Joint video frame set division and low-rank decomposition for background subtraction
IEEE Transactions on Circuits and Systems for Video Technology(2014)
摘要
The recently proposed robust principle component analysis (RPCA) has been successfully applied in background subtraction. However, low-rank decomposition makes sense on the condition that the foreground pixels (sparsity patterns) are uniformly located at the scene, which is not realistic in real-world applications. To overcome this limitation, we reconstruct the input video frames and aim to make the foreground pixels not only sparse in space but also sparse in time. Therefore, we propose a joint video frame set division and RPCA-based method for background subtraction. In addition, we use the motion as a priori knowledge which has not been considered in the current subspace-based methods. The proposed method consists of two phases. In the first phase, we propose a lower bound-based within-class maximum division method to divide the video frame set into several subsets. In this way, the successive frames are assigned to different subsets in which the foregrounds are located at the scene randomly. In the second phase, we augment each subset using the frames with a small quantity of motion. To evaluate the proposed method, the experiments are conducted on real-world and public datasets. The comparisons with the state-of-the-art background subtraction methods validate the superiority of our method.
更多查看译文
关键词
Background subtraction, low-rank decomposition, motion priori knowledge, within-class maximum division
AI 理解论文
溯源树
样例
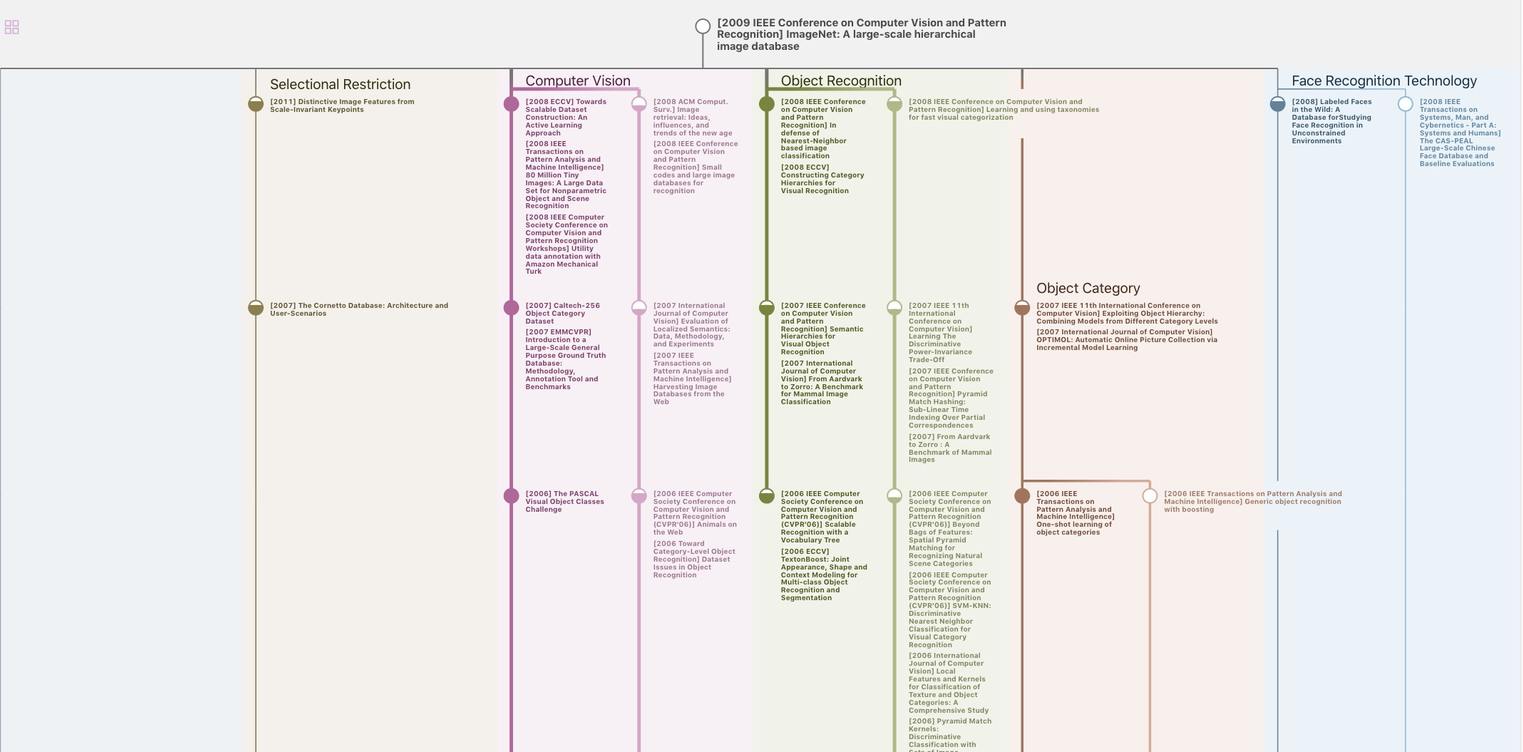
生成溯源树,研究论文发展脉络
Chat Paper
正在生成论文摘要