Efficient algorithm for ranking of nodes' importance in information dissemination
ASONAM(2014)
摘要
Identifying nodes that play important roles in network dynamics in large scale complex networks is crucial for both characterizing the network and resource management. Under the viral marketing setting, Diffusion Centrality (DC) estimates the influential power of an individual. For the transport and physics communities, a node is considered important in Markov centrality (MC) if it can be quickly reached from the other nodes. Because these networks could contain millions of nodes, any ranking algorithm must have low time requirements to be practically useful. In this paper, we show that both metrics are strongly correlated, and we present a new method to enable fast estimation of the two metrics for large scale networks. The new approach is further validated empirically by using both real and synthetic networks. Our results refined the intuition that the influential power of an individual is largely governed by the local topology, rather than the mere number of contacts (node degree) alone. This allows us to better characterize the properties of the nodes that affect the outcome of the two centrality metrics.
更多查看译文
关键词
node importance ranking,centralities,random walk,large scale complex networks,local topology,diffusion centrality,complex networks,resource management,information dissemination,viral marketing setting,markov centrality,dc,ranking algorithm,mc,graph theory,centrality metrics,network dynamics,markov processes,measurement,barium,erbium,correlation
AI 理解论文
溯源树
样例
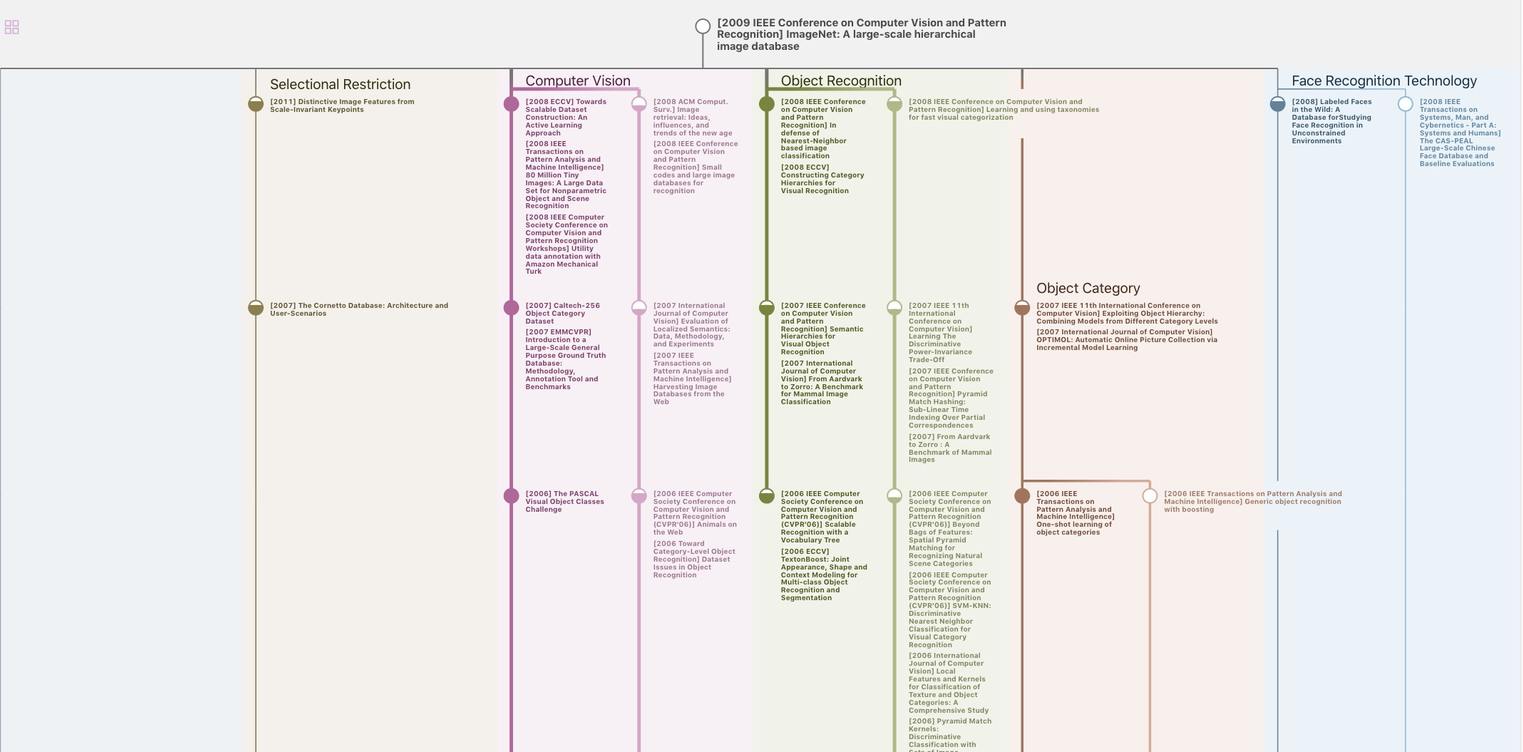
生成溯源树,研究论文发展脉络
Chat Paper
正在生成论文摘要