Technology-design co-optimization of resistive cross-point array for accelerating learning algorithms on chip
DATE(2015)
摘要
Technology-design co-optimization methodologies of the resistive cross-point array are proposed for implementing the machine learning algorithms on a chip. A novel read and write scheme is designed to accelerate the training process, which realizes fully parallel operations of the weighted sum and the weight update. Furthermore, technology and design parameters of the resistive cross-point array are co-optimized to enhance the learning accuracy, latency and energy consumption, etc. In contrast to the conventional memory design, a set of reverse scaling rules is proposed on the resistive cross-point array to achieve high learning accuracy. These include 1) larger wire width to reduce the IR drop on interconnects thereby increasing the learning accuracy; 2) use of multiple cells for each weight element to alleviate the impact of the device variations, at an affordable expense of area, energy and latency. The optimized resistive cross-point array with peripheral circuitry is implemented at the 65 nm node. Its performance is benchmarked for handwritten digit recognition on the MNIST database using gradient-based sparse coding. Compared to state-of-the-art software approach running on CPU, it achieves >103 speed-up and >106 energy efficiency improvement, enabling real-time image feature extraction and learning.
更多查看译文
关键词
machine learning,resistance,encoding,latency,feature extraction,accuracy,mnist database,neuromorphic computing,resistive memory,learning artificial intelligence
AI 理解论文
溯源树
样例
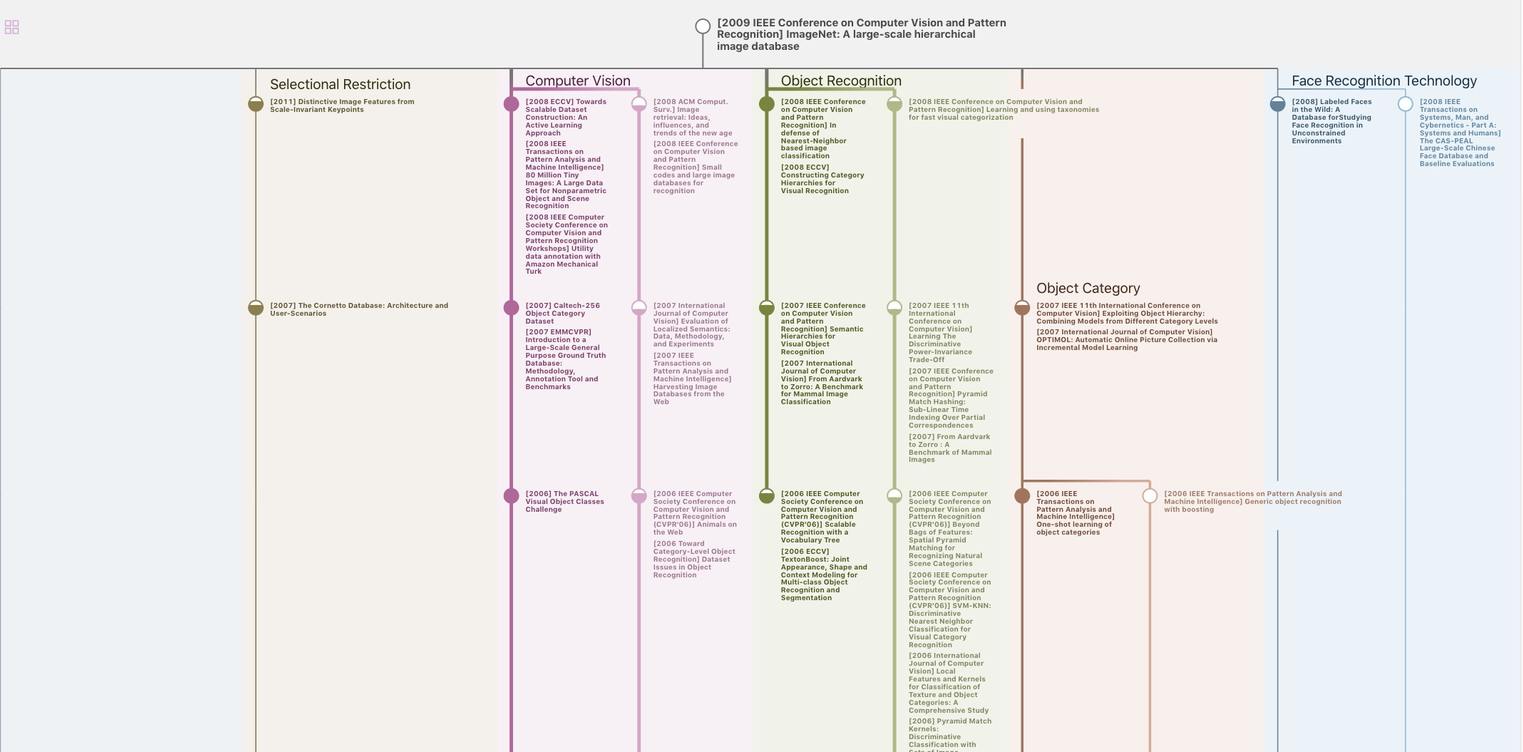
生成溯源树,研究论文发展脉络
Chat Paper
正在生成论文摘要