Participatory learning in the neurofuzzy short-term load forecasting
Computational Intelligence for Engineering Solutions(2014)
摘要
This paper presents a new approach for short-term load forecasting using the participatory learning paradigm. Participatory learning paradigm is a new training procedure that follows the human learning mechanism adopting an acceptance mechanism to determine which observation is used based upon its compatibility with the current beliefs. Here, participatory learning is used to train a class of hybrid neuro-fuzzy network to forecast 24-h daily energy consumption series of an electrical operation unit located at the Southeast region of Brazil. Experimental results show that the neurofuzzy approach with participatory learning requires less computational effort, is more robust, and more efficient than alternative neural methods. The approach is particularly efficient when training data reflects anomalous load conditions or contains spurious measurements. Comparisons with alternative approaches suggested in the literature are also included to show the effectiveness of participatory learning.
更多查看译文
关键词
fuzzy neural nets,learning (artificial intelligence),load forecasting,power engineering computing,Brazil,acceptance mechanism,daily energy consumption series,electrical operation unit,human learning mechanism,hybrid neuro-fuzzy network,neuro-fuzzy short-term load forecasting,participatory learning,training procedure
AI 理解论文
溯源树
样例
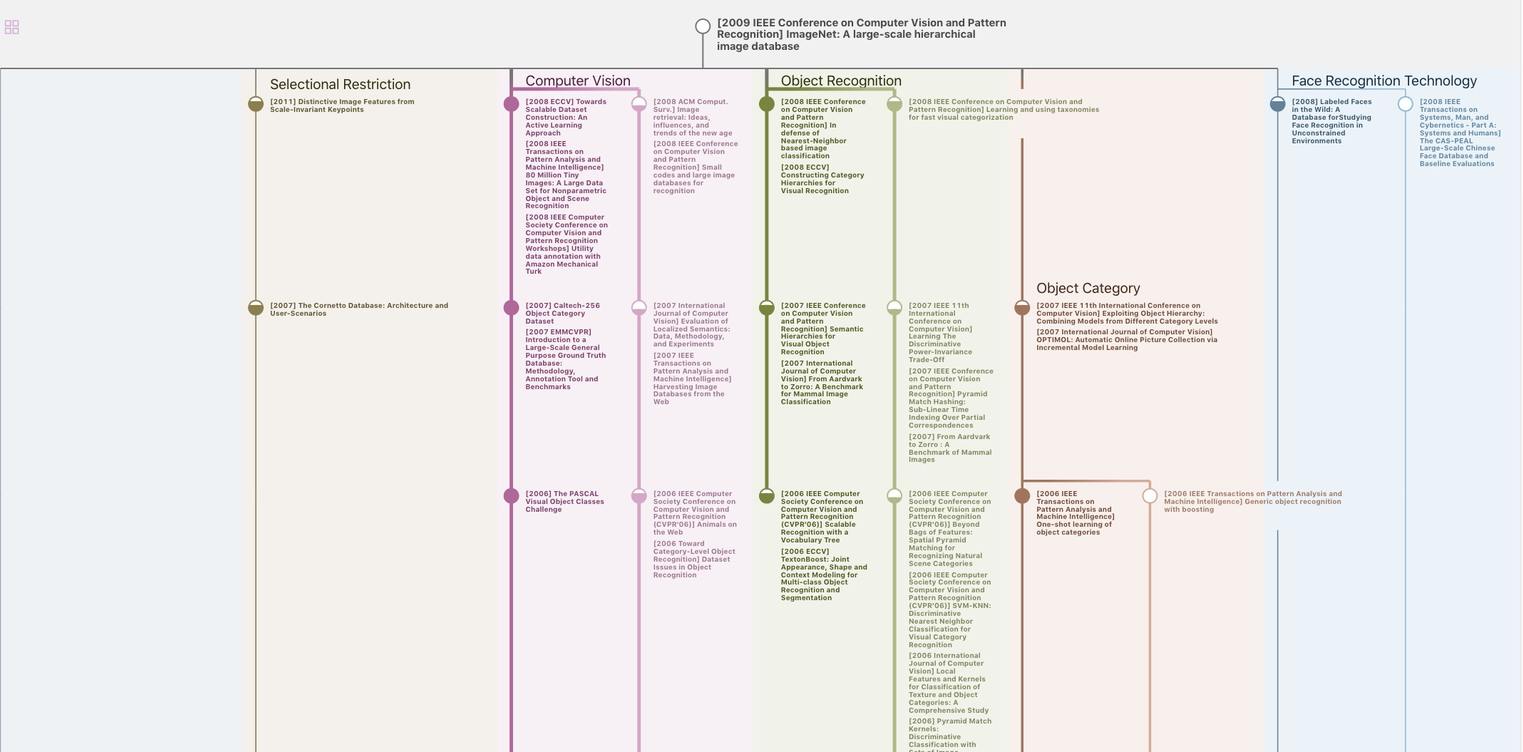
生成溯源树,研究论文发展脉络
Chat Paper
正在生成论文摘要