Accurate Object Detection with Joint Classification-Regression Random Forests
CVPR(2014)
摘要
In this paper, we present a novel object detection approach that is capable of regressing the aspect ratio of objects. This results in accurately predicted bounding boxes having high overlap with the ground truth. In contrast to most recent works, we employ a Random Forest for learning a template-based model but exploit the nature of this learning algorithm to predict arbitrary output spaces. In this way, we can simultaneously predict the object probability of a window in a sliding window approach as well as regress its aspect ratio with a single model. Furthermore, we also exploit the additional information of the aspect ratio during the training of the Joint Classification-Regression Random Forest, resulting in better detection models. Our experiments demonstrate several benefits: (i) Our approach gives competitive results on standard detection benchmarks. (ii) The additional aspect ratio regression delivers more accurate bounding boxes than standard object detection approaches in terms of overlap with ground truth, especially when tightening the evaluation criterion. (iii) The detector itself becomes better by only including the aspect ratio information during training.
更多查看译文
关键词
detection models,classification-regression random forests,learning (artificial intelligence),regression analysis,object probability,standard detection benchmarks,object detection approach,bounding boxes prediction,image recognition,template-based model,arbitrary output spaces prediction,object detection,prediction theory,evaluation criterion,objects aspect ratio regression,aspect ratio information,learning algorithm,sliding window approach,probability,detectors,vegetation,predictive models,learning artificial intelligence,training data
AI 理解论文
溯源树
样例
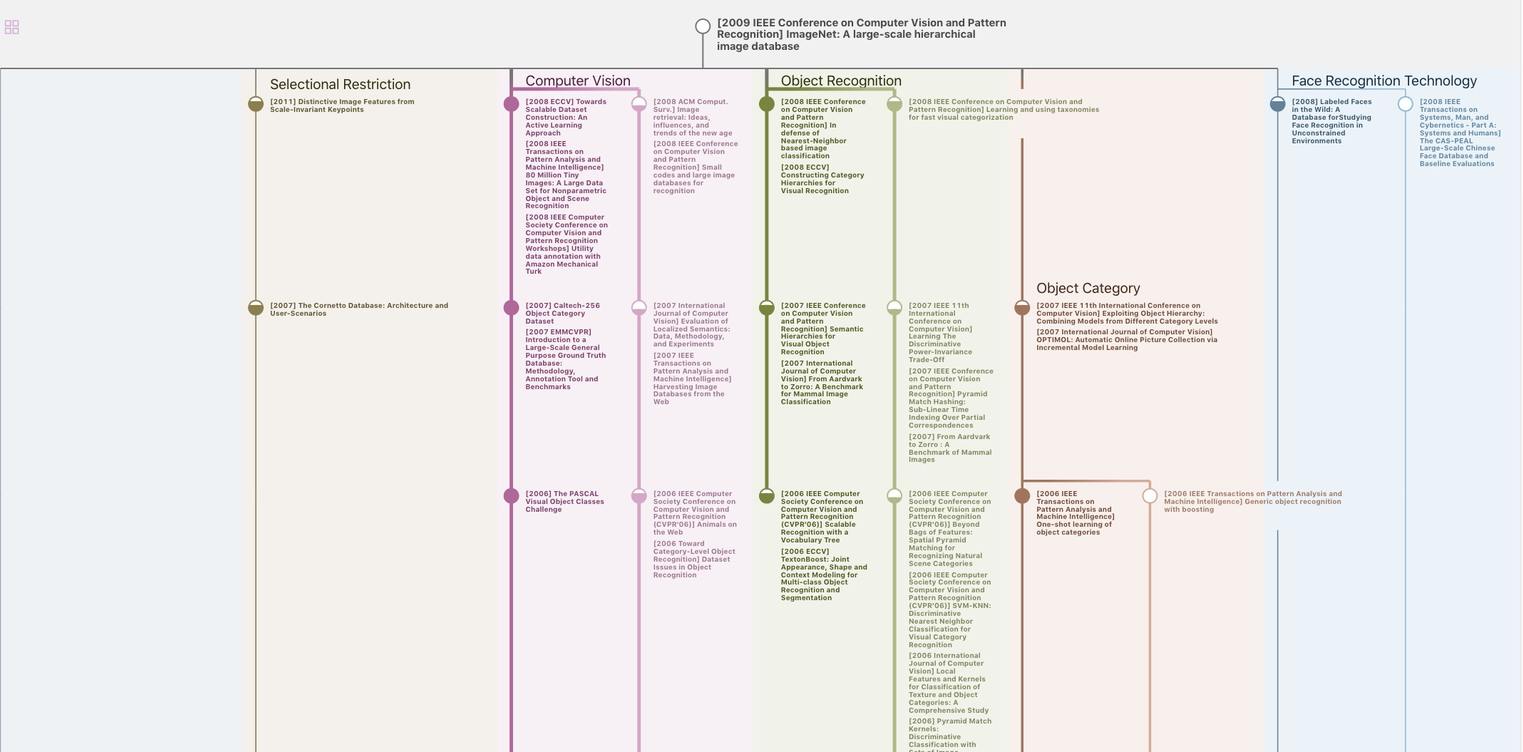
生成溯源树,研究论文发展脉络
Chat Paper
正在生成论文摘要