Prediction of Speech Intelligibility Using a Neurogram Orthogonal Polynomial Measure (NOPM)
IEEE/ACM Transactions on Audio, Speech & Language Processing(2015)
摘要
Sensorineural hearing loss (SNHL) is an increasingly prevalent condition, resulting from damage to the inner ear and causing a reduction in speech intelligibility. This paper proposes a new speech intelligibility prediction metric, the neurogram orthogonal polynomial measure (NOPM). This metric applies orthogonal moments to the auditory neurogram to predict speech intelligibility for listeners with and without hearing loss. The model simulates the responses of auditory-nerve fibers to speech signals under quiet and noisy conditions. Neurograms were created using a physiologically based computational model of the auditory periphery. A well-known orthogonal polynomial measure, Krawtchouk moments, was applied to extract features from the auditory neurogram. The predicted intelligibility scores were compared to subjective results, and NOPM showed a good fit with the subjective scores for normal listeners and also for listeners with hearing loss. The proposed metric has a realistic and wider dynamic range than corresponding existing metrics, such as mean structural similarity index measure and neurogram similarity index measure, and the predicted scores are also well-separated as a function of hearing loss. The application of this metric could be extended for assessing hearing-aid and speech-enhancement algorithms.
更多查看译文
关键词
biomedical measurement,ear,feature extraction,hearing,medical computing,medical signal processing,method of moments,neurophysiology,polynomial approximation,speech enhancement,speech intelligibility,krawtchouk moments,nopm metric,neurogram orthogonal polynomial measure,snhl prevalence,auditory neurogram feature extraction,auditory periphery computational model,auditory-nerve fiber response simulation,hearing loss function,hearing-aid algorithm assessment,inner ear damage,listener hearing loss,mean structural similarity index measure,neurogram similarity index measure,noisy condition speech signals,normal listener subjective score,orthogonal moments,physiologically based computational model,predicted intelligibility scores,quiet condition speech signals,realistic dynamic range,sensorineural hearing loss,speech intelligibility prediction metric,speech intelligibility reduction,speech-enhancement algorithm assessment,well-known orthogonal polynomial measure,well-separated predicted scores,wider dynamic range,auditory-nerve model,neurogram,orthogonal moment,measurement,polynomials,speech,computational modeling,speech recognition
AI 理解论文
溯源树
样例
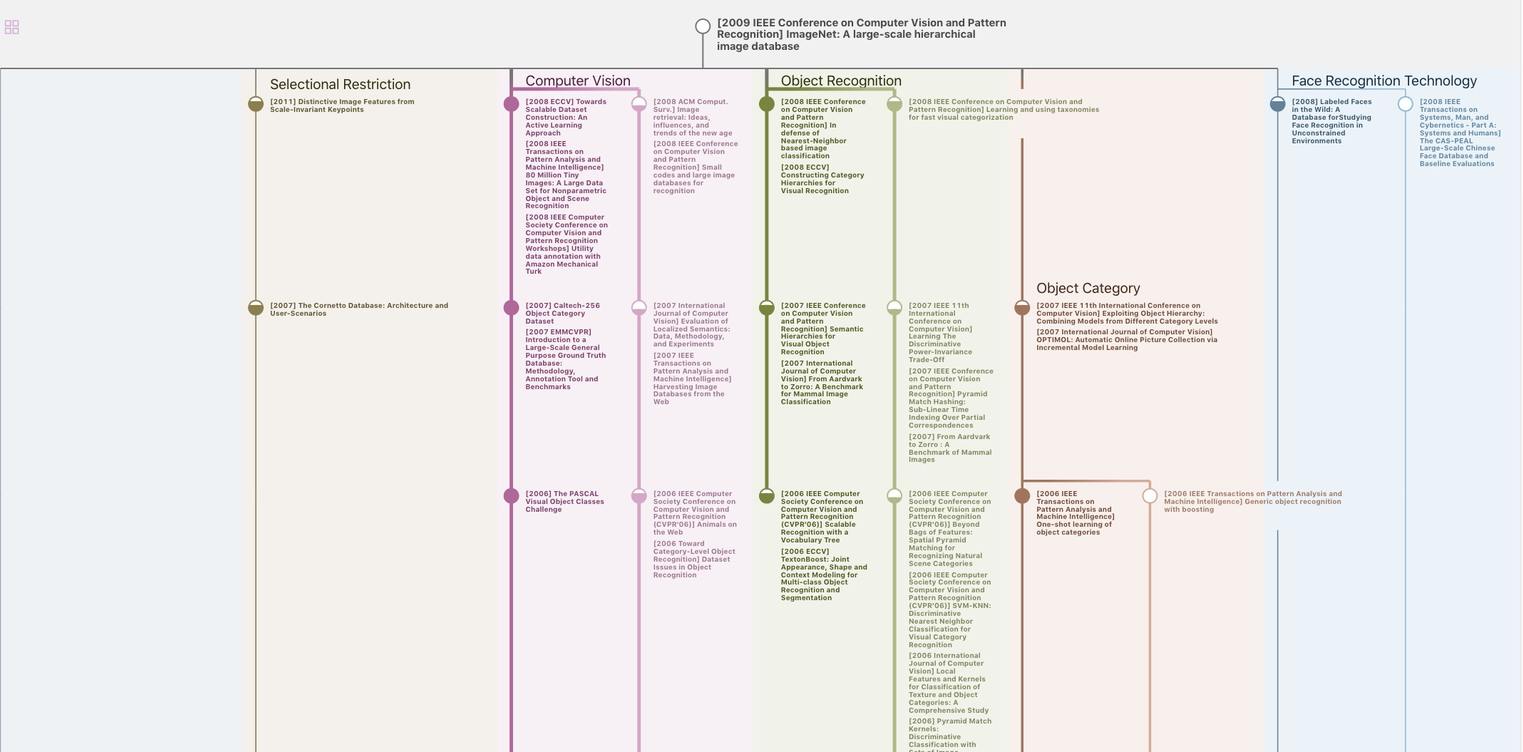
生成溯源树,研究论文发展脉络
Chat Paper
正在生成论文摘要