Spectral Clustering With Neighborhood Attribute Reduction Based On Information Entropy
Journal of Computers(2014)
摘要
Traditional rough set theory is only suitable for dealing with discrete variables and need data preprocessing. Neighborhood rough sets overcome these shortcomings with the ability to directly process numeric data. This paper modifies the attribute reduction method based on neighborhood rough sets, in which the attribute importance is combined with information entropy to select the appropriate attributes. When multiple attributes have the same importance degree, compare the information entropy of these attributes. Put the attribute having the minimal entropy into the reduction set, so that the reduced attribute set is better. Then we introduce this attribute reduction method to improve spectral clustering and propose NRSRSC algorithm. It can highlight the differences between samples while maintaining the characteristics of data points to make the final clustering results closer to the real data classes. Experiments show that, NRSR-SC algorithm is superior to traditional spectral clustering algorithm and FCM algorithm. Its clustering accuracy is higher, and has strong robustness to the noise in high-dimensional data.
更多查看译文
关键词
neighborhood rough sets,information entropy,attribute reduction,spectral clustering
AI 理解论文
溯源树
样例
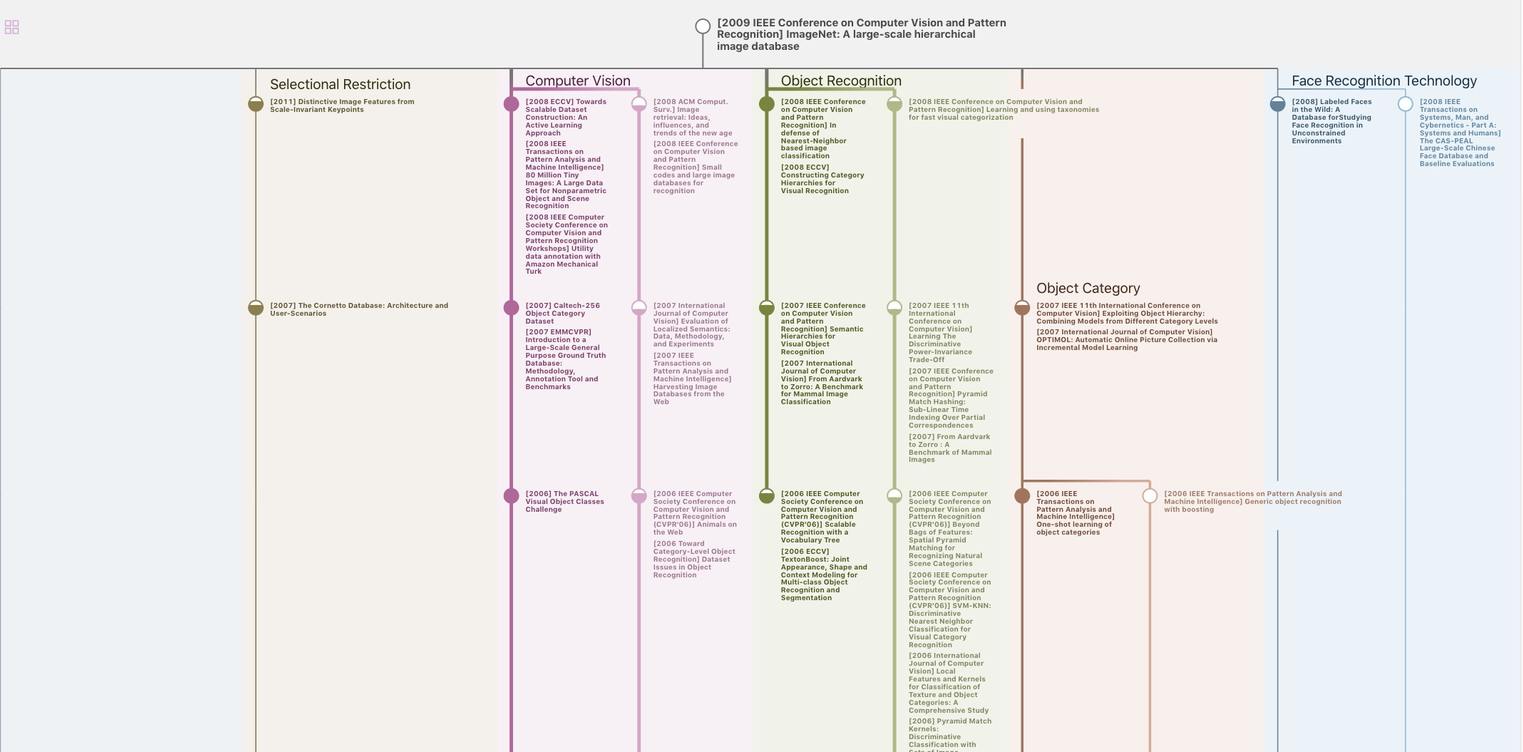
生成溯源树,研究论文发展脉络
Chat Paper
正在生成论文摘要