“Can you really trust that seed?”: Reducing the impact of seed noise in personalized PageRank
ASONAM(2014)
摘要
Network based recommendation systems leverage the topology of the underlying graph and the current user context to rank objects in the database. Random-walk based techniques, such as PageRank, encode the structure of the graph in the form of a transition matrix of a stochastic process from which the significances of the nodes in the graph are inferred. Personalized PageRank (PPR) techniques complement this with a seed node set which serves as the personalization context. In this paper, we note (and experimentally show) that PPR algorithms that do not differentiate among the seed nodes may not properly rank nodes in situations where the seed set is incomplete and/or noisy. To tackle this problem, we propose alternative robust personalized PageRank (RPR) strategies, which are insensitive to noise in the set of seed nodes and in which the rankings are not overly biased towards the seed nodes. In particular, we show that novel teleportation discounting and seed-set maximal PPR techniques help eliminate harmful bias of individual seed nodes and provide effective seed differentiation to lead to more accurate rankings.
更多查看译文
关键词
personalized pagerank techniques,ppr algorithms,seed noise,user context,robust personalized pagerank strategies,teleportation discounting,random-walk based techniques,underlying graph topology,matrix algebra,recommender systems,seed node set,network based recommendation systems,transition matrix,stochastic process,topology,graph theory,seed-set maximal ppr techniques,personalization context,vectors,motion pictures,teleportation,noise measurement,robustness
AI 理解论文
溯源树
样例
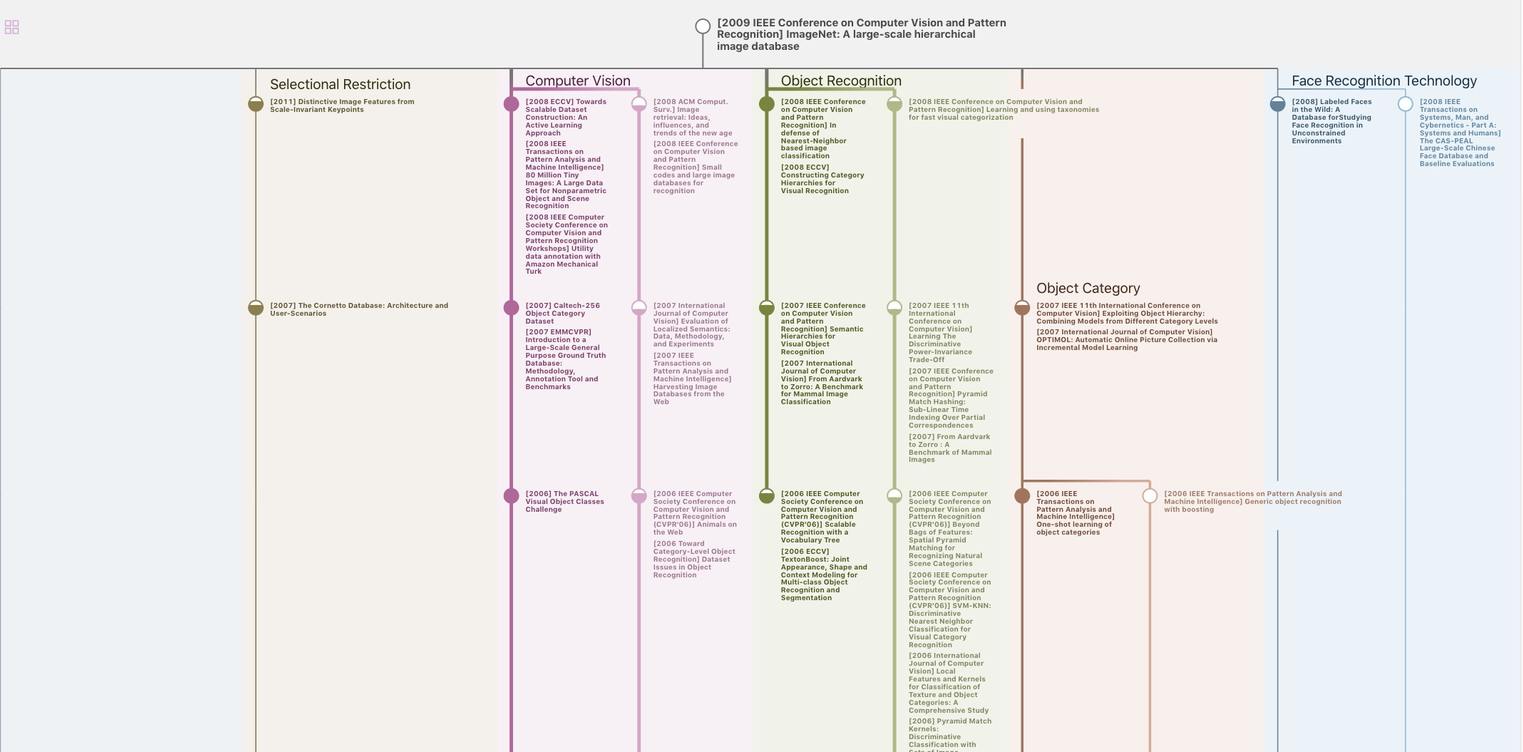
生成溯源树,研究论文发展脉络
Chat Paper
正在生成论文摘要