Comparing Person-And Process-Centric Strategies For Obtaining Quality Data On Amazon Mechanical Turk
CHI '15: CHI Conference on Human Factors in Computing Systems Seoul Republic of Korea April, 2015(2015)
摘要
In the past half-decade, Amazon Mechanical Turk has radically changed the way many scholars do research. The availability of a massive, distributed, anonymous crowd of individuals willing to perform general human-intelligence micro-tasks for micro-payments is a valuable resource for researchers and practitioners. This paper addresses the challenges of obtaining quality annotations for subjective judgment oriented tasks of varying difficulty. We design and conduct a large, controlled experiment (N=68,000) to measure the efficacy of selected strategies for obtaining high quality data annotations from non-experts. Our results point to the advantages of person-oriented strategies over process-oriented strategies. Specifically, we find that screening workers for requisite cognitive aptitudes and providing training in qualitative coding techniques is quite effective, significantly outperforming control and baseline conditions. Interestingly, such strategies can improve coder annotation accuracy above and beyond common benchmark strategies such as Bayesian Truth Serum (BTS).
更多查看译文
关键词
Human Computation,Crowd Sourcing,Mechanical Turk,Experimentation,Qualitative Coding,Micro Task
AI 理解论文
溯源树
样例
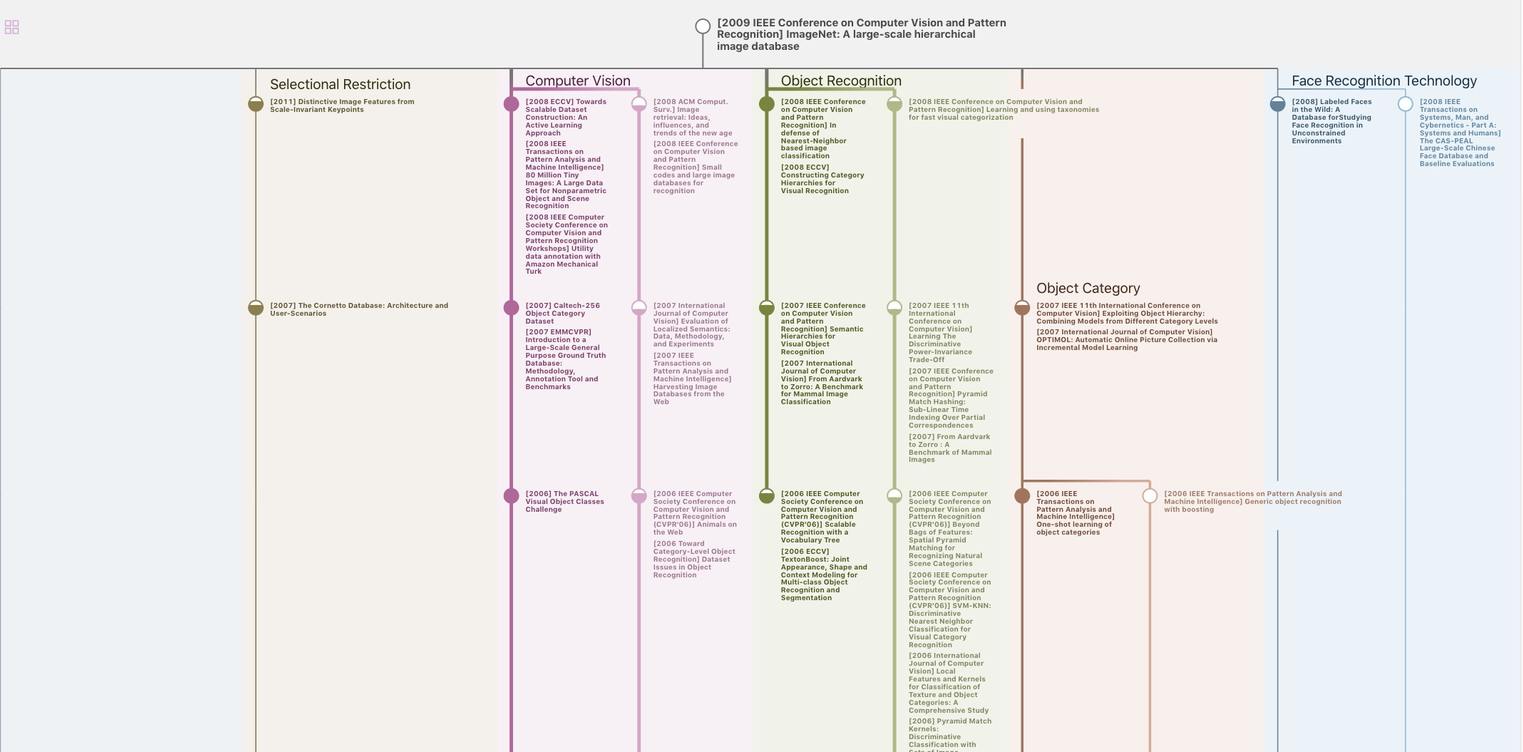
生成溯源树,研究论文发展脉络
Chat Paper
正在生成论文摘要