Three-dimensional Gabor feature extraction for hyperspectral imagery classification using a memetic framework
Information Sciences(2015)
摘要
Feature extraction based on three-dimensional (3D) wavelet transform is capable of improving the classification accuracy of hyperspectral imagery data by simultaneously capturing the geometrical and statistical spectral–spatial structure of the data. Nevertheless, the design of wavelets is always proceeded with empirical parameters, which tends to involve a large number of irrelevant and redundant spectral–spatial features and results in suboptimal configuration. This paper proposes a 3D Gabor wavelet feature extraction in a memetic framework, named M3DGFE, for hyperspectral imagery classification. Particularly, the parameter setting of 3D Gabor wavelet feature extraction is optimized using memetic algorithm so that discriminative and parsimonious feature set is acquired for accurate classification. M3DGFE is characterized by an efficient fitness evaluation function and a pruning local search. In the fitness evaluation function, a new concept of redundancy-free relevance based on conditional mutual information is proposed to measure the goodness of the extracted candidate features. The pruning local search is specially designed to eliminate both irrelevant and redundant features without sacrificing the discriminability of the obtained feature subset. M3DGFE is tested on both pixel-level and image-level classification using real-world hyperspectral remote sensing data and hyperspectral face data, respectively. The experimental results show that M3DGFE achieves promising classification accuracy with parsimonious feature subset.
更多查看译文
关键词
Memetic algorithm,Gabor wavelet transform,Feature extraction,Feature selection,Hyperspectral imagery classification
AI 理解论文
溯源树
样例
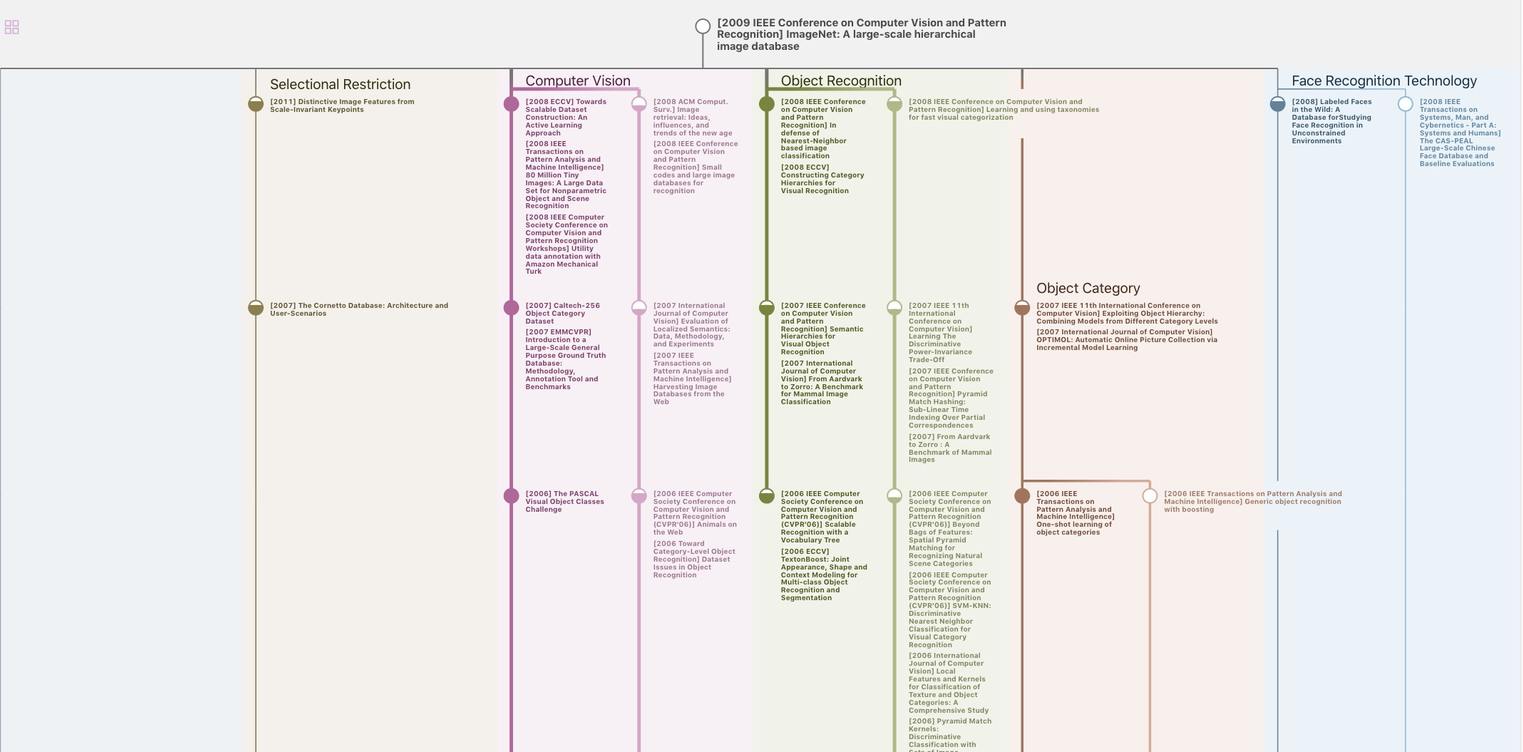
生成溯源树,研究论文发展脉络
Chat Paper
正在生成论文摘要