Consistency-preserving attribute reduction in fuzzy rough set framework
International Journal of Machine Learning and Cybernetics(2012)
摘要
Attribute reduction (feature selection) has become an important challenge in areas of pattern recognition, machine learning, data mining and knowledge discovery. Based on attribute reduction, one can extract fuzzy decision rules from a fuzzy decision table. As consistency is one of several criteria for evaluating the decision performance of a decision-rule set, in this paper, we devote to present a consistency-preserving attribute reduction in fuzzy rough set framework. Through constructing the membership function of an object, we first introduce a consistency measure to assess the consistencies of a fuzzy target set and a fuzzy decision table, which underlies a foundation for attribute reduction algorithm. Then, we derive two attribute significance measures based on the proposed consistency measure and design a forward greedy algorithm (ARBC) for attribute reduction from both numerical and nominal data sets. Numerical experiments show the validity of the proposed algorithm from search strategy and heuristic function in the meaning of consistency. Number of the selected features is the least for a given threshold of consistency measure.
更多查看译文
关键词
Fuzzy rough set,Fuzzy decision tables,Consistency measure,Attribute reduction
AI 理解论文
溯源树
样例
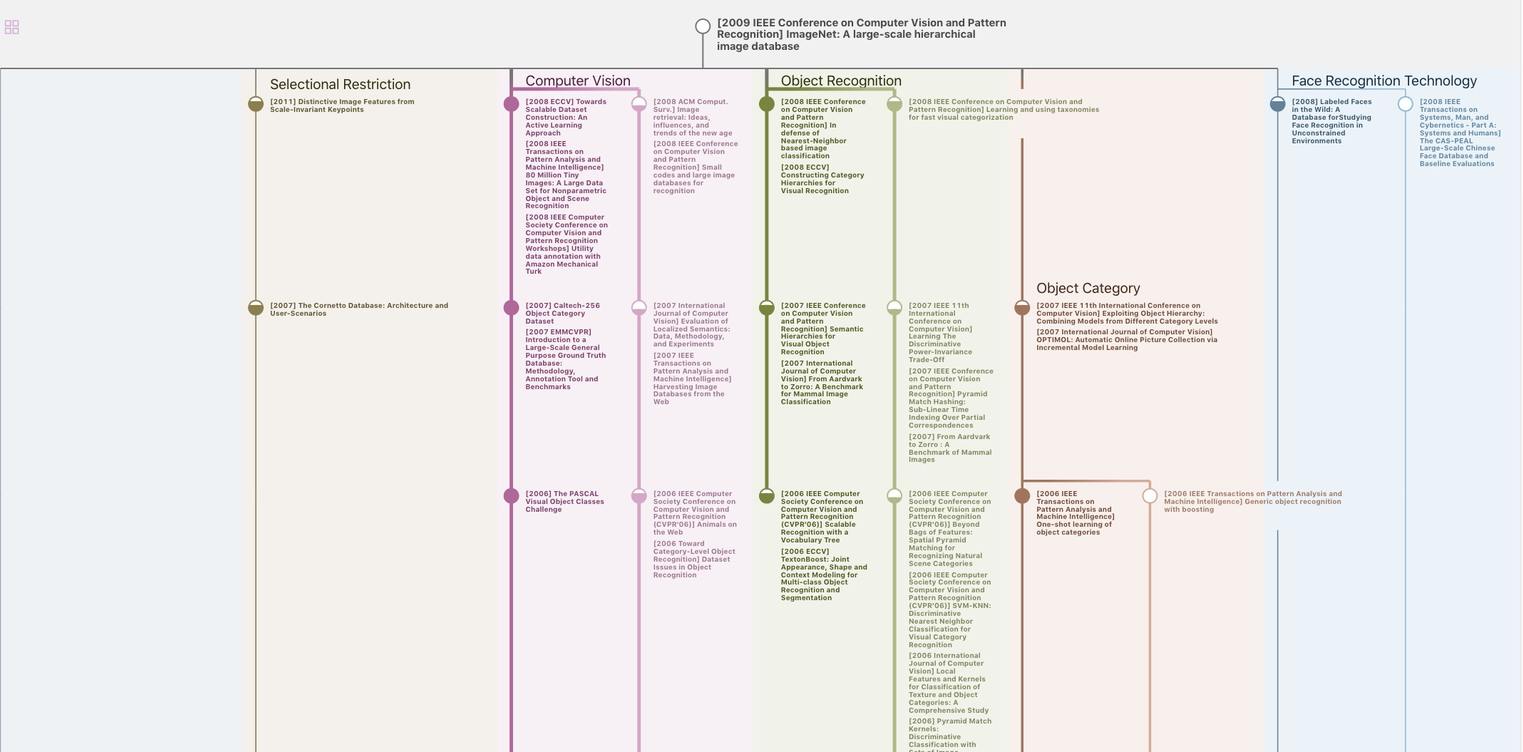
生成溯源树,研究论文发展脉络
Chat Paper
正在生成论文摘要