Multiple-manifolds Discriminant Analysis for Facial Expression Recognition from Local Patches Set.
Lecture Notes in Artificial Intelligence(2015)
摘要
In this paper, a novel framework is proposed for feature extraction and classification of facial expression recognition, namely multiple manifold discriminant analysis (MMDA), which assumes samples of different expressions reside on different manifolds, thereby learning multiple projection matrices from training set. In particular, MMDA first incorporates five local patches, including the regions of left and right eyes, mouth and left and right cheeks from each training sample to form a new training set, and then learns projection matrix from each expression so that maximizes the manifold margins among different expressions and minimizes the manifold distances of the same expression. A key feature of MMDA is that it can extract the discriminative information of expression-specific for classification rather than that of subject-specific, leading to a robust performance in practical applications. Our experiments on Cohn-Kanade and JAFFE databases demonstrate that MMDA can effectively enhance the discriminant power of the extracted expression features.
更多查看译文
关键词
Manifold learning,Facial expression recognition,Local patches,Multiple manifolds discriminant analysis
AI 理解论文
溯源树
样例
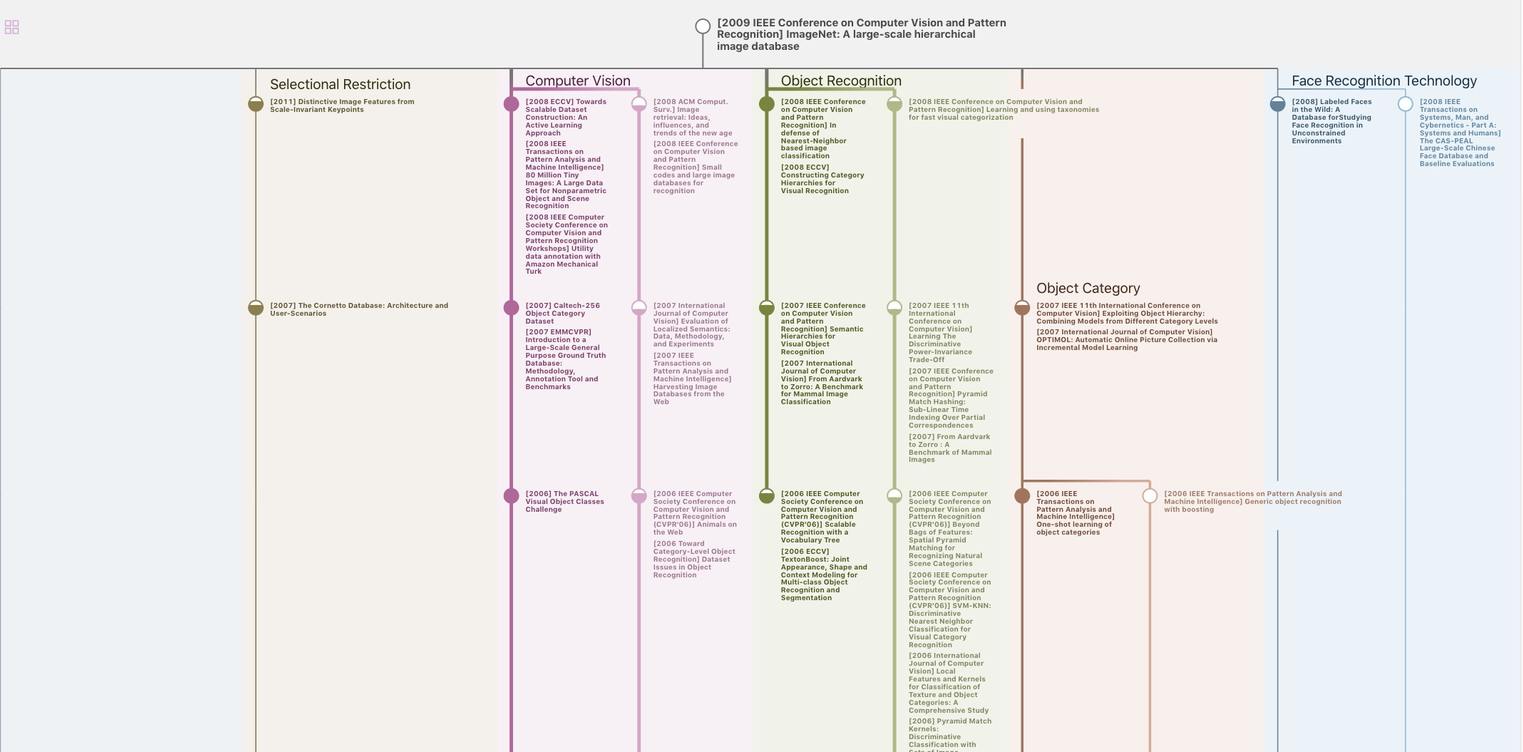
生成溯源树,研究论文发展脉络
Chat Paper
正在生成论文摘要