Fast object detection by regression in robot soccer
RoboCup 2009(2012)
摘要
Visual object detection in robot soccer is fundamental so the robots can act to accomplish their tasks. Current techniques rely on manually highly polished definitions of object models, that lead to accurate detection, but are quite often computationally inefficient. In this work, we contribute an efficient object detection through regression (ODR) method based on offline training. We build upon the observation that objects in robot soccer are of a well defined color and investigate an offline learning approach to model such objects. ODR consists of two main phases: (i) offline training, where the objects are automatically labeled offline by existing techniques, and (ii) online detection, where a given image is efficiently processed in real-time with the learned models. For each image, ODR determines whether the object is present and provides its position if so. We show comparing results with current techniques for precision and computational load.
更多查看译文
关键词
computational load,efficient object detection,offline training,offline learning approach,object model,robot soccer,current technique,visual object detection,accurate detection,online detection,computer vision
AI 理解论文
溯源树
样例
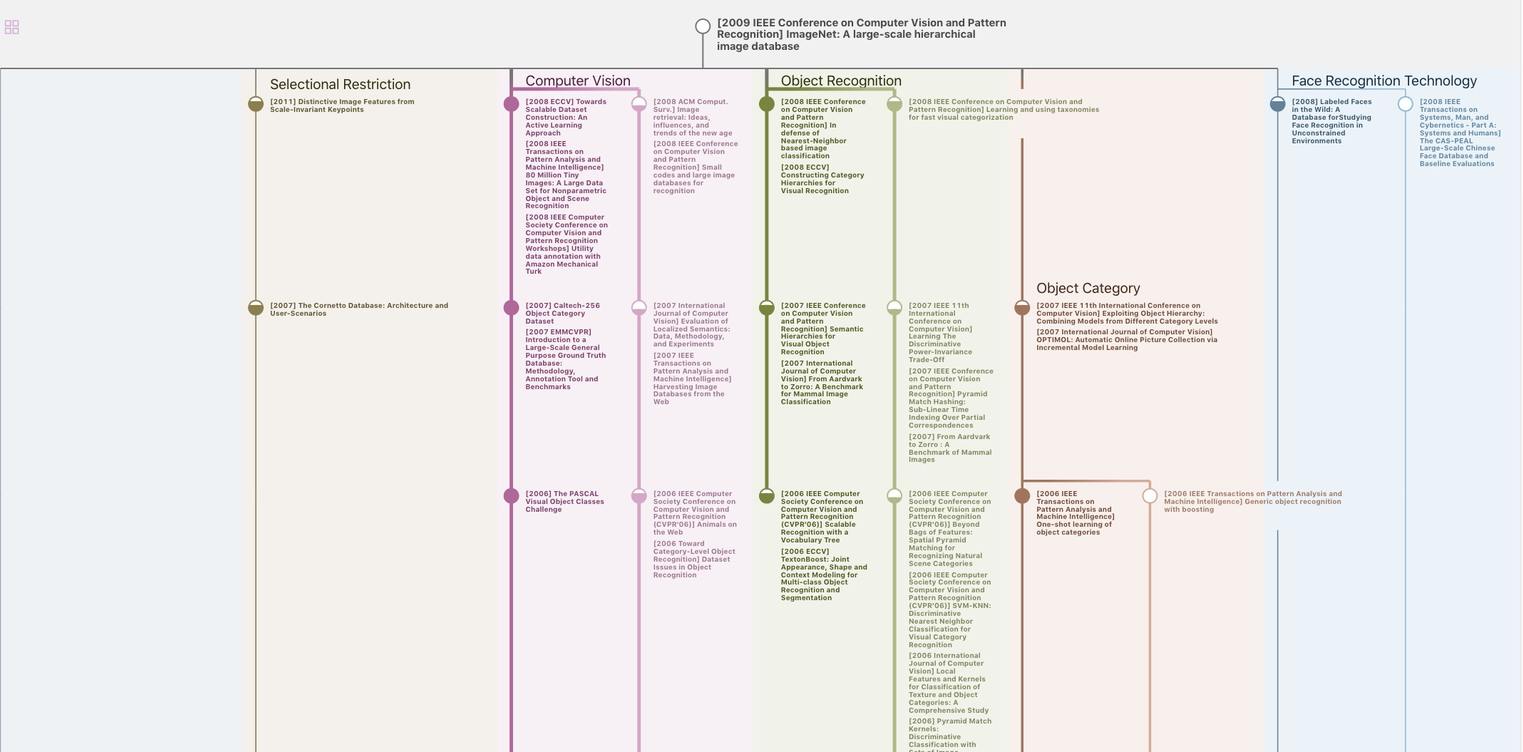
生成溯源树,研究论文发展脉络
Chat Paper
正在生成论文摘要