Transforming collaborative filtering into supervised learning.
Expert Syst. Appl.(2015)
摘要
We propose an transformation from CF problem to typical supervised learning.The proposed transformation is straightforward and domain-independent.Our transformation greatly outperforms classical collaborative filtering techniques. Collaborative Filtering (CF) is a well-known approach for Recommender Systems (RS). This approach extrapolates rating predictions from ratings given by user on items, which are represented by a user-item matrix filled with a rating r i , j given by an user i on an item j. Therefore, CF has been confined to this data structure relying mostly on adaptations of supervised learning methods to deal with rating predictions and matrix decomposition schemes to complete unfilled positions of the rating matrix. Although there have been proposals to apply Machine Learning (ML) to RS, these works had to transform the rating matrix into the typical Supervised Learning (SL) data set, i.e., a set of pairwise tuples ( x , y ) , where y is the correspondent class (the rating) of the instance x ¿ R k . So far, the proposed transformations were thoroughly crafted using the domain information. However, in many applications this kind of information can be incomplete, uncertain or stated in ways that are not machine-readable. Even when it is available, its usage can be very complex requiring specialists to craft the transformation. In this context, this work proposes a domain-independent transformation from the rating matrix representation to a supervised learning dataset that enables SL methods to be fully explored in RS. In addition, our transformation is said to be straightforward, in the sense that, it is an automatic process that any lay person can perform requiring no domain specialist. Our experiments have proven that our transformation, combined with SL methods, have greatly outperformed classical CF methods.
更多查看译文
关键词
recommender system,collaborative filtering,supervised learning,dimensionality reduction
AI 理解论文
溯源树
样例
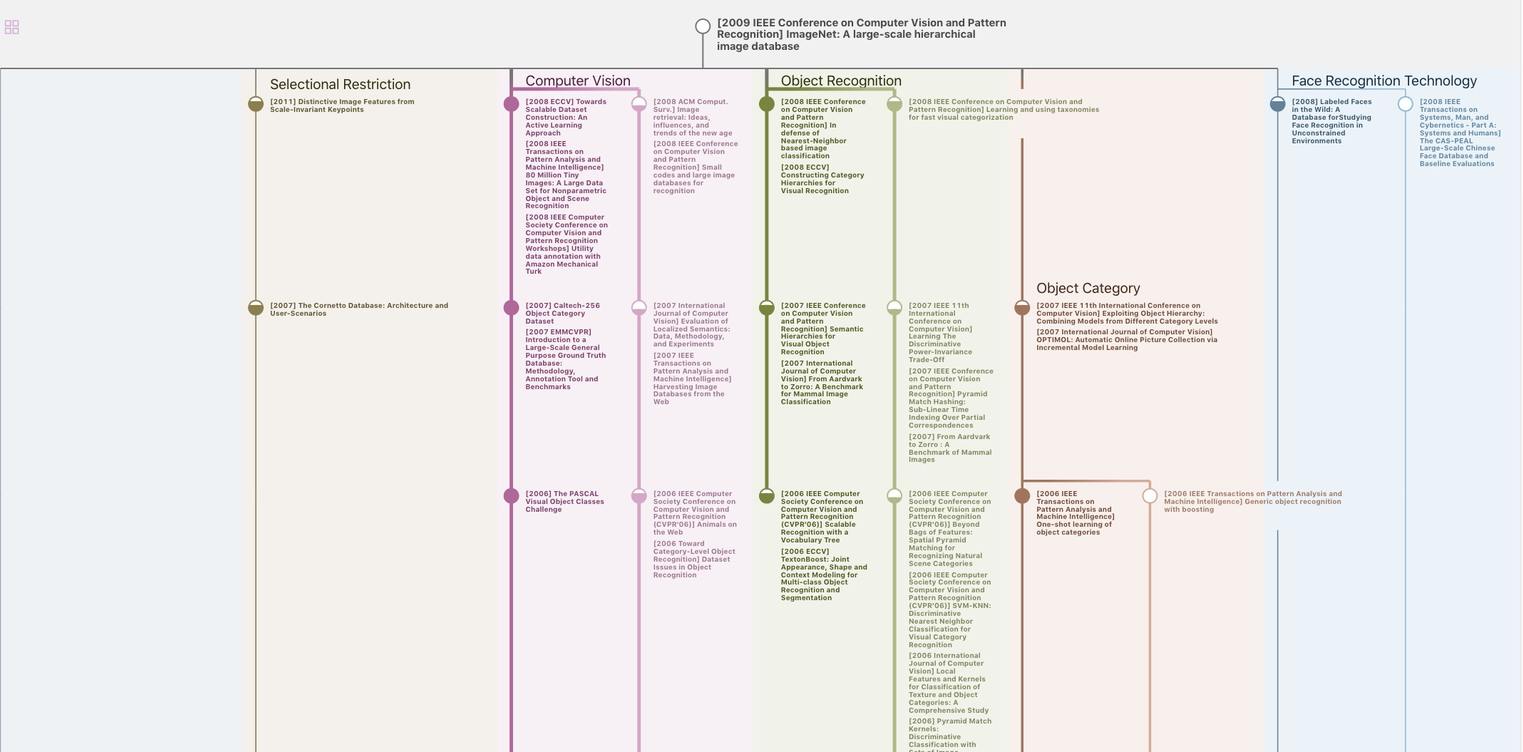
生成溯源树,研究论文发展脉络
Chat Paper
正在生成论文摘要