Direct adaptation of hybrid DNN/HMM model for fast speaker adaptation in LVCSR based on speaker code
ICASSP(2014)
摘要
Recently an effective fast speaker adaptation method using discriminative speaker code (SC) has been proposed for the hybrid DNN-HMM models in speech recognition [1]. This adaptation method depends on a joint learning of a large generic adaptation neural network for all speakers as well as multiple small speaker codes using the standard back-propagation algorithm. In this paper, we propose an alternative direct adaptation in model space, where speaker codes are directly connected to the original DNN models through a set of new connection weights, which can be estimated very efficiently from all or part of training data. As a result, the proposed method is more suitable for large scale speech recognition tasks since it eliminates the time-consuming training process to estimate another adaptation neural networks. In this work, we have evaluated the proposed direct SC-based adaptation method in the large scale 320-hr Switchboard task. Experimental results have shown that the proposed SC-based rapid adaptation method is very effective not only for small recognition tasks but also for very large scale tasks. For example, it has shown that the proposed method leads to up to 8% relative reduction in word error rate in Switchboard by using only a very small number of adaptation utterances per speaker (from 10 to a few dozens). Moreover, the extra training time required for adaptation is also significantly reduced from the method in [1].
更多查看译文
关键词
speaker code,adaptation method,lvcsr,speech recognition,speaker codes,hybrid dnn-hmm,word error rate,direct sc-based adaptation method,fast speaker adaptation,deep neural network (dnn),backpropagation,switchboard task,speaker recognition,speech codecs,standard backpropagation algorithm,hybrid dnn-hmm model,direct adaptation,discriminative speaker code,time 320 hr,neural nets,adaptation neural networks,training data,speech,neural networks,hidden markov models
AI 理解论文
溯源树
样例
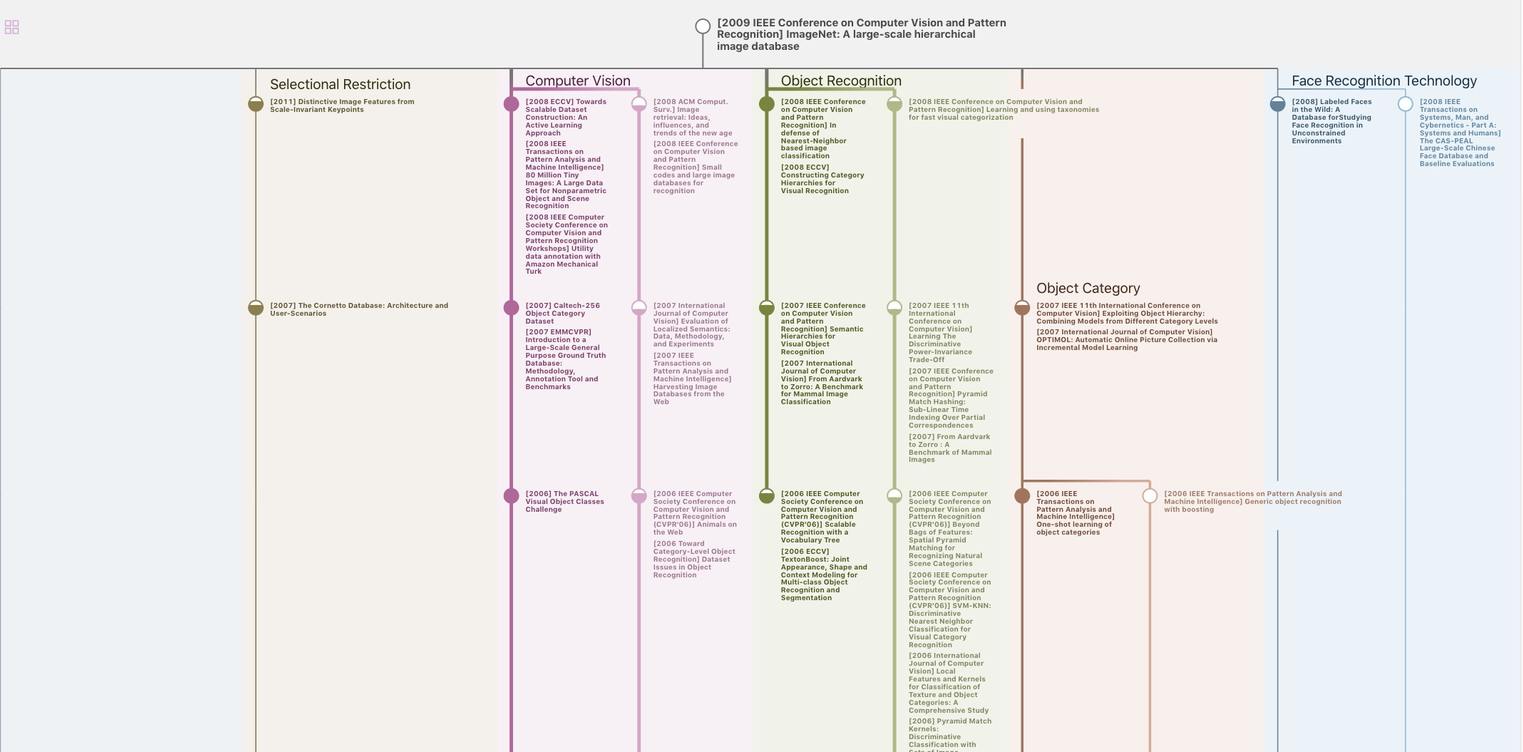
生成溯源树,研究论文发展脉络
Chat Paper
正在生成论文摘要