A multi-channel SSVEP-based brain-computer interface using a canonical correlation analysis in the frequency domain
Advances in Intelligent Systems and Computing(2014)
摘要
Brain-computer interface (BCI) is a new way for man-machine interaction with wide applications, in which steady-state visual evoked potentials (SSVEP) is a promising option. However, many characteristics of SSVEP show great user variation. So parameter optimization and channel selection for each subject were applied to improve the performance of BCI. These optimizations limit the practical applicability of the SSVEP-based BCI. The use of a canonical correlation analysis (CCA) method for multi-channel SSVEP in the time domain detection showed highly increased detection accuracy, but it is sensitive to the noise when the stimulate frequency is low. In this paper, a method of CCA in the frequency domain is presented for classifying multi-channel SSVEPs. First overlapping average is conducted on the original training signals. Then fast Fourier transform (FFT) is used to transform the signals from time domain to frequency domain to produce the reference data. Finally, according to the correlation coefficients of the new data and the references in the frequency domain, the SSVEP is classified. The experimental results show the enhanced accuracy of our method when applied to low stimulate frequencies. ? Springer-Verlag Berlin Heidelberg 2014.
更多查看译文
关键词
bci,cca in frequency domain,fft,overlapping average,ssvep
AI 理解论文
溯源树
样例
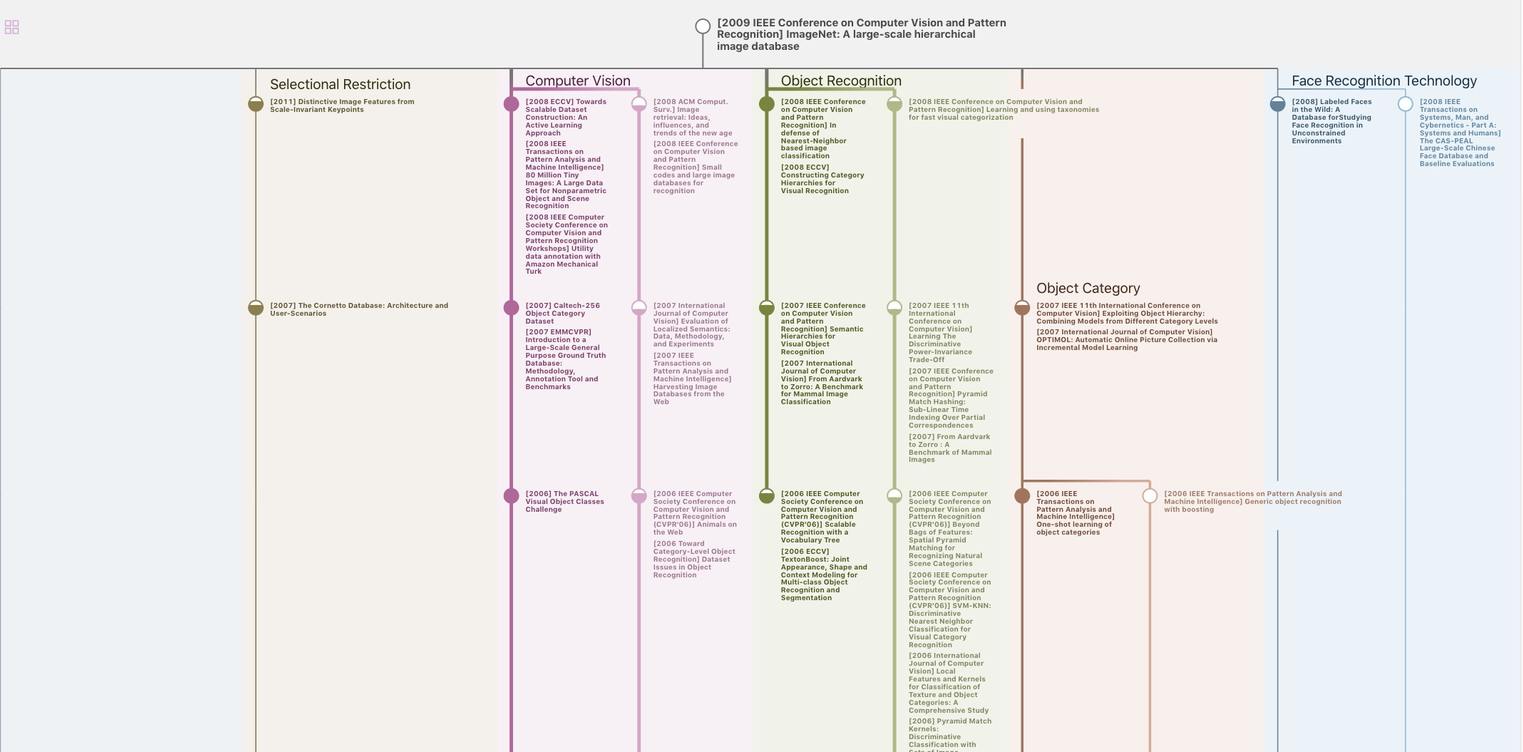
生成溯源树,研究论文发展脉络
Chat Paper
正在生成论文摘要