Maximizing Multi-scale Spatial Statistical Discrepancy.
CIKM '14: 2014 ACM Conference on Information and Knowledge Management Shanghai China November, 2014(2014)
摘要
Detecting anomalous events from spatial data has important applications in real world. The spatial scan statistic methods are popular in this area. With maximizing the spatial statistical discrepancy by comparing observed data with a given baseline data distribution, significant spatial overdensity and underdensity can be detected. In reality, the spatial discrepancy is often irregularly shaped and has a structure of multiple spatial scales. However, a large-scale discrepancy pattern may not be significant when conducting fine granularity analysis. Meanwhile, local irregular boundaries of a maximized discrepancy cannot be well approximated with a coarse granularity analysis. Existing methods mostly work either on a fixed granularity, or with a regularly shaped scanning window. Thus, they have difficulties in characterizing such flexible spatial discrepancies. To solve the problem, in this paper we propose a novel discrepancy maximization algorithm, RefineScan. A grid hierarchy encoding multi-scale information is employed, making the algorithm capable of maximizing spatial discrepancies with multi-scale structures and irregular shapes. Experiments on a wide range of datasets demonstrate the advantages of RefineScan over the state-of-the-art algorithms: It always finds the largest discrepancy scores and remarkably better characterizes multi-scale discrepancy boundaries. Theoretical and empirical analyses also show that RefineScan has a moderate computational complexity and a good scalability.
更多查看译文
AI 理解论文
溯源树
样例
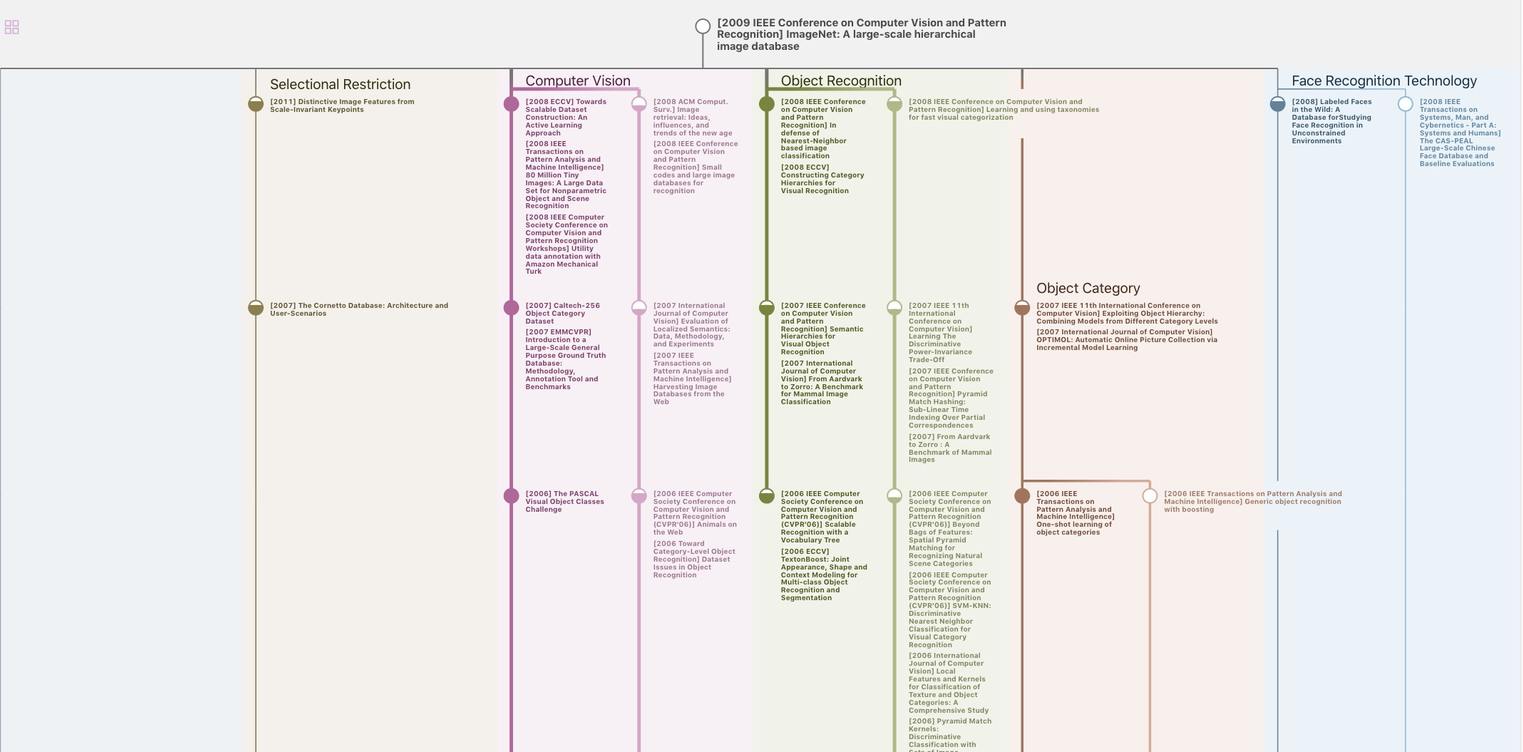
生成溯源树,研究论文发展脉络
Chat Paper
正在生成论文摘要