Modeling Impression Discounting In Large-Scale Recommender Systems
KDD '14: The 20th ACM SIGKDD International Conference on Knowledge Discovery and Data Mining New York New York USA August, 2014(2014)
摘要
Recommender systems have become very important for many online activities, such as watching movies, shopping for products, and connecting with friends on social networks. User behavioral analysis and user feedback (both explicit and implicit) modeling are crucial for the improvement of any online recommender system. Widely adopted recommender systems at LinkedIn such as "People You May Know" and "Endorsements" are evolving by analyzing user behaviors on impressed recommendation items.In this paper, we address modeling impression discounting of recommended items, that is, how to model user's no-action feedback on impressed recommended items. The main contributions of this paper include (1) large-scale analysis of impression data from LinkedIn and KDD Cup; (2) novel anti-noise regression techniques. and its application to learn four different impression discounting functions including linear decay, inverse decay, exponential decay, and quadratic decay; (3) applying these impression discounting functions to LinkedIn's "People You May Know" and "Endorsements" recommender systems.
更多查看译文
关键词
Impression discounting,Recommender system
AI 理解论文
溯源树
样例
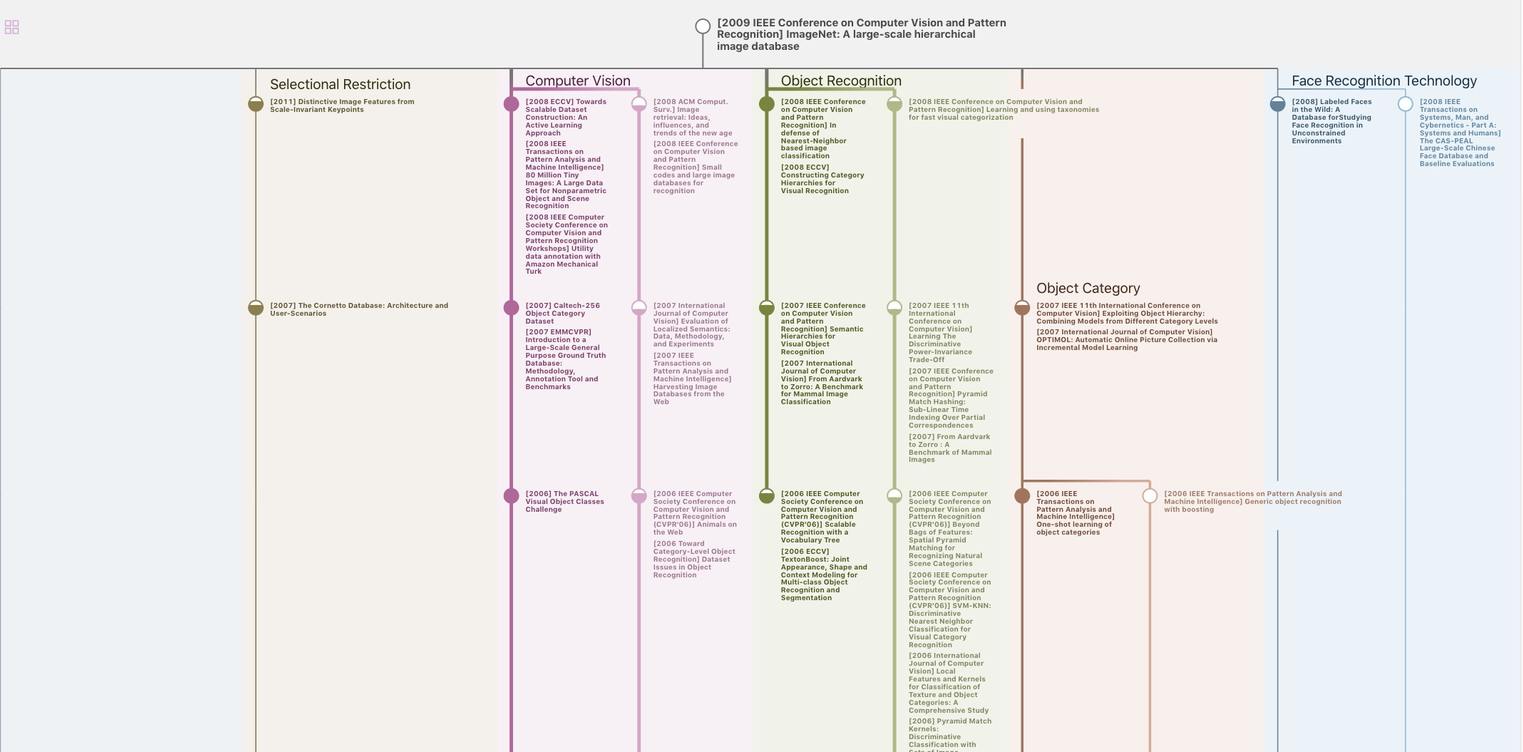
生成溯源树,研究论文发展脉络
Chat Paper
正在生成论文摘要