Robust Beamforming by an Improved Neural Minor Component Analysis Algorithm
Computational Science and Engineering(2014)
摘要
Recently, neural minor component analysis (MCA) has attracted much attention. It has features of high calculation speed and strong fault tolerant property. In this paper, neural MCA network is applied in adaptive beam forming. In order to get over the disadvantages that MCA based beam former cannot form null steering beams at the direction of interference and avoid stagnation behavior of algorithm convergence, a novel algorithm combining the least mean square (LMS) algorithm with MCA algorithm is provided, which uses LMS learning rule to continue the training of the neural MCA network. Simulations show that the MCA-LMS based beam former has strong stability, resembled convergence rates and can obtain a 0dB main-lobe at the direction of primary signal while forming a more than 40dB null steering at the direction of interference.
更多查看译文
关键词
least mean square,neural network,strong fault tolerant property,neural mca network,mca-lms based beamformer,primary signal direction,interference direction,stagnation behavior avoidance,learning (artificial intelligence),null steering beams,lms learning rule,fault tolerance,array signal processing,least mean squares methods,convergence rates,robust adaptive beamforming,high calculation speed,minor component analysis,beam steering,least mean square algorithm,neural nets,interference (signal),neural minor component analysis algorithm improvement,adaptive beamforming,robustness,interference,vectors
AI 理解论文
溯源树
样例
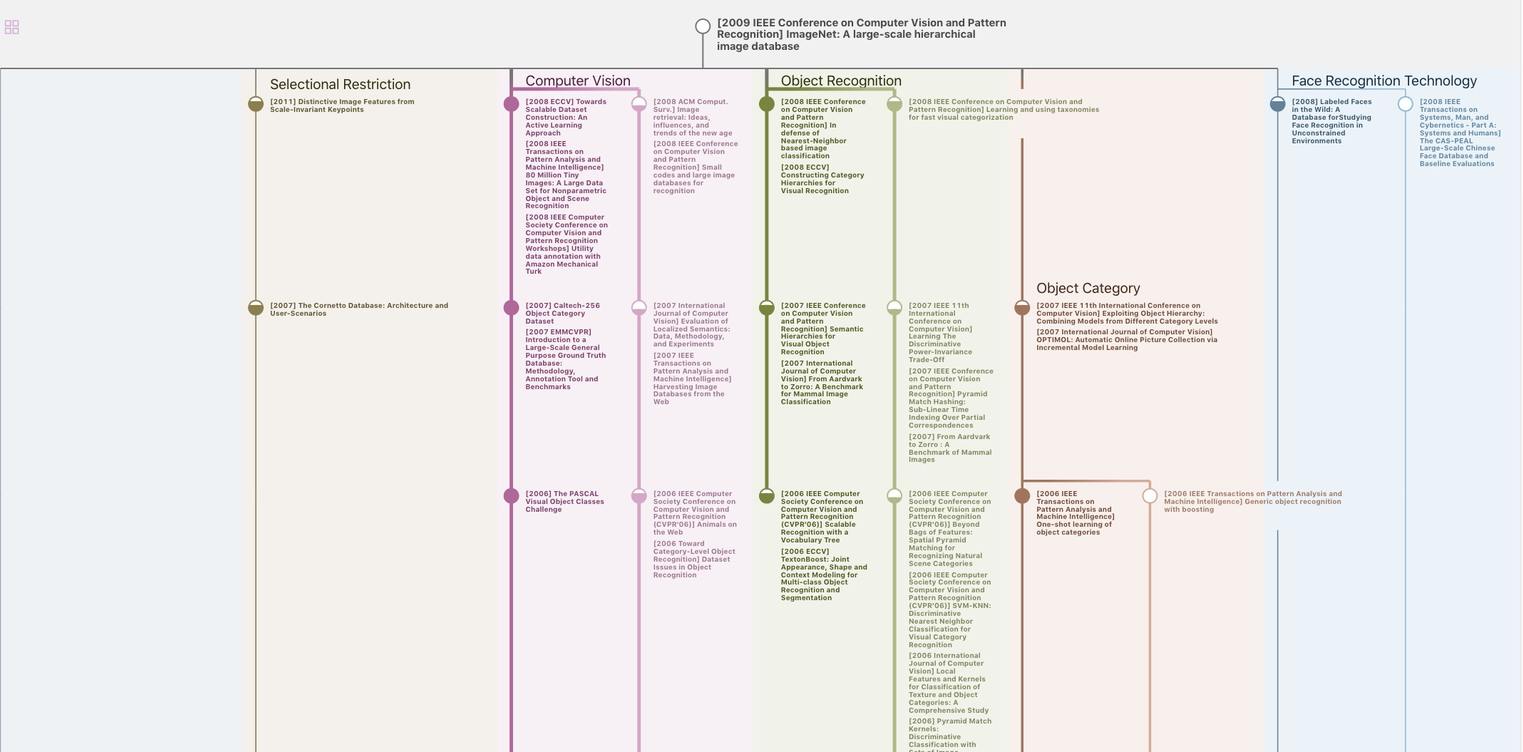
生成溯源树,研究论文发展脉络
Chat Paper
正在生成论文摘要