or inferred. Ground truth is given by a semantic description of the event, and by a number of example videos. We describe and compare several algorithmic approaches, and report results on the 2013 TRECVID Multimedia Event Detection (MED) task, using arguably the largest such research set currently available. The presented system achieved the best results in most audio-only conditions. While the overall finding is that MFCC features perform best, we find that ANN as well as LSP features provide complementary information at various levels of temporal resolution. This paper provides analysis of both low-level and high-level features, investigating their relative contributions to overall system performance.
更多查看译文
关键词
audio signal processing,feature extraction,neural nets,speech recognition,video signal processing,ANN,LSP features,LSPF,MED,MFCC features,TRECVID multimedia event detection,artificial neural networks,audio channel,audio feature improvement,consumer videos,delayed approach,high-level features,large-scale multimedia event detection,large-scale pooling feature extraction,low-level features,nonspeech segmentation,semantic description,speech segmentation,temporal resolution,acoustic event detection,computational acoustic scene analysis,multimedia retrieval
AI 理解论文
溯源树
样例
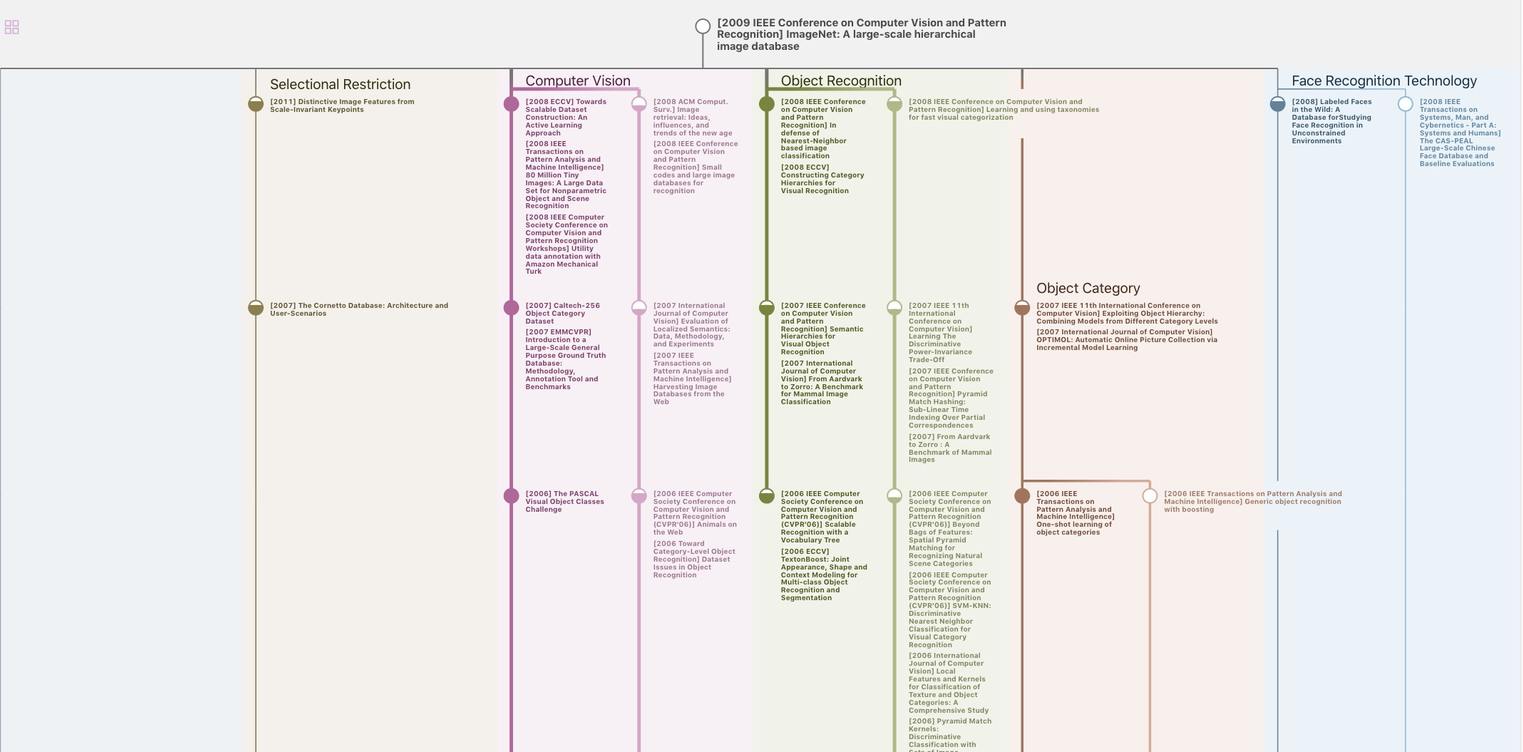
生成溯源树,研究论文发展脉络
Chat Paper
正在生成论文摘要