Driven By Food: Modeling Geographic Choice
WSDM(2015)
摘要
In this work we study the dynamics of geographic choice, i.e., how users choose one from a set of objects in a geographic region. We postulate a model in which an object is selected from a slate of candidates with probability that depends on how far it is (distance) and how many closer alternatives exist (rank). Under a discrete choice formulation, we argue that there exists a factored form in which unknown functions of rank and distance may be combined to produce an accurate estimate of the likelihood that a user will select each alternative. We then learn these hidden functions and show that each can be closely approximated by an appropriately parameterized lognormal, even though the respective marginals look quite different. We give a theoretical justification to support the presence of lognormal distributions.We then apply this framework to study restaurant choices in map search logs. We show that a four-parameter model based on combinations of lognormals has excellent performance at predicting restaurant choice, even compared to baseline models with access to the full (densely parameterized) marginal distribution for rank and distance. Finally, we show how this framework can be extended to simultaneously learn a per-restaurant quality score representing the residual likelihood of choice after distance and rank have been accounted for. We show that, compared to a per-place score that predicts likelihood without factoring out rank and distance, our score is a significantly better predictor of user quality judgments.
更多查看译文
AI 理解论文
溯源树
样例
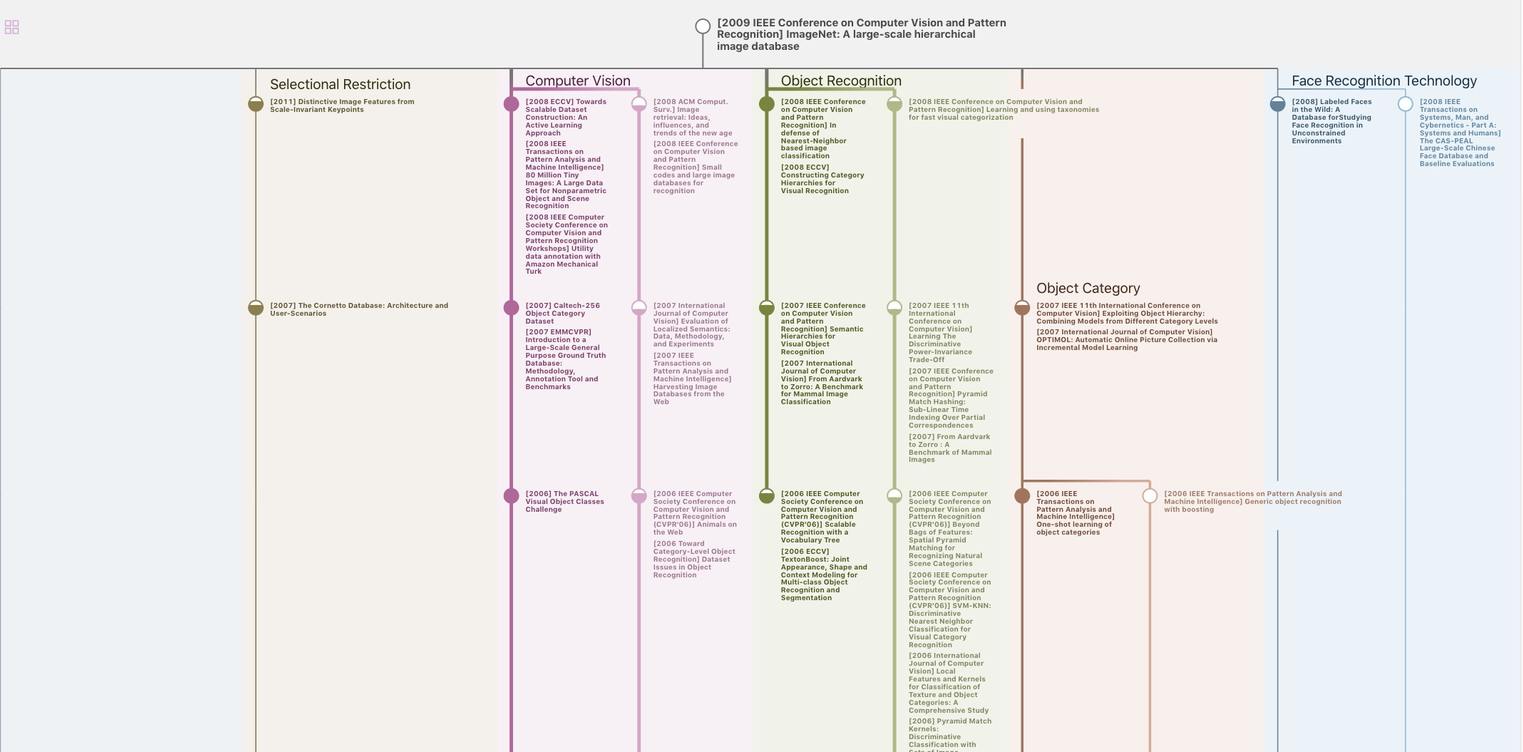
生成溯源树,研究论文发展脉络
Chat Paper
正在生成论文摘要