Component Detection in Directed Networks.
CIKM '14: 2014 ACM Conference on Information and Knowledge Management Shanghai China November, 2014(2014)
摘要
Community detection has been one of the fundamental problems in network analysis. Results from community detection (for example, grouping of products by latent category) can also serve as information nuggets to other business applications, such as product recommendation or taxonomy building. Because several real networks are naturally directed, e.g. World Wide Web, some recent studies proposed algorithms for detecting various types of communities in a directed network. However, few of them considered that nodes play two different roles, source and terminal, in a directed network.
In this paper, we adopt a novel concept of communities, directional community, and propose a new algorithm based on Markov Clustering to detect directional communities. We then first compare our algorithm, Dual R-MCL, on synthetic networks with two recent algorithms also designed for detecting directional communities. We show that Dual R-MCL can detect directional communities with significantly higher accuracy and 3x to 25x faster than the two other algorithms. Second, we compare a set of directed network community detection algorithms on a one-day Twitter interaction network and demonstrate that Dual R-MCL can generate clusters more correctly matched to hashtags. Finally, we exhibit our algorithm's capacity to identify directional communities from product description networks, where nodes are otherwise not directly connected.
Results indicate that directional communities exist in real networks, and Dual R-MCL can effectively detect these directional communities. We believe it will enable the discovery of interesting components in a diverse types of networks where existing methods cannot, and it manifests strong application values.
更多查看译文
AI 理解论文
溯源树
样例
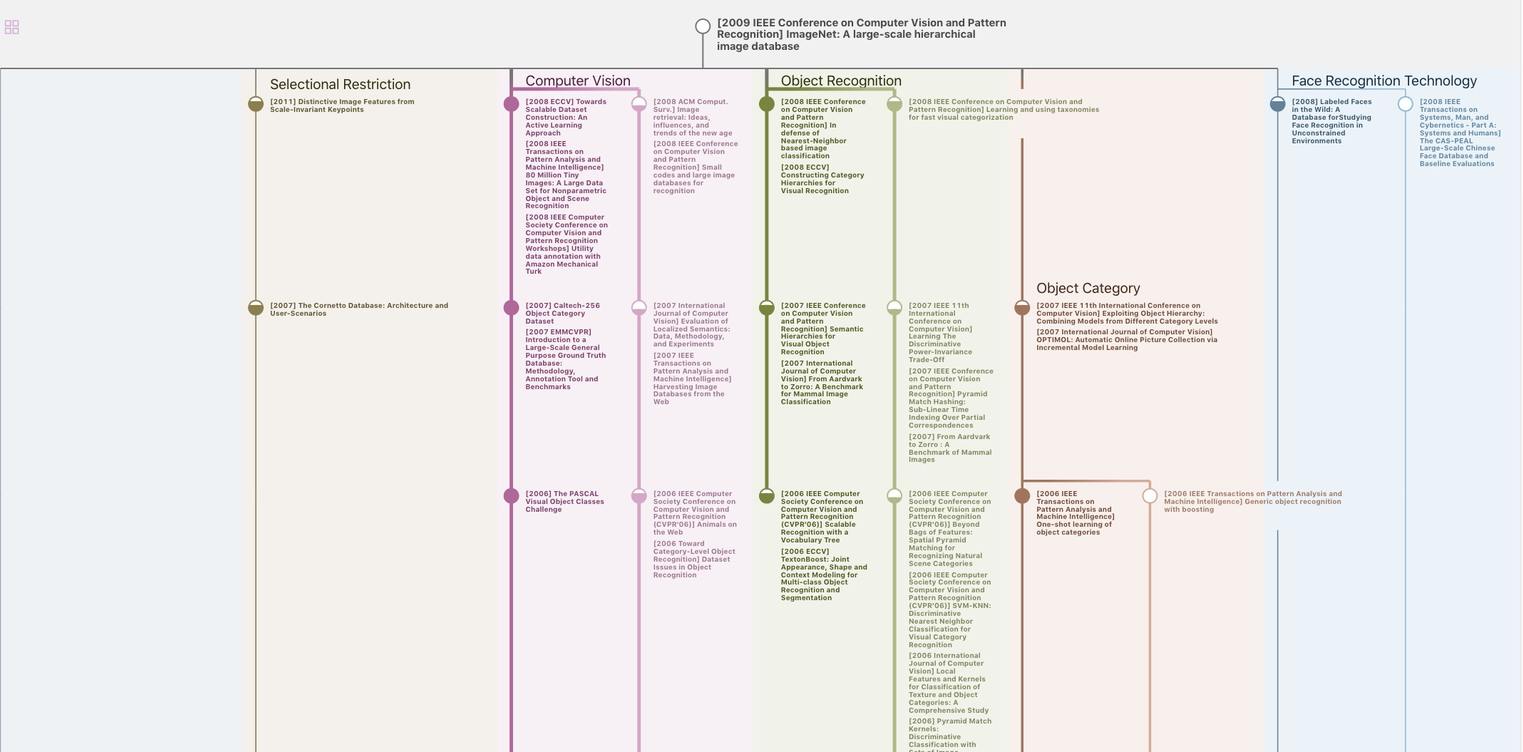
生成溯源树,研究论文发展脉络
Chat Paper
正在生成论文摘要