Iterative learning belief rule-base inference methodology using evidential reasoning for delayed coking unit
2011 International Symposium on Advanced Control of Industrial Processes, ADCONIP 2011(2012)
摘要
The belief rule-base inference methodology using evidential reasoning (RIMER) approach has been proved to be an effective extension of traditional rule-based expert systems and a powerful tool for representing more complicated causal relationships using different types of information with uncertainties. With a predetermined structure of the initial belief rule-base (BRB), the RIMER approach requires the assignment of some system parameters including rule weights, attribute weights, and belief degrees using experts' knowledge. Although some updating algorithms were proposed to solve this problem, it's still difficult to find an optimal compact BRB. In this paper, a novel updating algorithm is proposed based on iterative learning strategy for delayed coking unit (DCU), which contains both continuous and discrete characteristics. Daily DCU operations under different conditions are modeled by a BRB, which is then updated using iterative learning methodology, based on a novel statistical utility for every belief rule. Compared with the other learning algorithms, our methodology can lead to a more optimal compact final BRB. With the help of this expert system, a feed-forward compensation strategy is introduced to eliminate the disturbance caused by the drum-switching operations. The advantages of this approach are demonstrated through the developed DCU operation expert system modeled and optimized on the field data from a real oil refinery. © 2011 Zhejiang University.
更多查看译文
关键词
iterative learning,expert system,evidential reasoning
AI 理解论文
溯源树
样例
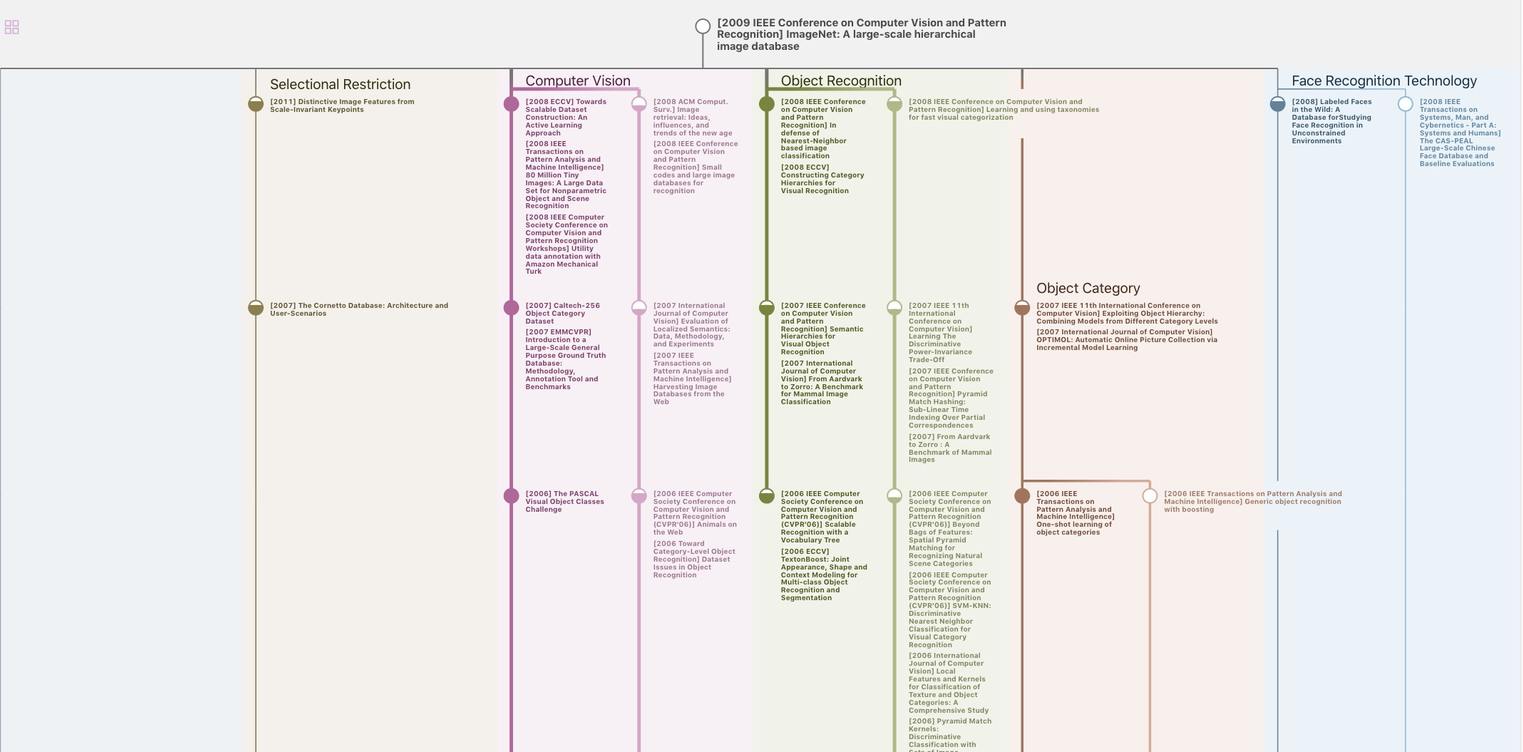
生成溯源树,研究论文发展脉络
Chat Paper
正在生成论文摘要