Compact color-texture description for texture classification
Pattern Recognition Letters(2015)
摘要
We show that combining multiple texture description methods significantly improves the performance compared to using the single best texture method alone.We further propose to use information theoretic compression approach to compress high-dimensional multi-texture features into a compact heterogeneous texture representation.We perform a comprehensive evaluation of color features, popular in object recognition, for the task of texture classification.We show that late fusion of our compact texture descriptor with discriminative color feature outperforms state-of-the-art results on challenging texture recognition datasets. Describing textures is a challenging problem in computer vision and pattern recognition. The classification problem involves assigning a category label to the texture class it belongs to. Several factors such as variations in scale, illumination and viewpoint make the problem of texture description extremely challenging. A variety of histogram based texture representations exists in literature. However, combining multiple texture descriptors and assessing their complementarity is still an open research problem. In this paper, we first show that combining multiple local texture descriptors significantly improves the recognition performance compared to using a single best method alone. This gain in performance is achieved at the cost of high-dimensional final image representation. To counter this problem, we propose to use an information-theoretic compression technique to obtain a compact texture description without any significant loss in accuracy. In addition, we perform a comprehensive evaluation of pure color descriptors, popular in object recognition, for the problem of texture classification. Experiments are performed on four challenging texture datasets namely, KTH-TIPS-2a, KTH-TIPS-2b, FMD and Texture-10. The experiments clearly demonstrate that our proposed compact multi-texture approach outperforms the single best texture method alone. In all cases, discriminative color names outperforms other color features for texture classification. Finally, we show that combining discriminative color names with compact texture representation outperforms state-of-the-art methods by 7.8%, 4.3% and 5.0% on KTH-TIPS-2a, KTH-TIPS-2b and Texture-10 datasets respectively.
更多查看译文
关键词
image classification
AI 理解论文
溯源树
样例
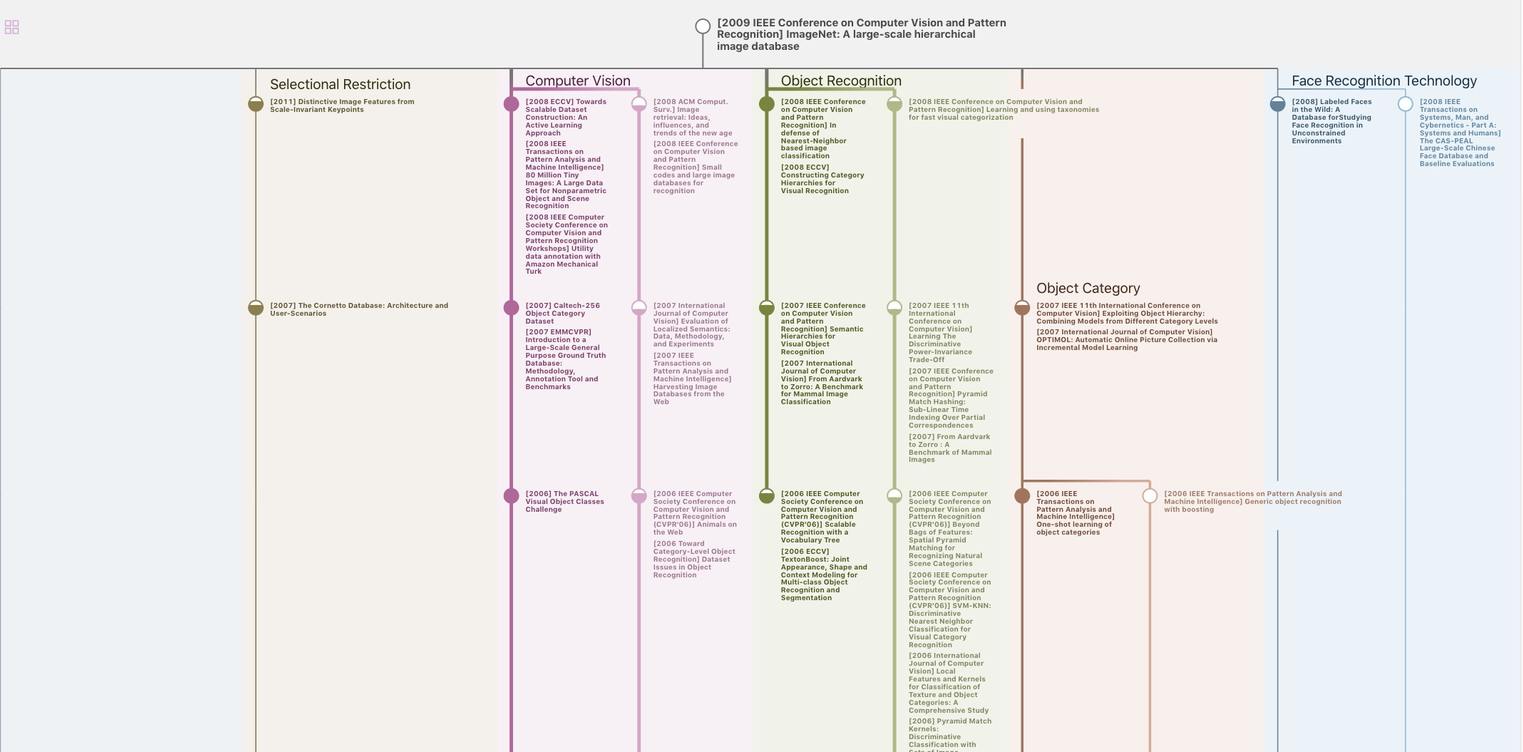
生成溯源树,研究论文发展脉络
Chat Paper
正在生成论文摘要