A new classification algorithm based on entropy and relative reduced extended concept lattice
Machine Learning and Cybernetics, 2004. Proceedings of 2004 International Conference(2004)
摘要
Classification is an important form of data analysis, and many algorithms have been proposed. entropy heuristics is an important method of rule learning, and it has been used in many fields. Concept lattice (also called Galois lattice) was proposed by R. Wilie in 1982, the equivalence relations to the intension of concepts and relative reduced intension [Hu Xuegang, 2000] was introduced to improve concept lattice to relative reduced extended concept lattice (RRECL). Due to the completeness of the RRECL, the cost of time and space is much larger in the construction of the RRECL. An EN-RRECL algorithm is presented in This work, which applies entropy theory to the RRECL to extract classification rules. The algorithm can simplify the RRECL's construction. From the simplified RRECL, we can extract classification rules which approximate to the one extracted from the not simplified the RRECL. The algorithm can cut the branches of the RRECL that are redundant for extracting classification rules, so the cost of time and space is reduced greatly. We also use an example to show the EN-RRECL algorithm's validity compared with the not simplified RRECL and show its completeness compared with ID3 algorithm.
更多查看译文
关键词
entropy theory,classification rules,pattern classification,data analysis,rule learning,relative reduced extended concept lattice,galois fields,data mining,entropy,entropy heuristics,galois lattice,equivalence relation
AI 理解论文
溯源树
样例
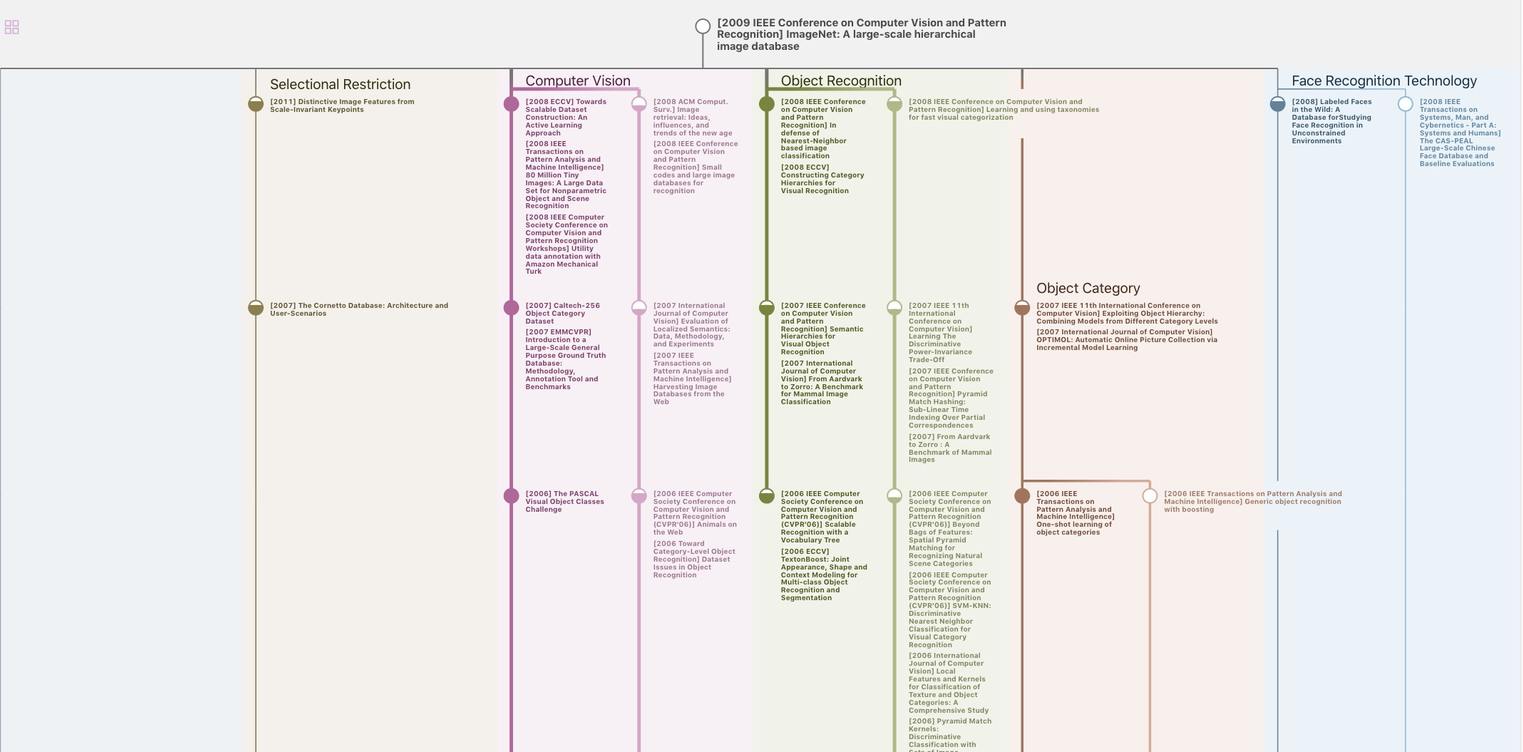
生成溯源树,研究论文发展脉络
Chat Paper
正在生成论文摘要