Exponential Random Graph Estimation Under Differential Privacy
KDD '14: The 20th ACM SIGKDD International Conference on Knowledge Discovery and Data Mining New York New York USA August, 2014(2014)
摘要
The effective analysis of social networks and graph-structured data is often limited by the privacy concerns of individuals whose data make up these networks. Differential privacy offers individuals a rigorous and appealing guarantee of privacy. But while differentially private algorithms for computing basic graph properties have been proposed, most graph modeling tasks common in the data mining community cannot yet be carried out privately.In this work we propose algorithms for privately estimating the parameters of exponential random graph models (ERGMs). We break the estimation problem into two steps: computing private sufficient statistics, then using them to estimate the model parameters. We consider specific alternating statistics that are in common use for ERGM models and describe a method for estimating them privately by adding noise proportional to a high-confidence bound on their local sensitivity. In addition, we propose an estimation algorithm that considers the noise distribution of the private statistics and offers better accuracy than performing standard parameter estimation using the private statistics.
更多查看译文
关键词
Differential privacy,Exponential random graph model
AI 理解论文
溯源树
样例
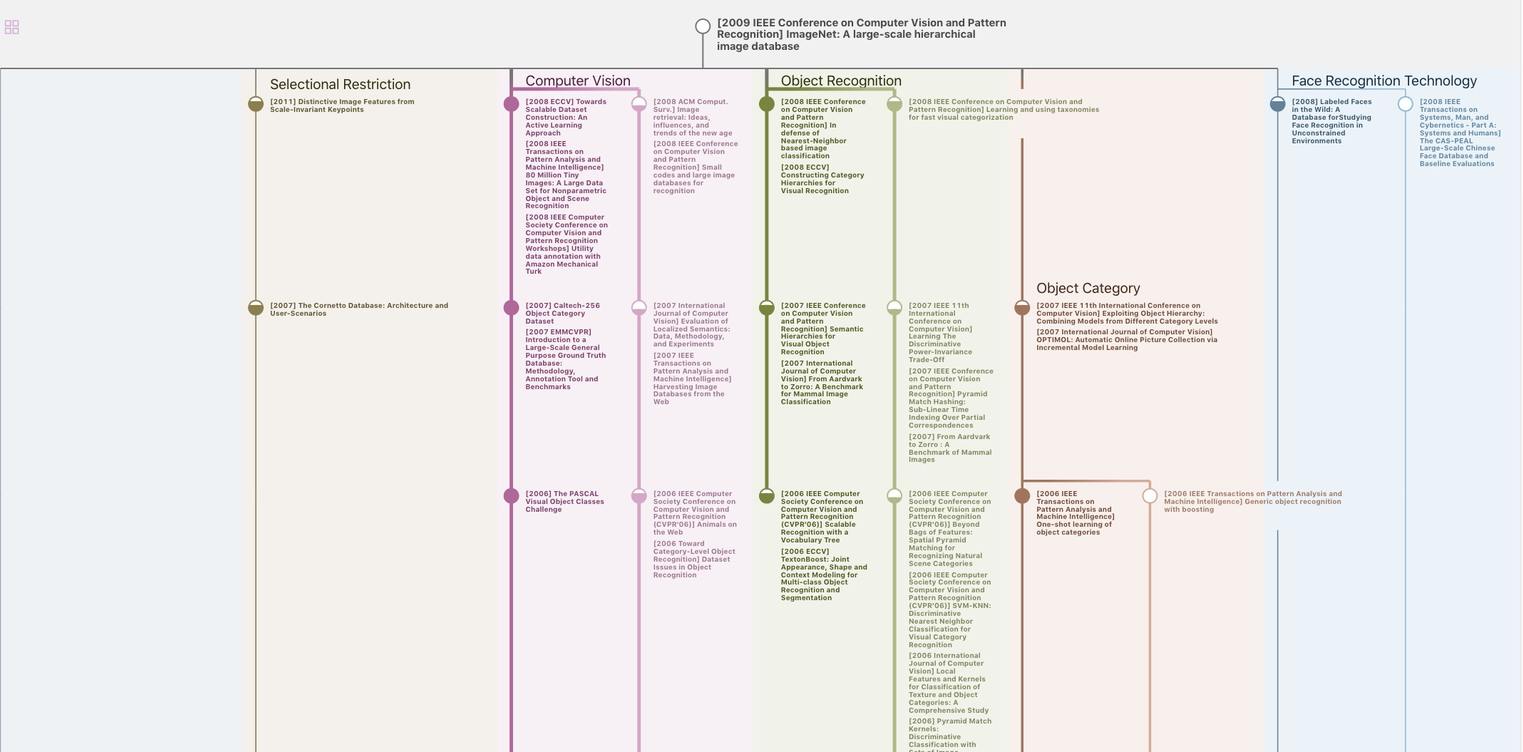
生成溯源树,研究论文发展脉络
Chat Paper
正在生成论文摘要