Sparse Dictionary Learning for Edit Propagation of High-Resolution Images
CVPR(2014)
摘要
We introduce a method of sparse dictionary learning for edit propagation of high-resolution images or video. Previous approaches for edit propagation typically employ a global optimization over the whole set of image pixels, incurring a prohibitively high memory and time consumption for high-resolution images. Rather than propagating an edit pixel by pixel, we follow the principle of sparse representation to obtain a compact set of representative samples (or features) and perform edit propagation on the samples instead. The sparse set of samples provides an intrinsic basis for an input image, and the coding coefficients capture the linear relationship between all pixels and the samples. The representative set of samples is then optimized by a novel scheme which maximizes the KL-divergence between each sample pair to remove redundant samples. We show several applications of sparsity-based edit propagation including video recoloring, theme editing, and seamless cloning, operating on both color and texture features. We demonstrate that with a sample-to-pixel ratio in the order of 0.01%, signifying a significant reduction on memory consumption, our method still maintains a high-degree of visual fidelity.
更多查看译文
关键词
seamless cloning,image resolution,video recoloring,theme editing,edit propagation,kl-divergence,texture features,compressed sensing,sparse dictionary learning,color features,high-resolution images,high-resolution video,coding coefficients,image pixels,image texture,sparse representation,optimization,mathematical model,kl divergence,dictionaries,encoding,cloning
AI 理解论文
溯源树
样例
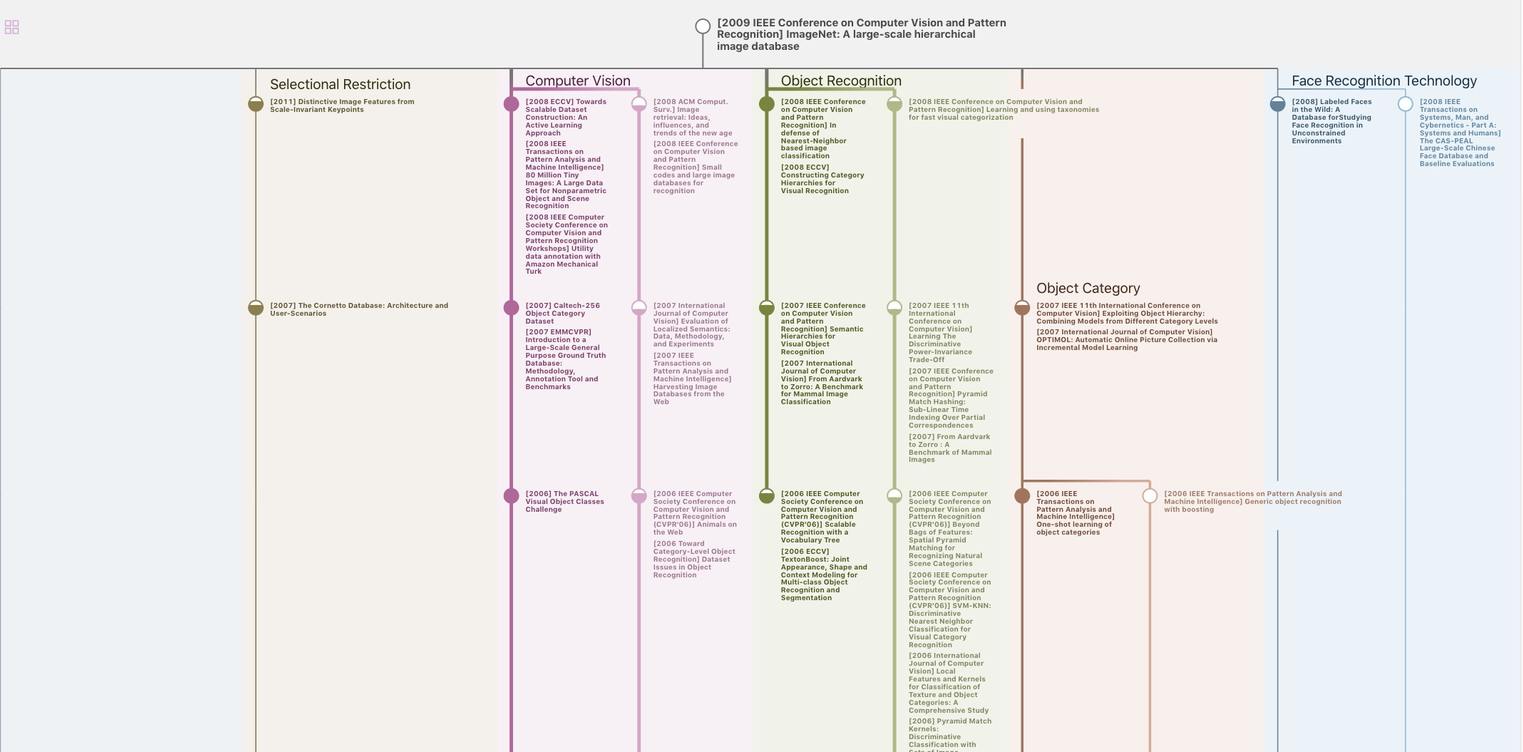
生成溯源树,研究论文发展脉络
Chat Paper
正在生成论文摘要