A new approach to query segmentation for relevance ranking in web search
Information Retrieval Journal(2015)
摘要
In this paper, we try to determine how best to improve state-of-the-art methods for relevance ranking in web searching by query segmentation. Query segmentation is meant to separate the input query into segments, typically natural language phrases. We propose employing the re-ranking approach in query segmentation, which first employs a generative model to create the top k candidates and then employs a discriminative model to re-rank the candidates to obtain the final segmentation result. The method has been widely utilized for structure prediction in natural language processing, but has not been applied to query segmentation, as far as we know. Furthermore, we propose a new method for using the results of query segmentation in relevance ranking, which takes both the original query words and the segmented query phrases as units of query representation. We investigate whether our method can improve three relevance models, namely n -gram BM25, key n -gram model and term dependency model, within the framework of learning to rank. Our experimental results on large scale web search datasets show that our method can indeed significantly improve relevance ranking in all three cases.
更多查看译文
关键词
Web search,Query segmentation,Relevance ranking,Query processing,Re-ranking,BM25,Term dependency model,Key n-gram extraction
AI 理解论文
溯源树
样例
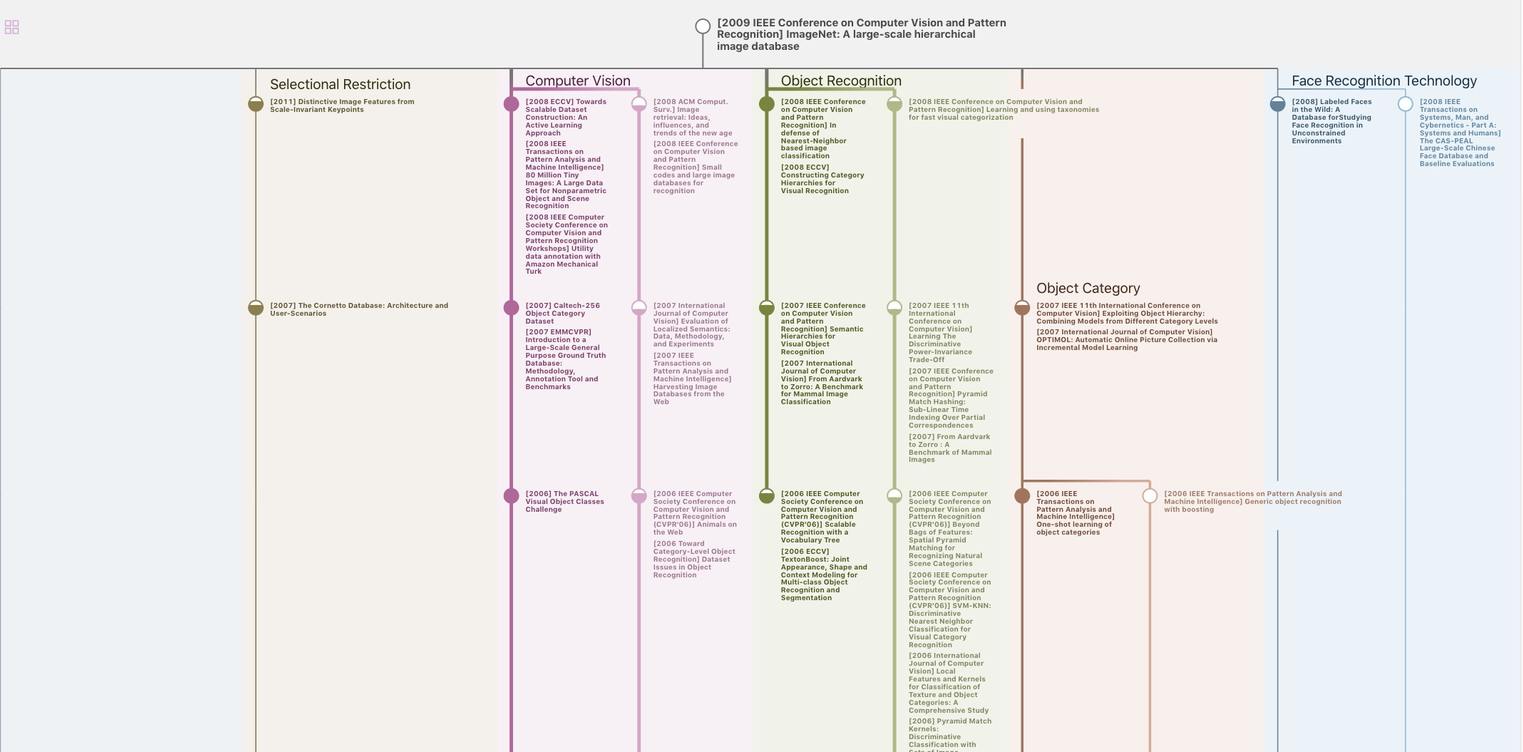
生成溯源树,研究论文发展脉络
Chat Paper
正在生成论文摘要