Task-Based Robot Grasp Planning Using Probabilistic Inference
Robotics, IEEE Transactions (2015)
摘要
Grasping and manipulating everyday objects in a goal-directed manner is an important ability of a service robot. The robot needs to reason about task requirements and ground these in the sensorimotor information. Grasping and interaction with objects are challenging in real-world scenarios, where sensorimotor uncertainty is prevalent. This paper presents a probabilistic framework for the representation and modeling of robot-grasping tasks. The framework consists of Gaussian mixture models for generic data discretization, and discrete Bayesian networks for encoding the probabilistic relations among various task-relevant variables, including object and action features as well as task constraints. We evaluate the framework using a grasp database generated in a simulated environment including a human and two robot hand models. The generative modeling approach allows the prediction of grasping tasks given uncertain sensory data, as well as object and grasp selection in a task-oriented manner. Furthermore, the graphical model framework provides insights into dependencies between variables and features relevant for object grasping.
更多查看译文
关键词
Robot sensing systems,Grasping,Probabilistic logic,Data models,Planning,Training
AI 理解论文
溯源树
样例
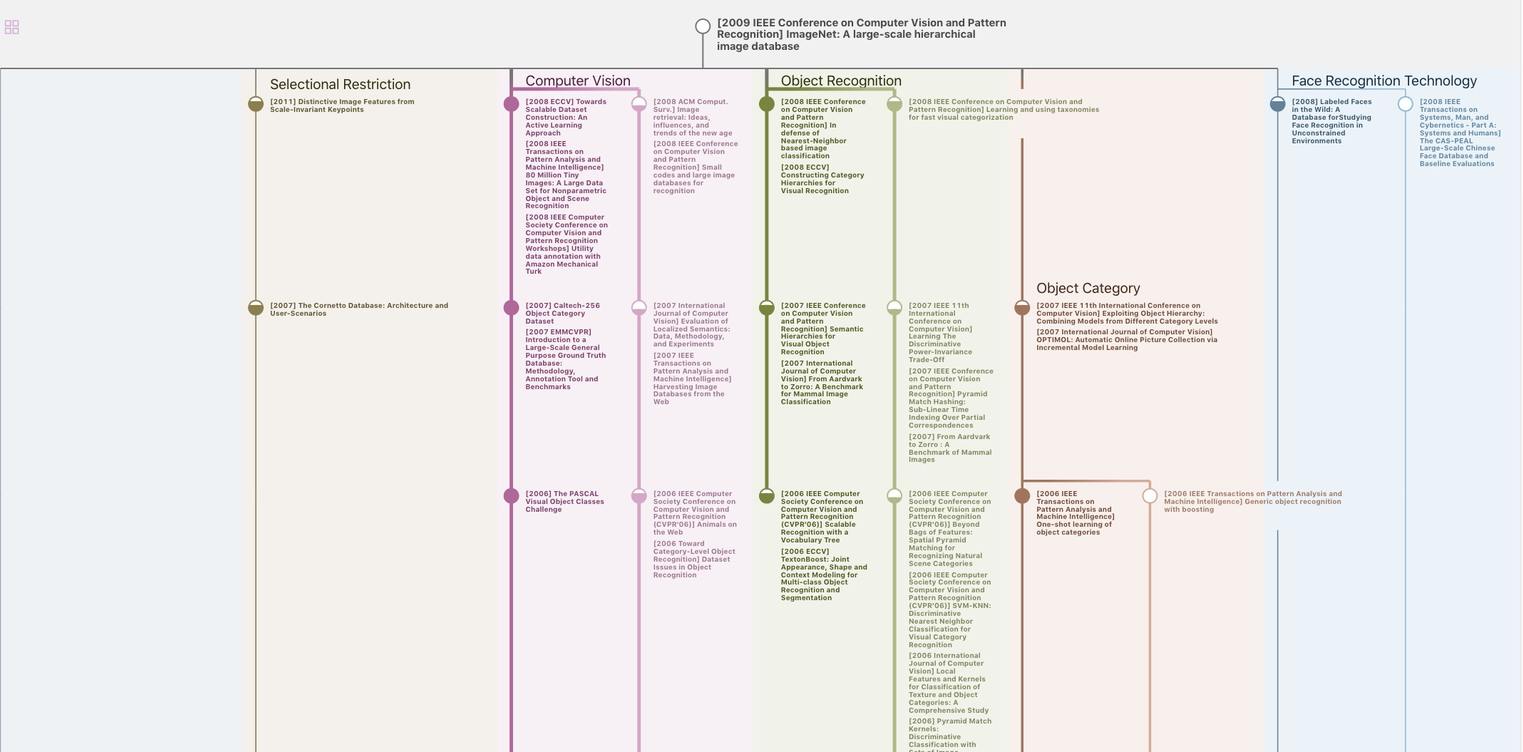
生成溯源树,研究论文发展脉络
Chat Paper
正在生成论文摘要