Clustering and indexing of experience sequences for popularity-driven recommendations
Proceedings of the 3rd ACM workshop on Continuous archival and retrival of personal experences(2006)
摘要
As part of our iCare efforts, we are developing mechanisms that provide guidance to individuals who are blind in diverse contexts. A fundamental challenge in this context is to represent and index experiences that can be used to provide recommendations. In this paper, we address the challenge of indexing experiences in order to retrieve them based on their popularities. In particular, we model experiences as sequences of propositional statements from a particular domain (daily life, web browsing, etc.). We then show that knowledge about domain constraints (such as commutativity between possible statements) need to be used for clustering and indexing experiences for popularity-search. We also highlight that don't cares (propositional statements not relevant to the user's query) make the task of popularity indexing challenging. Thus, we develop a canonical-sequence based approach that significantly reduces the experience sequence retrieval time in the presence of commutations. We introduce rule-compression, which helps achieve further reductions in the retrieval cost. We propose a novel two-level index structure, EXPdex, to efficiently answer wildcard (don't care) queries. We compare the proposed approach analytically and experimentally to a don't care-unaware solution, which does not take into account wildcards in queries while constructing the popularity index. Experiments show that the proposed approach provides large savings in retrieval time when commutations between the elements of sequences are allowed.
更多查看译文
关键词
indexation,web browsing
AI 理解论文
溯源树
样例
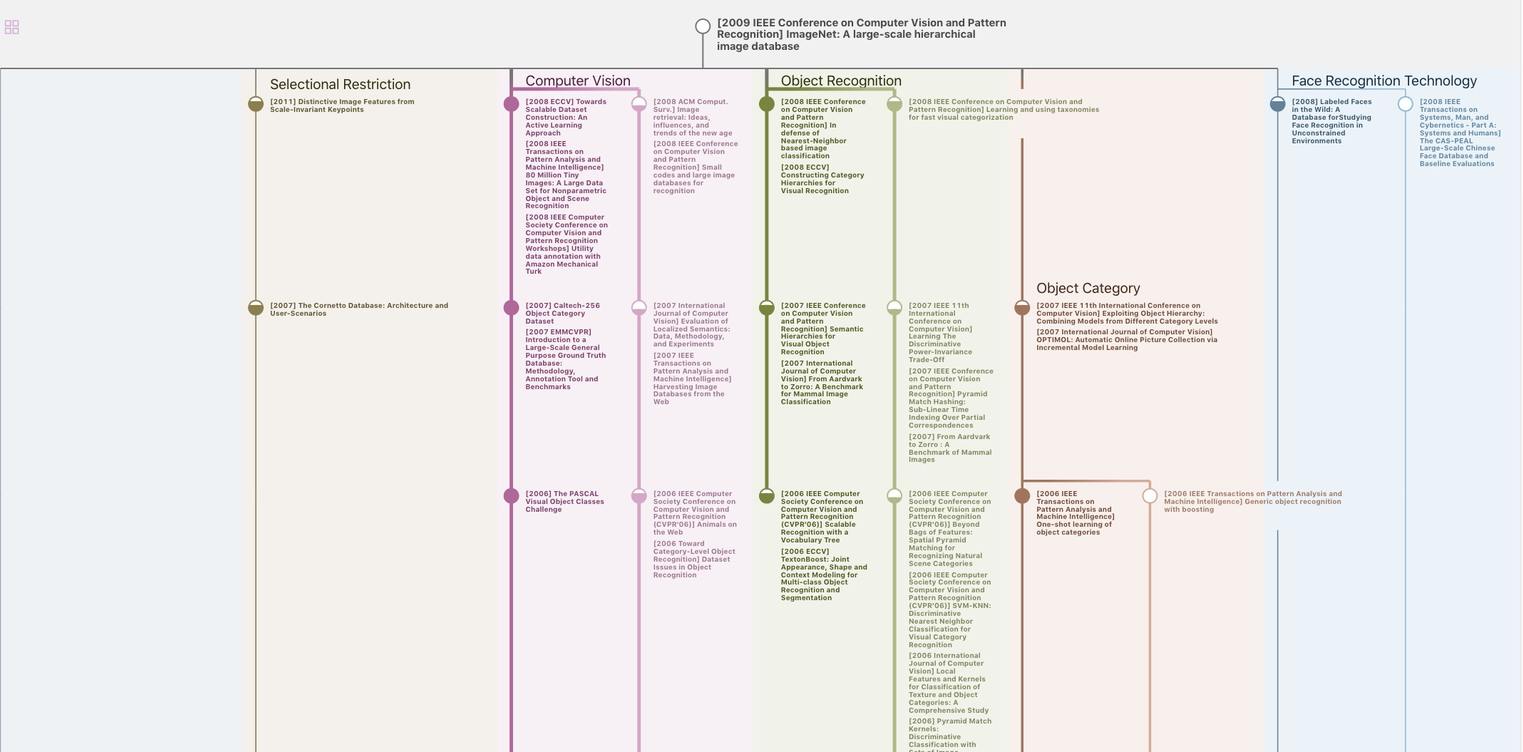
生成溯源树,研究论文发展脉络
Chat Paper
正在生成论文摘要