Fast adaptation of deep neural network based on discriminant codes for speech recognition
IEEE/ACM Transactions on Audio, Speech & Language Processing(2014)
摘要
Fast adaptation of deep neural networks (DNN) is an important research topic in deep learning. In this paper, we have proposed a general adaptation scheme for DNN based on discriminant condition codes, which are directly fed to various layers of a pre-trained DNN through a new set of connection weights. Moreover, we present several training methods to learn connection weights from training data as well as the corresponding adaptation methods to learn new condition code from adaptation data for each new test condition. In this work, the fast adaptation scheme is applied to supervised speaker adaptation in speech recognition based on either frame-level cross-entropy or sequence-level maximum mutual information training criterion. We have proposed three different ways to apply this adaptation scheme based on the so-called speaker codes: i) Nonlinear feature normalization in feature space; ii) Direct model adaptation of DNN based on speaker codes; iii) Joint speaker adaptive training with speaker codes. We have evaluated the proposed adaptation methods in two standard speech recognition tasks, namely TIMIT phone recognition and large vocabulary speech recognition in the Switchboard task. Experimental results have shown that all three methods are quite effective to adapt large DNN models using only a small amount of adaptation data. For example, the Switchboard results have shown that the proposed speaker-code-based adaptation methods may achieve up to 8-10% relative error reduction using only a few dozens of adaptation utterances per speaker. Finally, we have achieved very good performance in Switchboard (12.1% in WER) after speaker adaptation using sequence training criterion, which is very close to the best performance reported in this task (\"Deep convolutional neural networks for LVCSR,\" T. N. Sainath et al., Proc. IEEE Acoust., Speech, Signal Process., 2013).
更多查看译文
关键词
speaker code,maximum mutual information,deep neural network,speech recognition and synthesis,design,sequence training criterion,experimentation,standard speech recognition tasks,general adaptation scheme,test condition,frame-level cross-entropy,cross entropy (ce),pre-trained dnn,learning (artificial intelligence),direct model adaptation,maximum mutual information (mmi),large vocabulary speech recognition,deep neural network (dnn),connection weight learning,speaker-code-based adaptation methods,fast adaptation,switchboard task,discriminant codes,speaker recognition,self-modifying machines,joint speaker adaptive training,measurement,languages,deep learning,signal analysis, synthesis, and processing,supervised speaker adaptation,speech coding,adaptation data,feature space,sequence-level maximum mutual information training criterion,entropy,cross entropy,nonlinear feature normalization,performance,neural nets,condition code,timit phone recognition,training data,error reduction,deep neural network fast adaptation
AI 理解论文
溯源树
样例
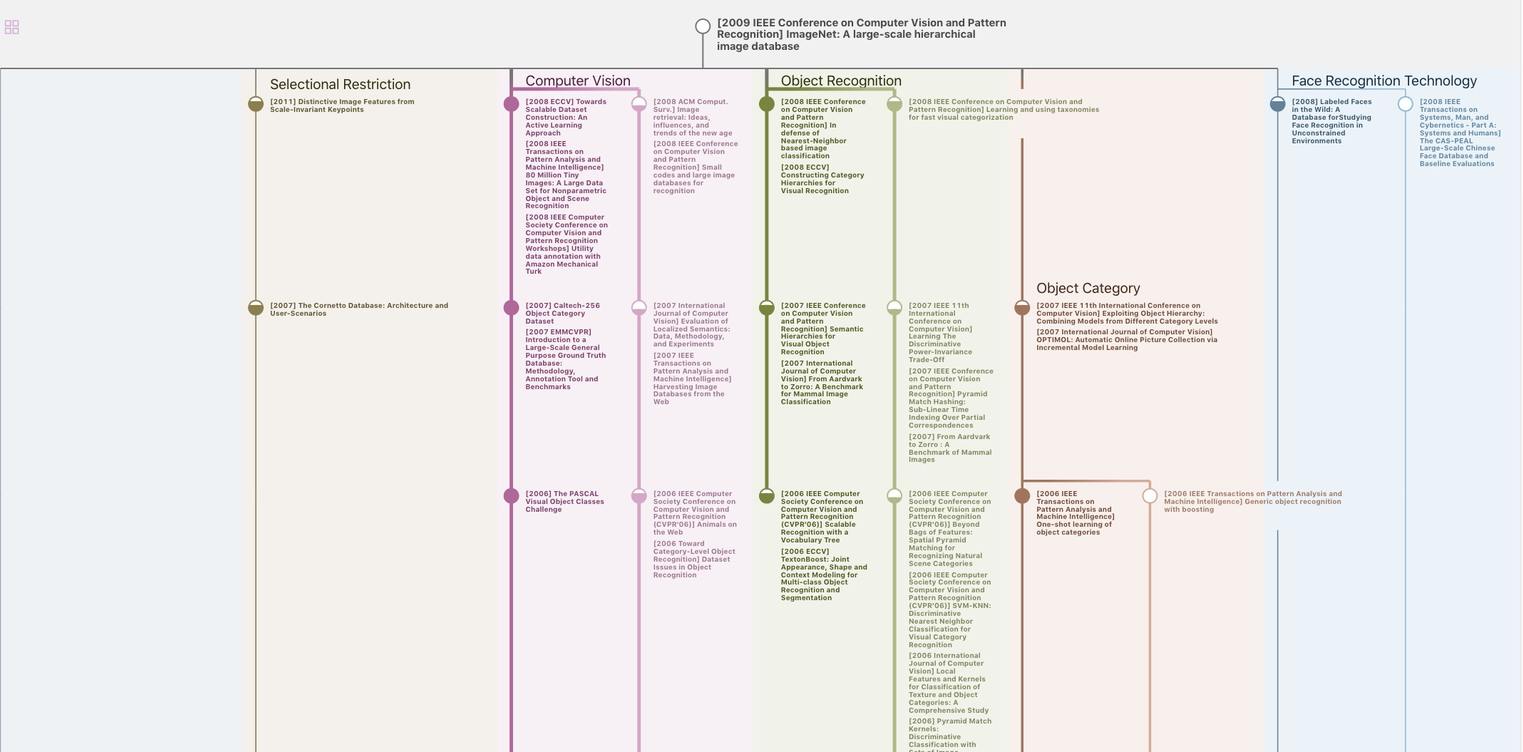
生成溯源树,研究论文发展脉络
Chat Paper
正在生成论文摘要