Soft-sensing model for flue gas oxygen content based on kernel fuzzy C-means clustering and local modeling method
Control Conference(2011)
摘要
Based on the fact that the flue gas oxygen content in power plant is hard to detect effectively, a soft-sensing model based on kernel fuzzy C-means clustering and local modeling method is proposed from improving the online self-adaptive ability of the soft-sensing model. Firstly, several sub-sample sets are formed by using kernel fuzzy C-means clustering algorithm to cluster analysis of the history database. Secondly, the modeling neighborhood dataset is obtained through traversal search in the sub-sample set, whose clustering center has the highest similarity with the current input data. Thirdly, the least square support vector machine based on multi-population hybrid optimization algorithm is used to build the local model for flue gas oxygen content. Finally, the simulation experiments are carried out based on the actual operation data. Simulation results show that compared with the standard LSSVM soft-sensing model, although the computing cost is increased, the proposed soft-sensing model has better prediction performance and can satisfy the real-time requirements for flue gas oxygen content in boiler combustion process.
更多查看译文
关键词
optimisation,kernel fuzzy c-means clustering,fuzzy set theory,pattern clustering,multi-population hybrid optimization algorithm,local modeling method,multipopulation hybrid optimization algorithm,online adaptive,least square support vector machine,soft-sensing model,traversal search,neighborhood dataset modeling,least squares approximations,oxygen,flue gas oxygen content,flue gases,gas sensors,power plant,support vector machines,power plants,online self-adaptive ability,clustering algorithms,data models,predictive models,computational modeling,kernel
AI 理解论文
溯源树
样例
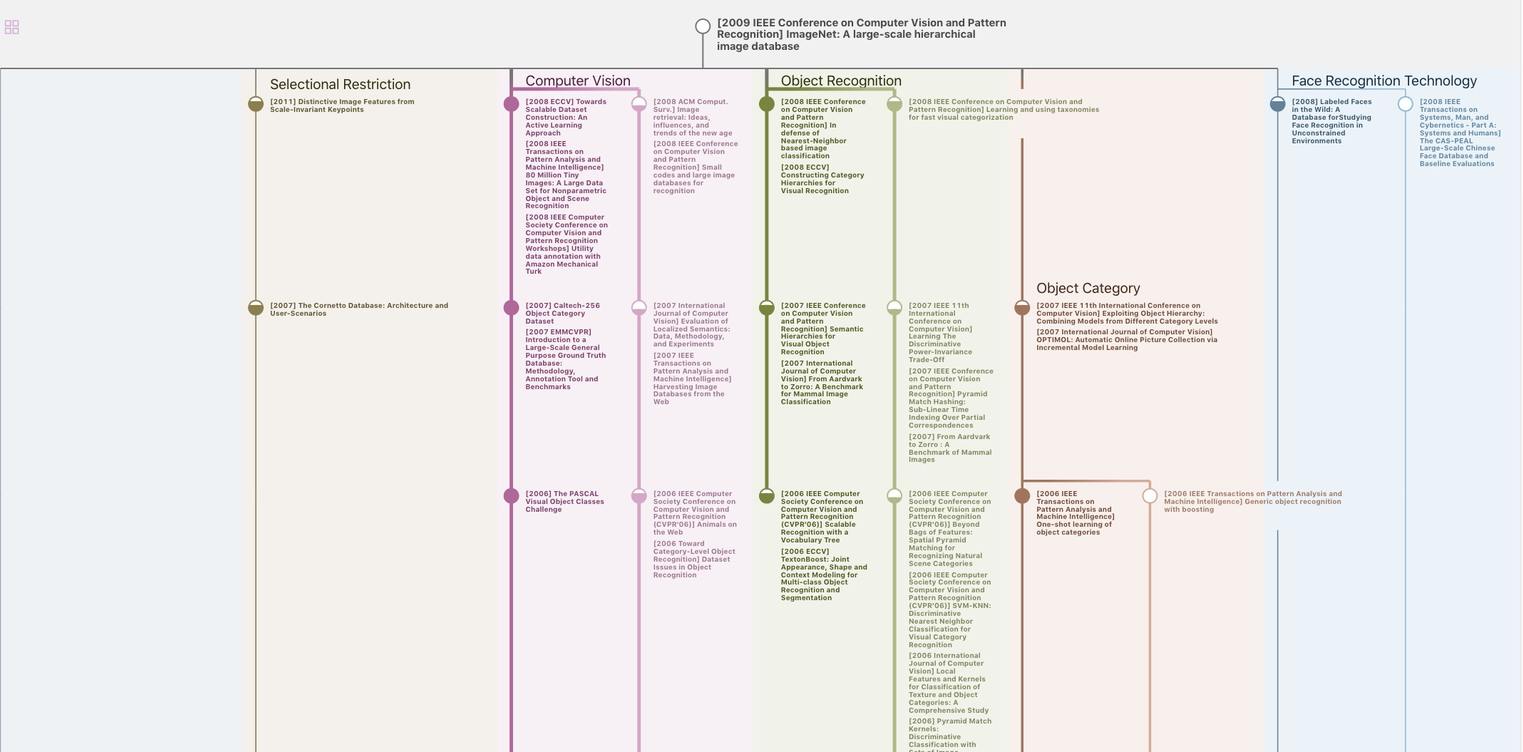
生成溯源树,研究论文发展脉络
Chat Paper
正在生成论文摘要