An extended supervised term weighting method for text categorization
Lecture Notes in Electrical Engineering(2011)
摘要
When Support Vector Machines (SVM) are exploited for automatic text categorization, text representation and term weighting have a significant impact on the performance of text classification. Conventional supervised weighting methods only focus on the frequency characteristics of feature terms, without consideration of semantic characteristics of them. Inspired by supervised weighting method, semantic distance between terms and categories is introduced into term weights calculation. The first step is modeling each category with two vectors of feature terms, which are called category core terms, and acquiring these terms by machine learning methods. Second, the semantic distance between feature terms and category core terms is calculated based on semantic database. Third, the global weight factor is replaced by the sematic distance to calculate the weight of every term. Based on the standard benchmark Reuters-21578, this kind of term weighting schemas can generally produce satisfied results of classification using SVMlight as classifier with default parameters. © 2011 Springer Science+Business Media B.V.
更多查看译文
关键词
semantic distance,support vector machines,term weighting,text categorization
AI 理解论文
溯源树
样例
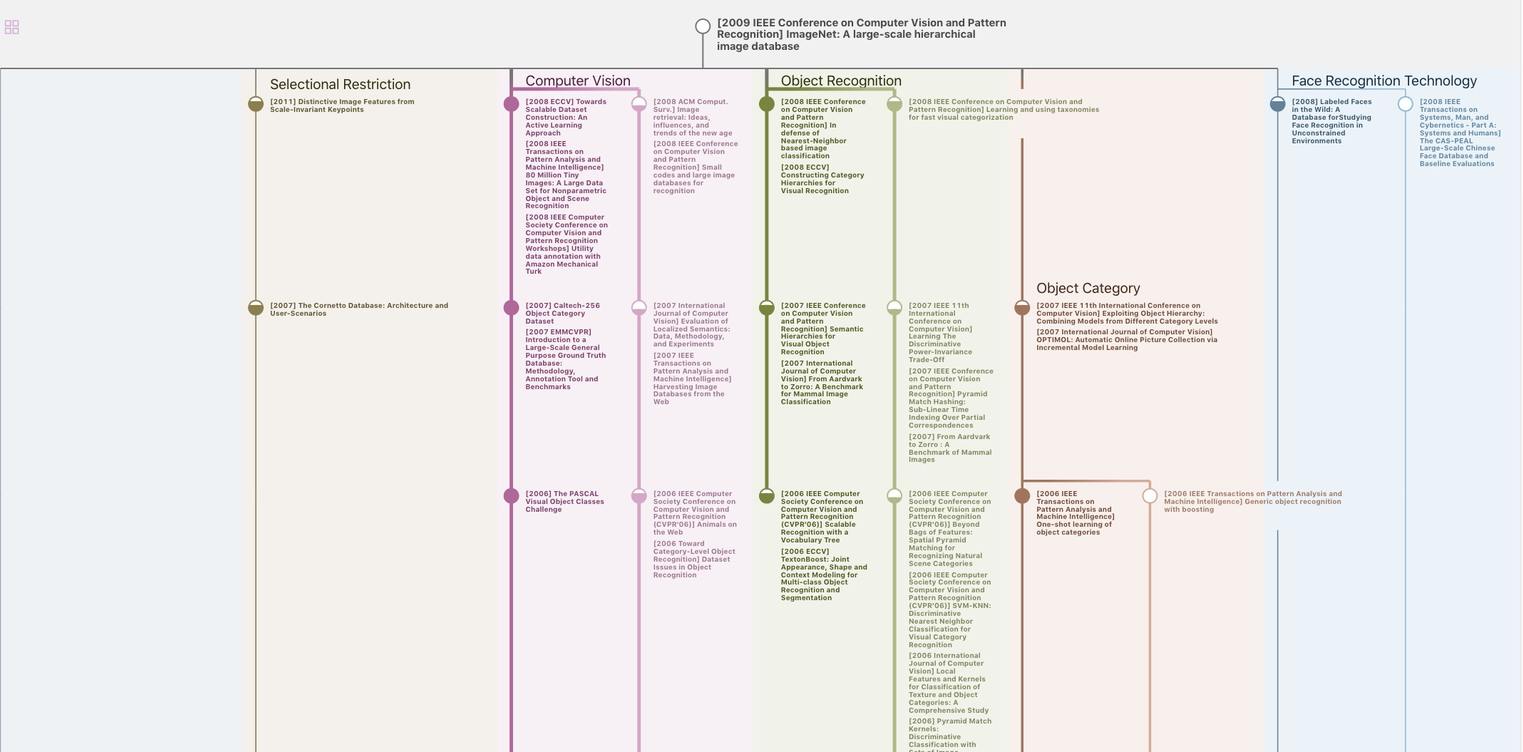
生成溯源树,研究论文发展脉络
Chat Paper
正在生成论文摘要