Active Exploration in Networks: Using Probabilistic Relationships for Learning and Inference.
CIKM '14: 2014 ACM Conference on Information and Knowledge Management Shanghai China November, 2014(2014)
摘要
Many interesting domains in machine learning can be viewed as networks, with relationships (e.g., friendships) connecting items (e.g., individuals). The Active Exploration (AE) task is to identify all items in a network with a desired trait (i.e., positive labels) given only partial information about the network. The AE process iteratively queries for labels or network structure within a limited budget; thus, accurate predictions prior to making each query is critical to maximizing the number of positives gathered. However, the targeted AE query process produces partially observed networks that can create difficulties for predictive modeling. In particular, we demonstrate that these partial networks can exhibit extreme label correlation bias, which makes it difficult for conventional relational learning methods to accurately estimate relational parameters. To overcome this issue, we model the joint distribution of possible edges and labels to improve learning and inference. Our proposed method, Probabilistic Relational Expectation Maximization (PR-EM), is the first AE approach to accurately learn the complex dependencies between attributes, labels, and structure to improve predictions. PR-EM utilizes collective inference over the missing relationships in the partial network to jointly infer unknown item traits. Further, we develop a linear inference algorithm to facilitate efficient use of PR-EM in large networks. We test our approach on four real world networks, showing that AE with PR-EM gathers significantly more positive items compared to state-of-the-art methods.
更多查看译文
AI 理解论文
溯源树
样例
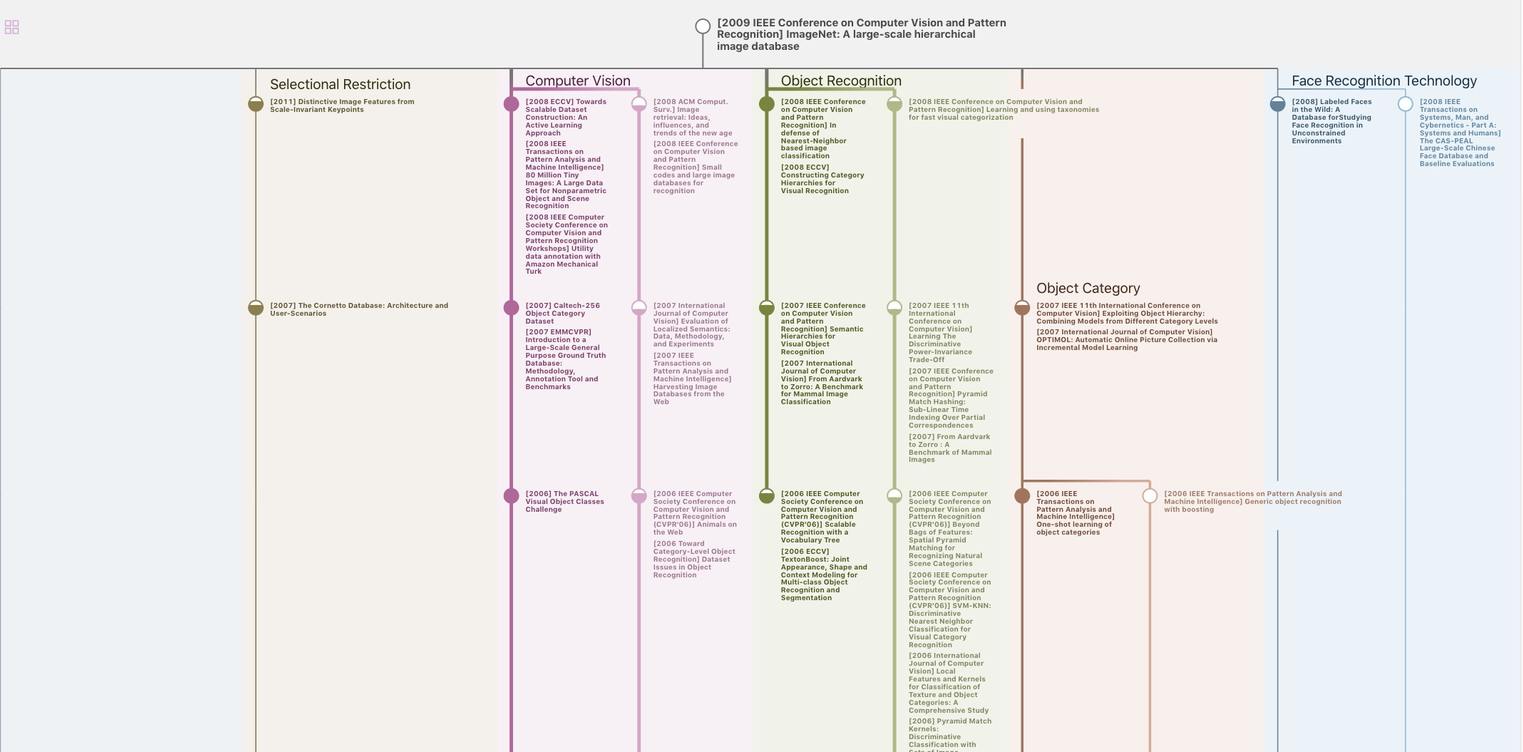
生成溯源树,研究论文发展脉络
Chat Paper
正在生成论文摘要