Randomly Spiking Dynamic Neural Fields
JETC(2015)
摘要
Bio-inspired neural computation attracts a lot of attention as a possible solution for the future challenges in designing computational resources. Dynamic neural fields (DNF) provide cortically inspired models of neural populations to which computation can be applied for a wide variety of tasks, such as perception and sensorimotor control. DNFs are often derived from continuous neural field theory (CNFT). In spite of the parallel structure and regularity of CNFT models, few studies of hardware implementations have been carried out targeting embedded real-time processing. In this article, a hardware-friendly model adapted from the CNFT is introduced, namely the RSDNF model (randomly spiking dynamic neural fields). Thanks to their simplified 2D structure, RSDNFs achieve scalable parallel implementations on digital hardware while maintaining the behavioral properties of CNFT models. Spike-based computations within neurons in the field are introduced to reduce interneuron connection bandwidth. Additionally, local stochastic spike propagation ensures inhibition and excitation broadcast without a fully connected network. The behavioral soundness and robustness of the model in the presence of noise and distracters is fully validated through software and hardware. A field programmable gate array (FPGA) implementation shows how the RSDNF model ensures a level of density and scalability out of reach for previous hardware implementations of dynamic neural field models.
更多查看译文
关键词
design,fpga,cellular automata,neuromorphic engineering,reliability,vision and scene understanding,spiking neurons,dynamic neural fields
AI 理解论文
溯源树
样例
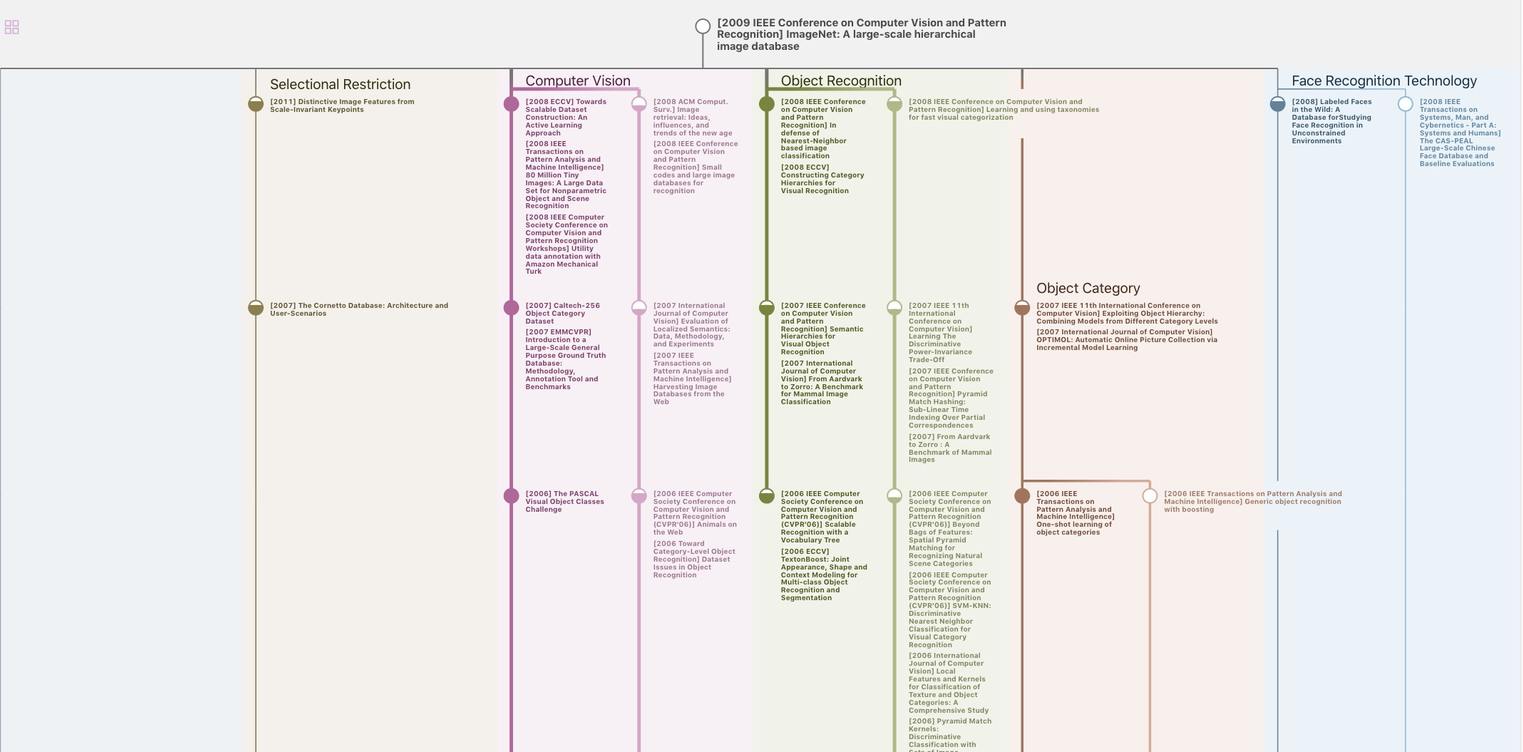
生成溯源树,研究论文发展脉络
Chat Paper
正在生成论文摘要