A Unifying Framework for Typical Multitask Multiple Kernel Learning Problems
IEEE Trans. Neural Netw. Learning Syst.(2014)
摘要
Over the past few years, multiple kernel learning (MKL) has received significant attention among data-driven feature selection techniques in the context of kernel-based learning. MKL formulations have been devised and solved for a broad spectrum of machine learning problems, including multitask learning (MTL). Solving different MKL formulations usually involves designing algorithms that are tailored to the problem at hand, which is, typically, a nontrivial accomplishment. In this paper we present a general multitask multiple kernel learning (MT-MKL) framework that subsumes well-known MT-MKL formulations, as well as several important MKL approaches on single-task problems. We then derive a simple algorithm that can solve the unifying framework. To demonstrate the flexibility of the proposed framework, we formulate a new learning problem, namely partially-shared common space MT-MKL, and demonstrate its merits through experimentation.
更多查看译文
关键词
machine learning,supervised learning,optimization methods,support vector machines (svms),multitask multiple kernel learning problems,pattern recognition,multitask learning,multitask multiple kernel learning framework,learning (artificial intelligence),partially-shared common space mt-mkl,data-driven feature selection techniques,kernel-based learning,single-task problems,feature selection,mt-mkl framework,mtl,support vector machines (svms).,learning artificial intelligence,algorithm design and analysis,kernel,support vector machines,optimization,vectors
AI 理解论文
溯源树
样例
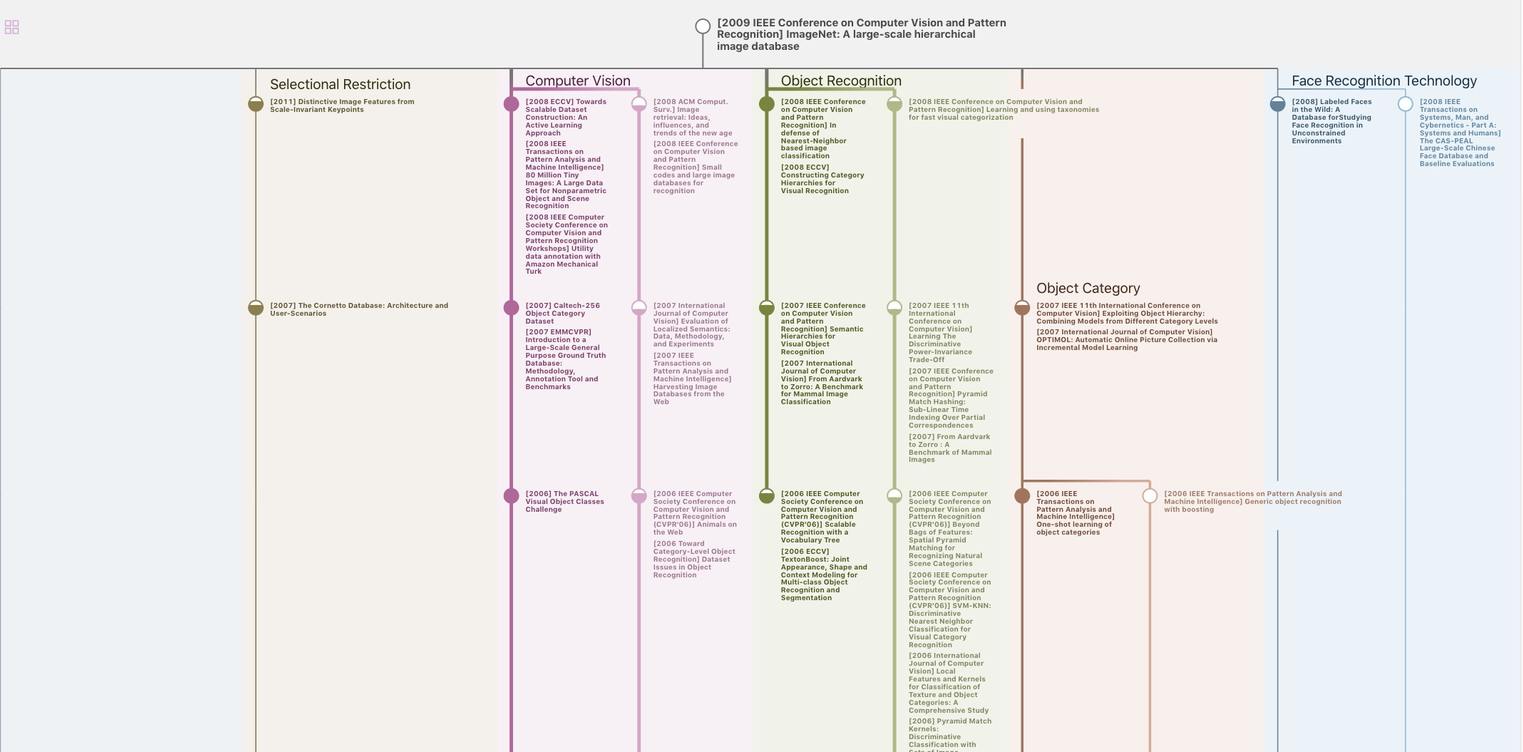
生成溯源树,研究论文发展脉络
Chat Paper
正在生成论文摘要