A New Mechanism for Eliminating Implicit Conflict in Graph Contrastive Learning
AAAI 2024(2024)
摘要
Graph contrastive learning (GCL) has attracted considerable attention because it can self-supervisedly extract low-dimensional representation of graph data. InfoNCE-based loss function is widely used in graph contrastive learning, which pulls the representations of positive pairs close to each other and pulls the representations of negative pairs away from each other. Recent works mainly focus on designing new augmentation methods or sampling strategies. However, we argue that the widely used InfoNCE-based methods may contain an implicit conflict which seriously confuses models when learning from negative pairs. This conflict is engendered by the encoder's message-passing mechanism and the InfoNCE loss function. As a result, the learned representations between negative samples cannot be far away from each other, compromising the model performance. To our best knowledge, this is the first time to report and analysis this conflict of GCL. To address this problem, we propose a simple but effective method called Partial ignored Graph Contrastive Learning (PiGCL). Specifically, PiGCL first dynamically captures the conflicts during training by detecting the gradient of representation similarities. It then enables the loss function to ignore the conflict, allowing the encoder to adaptively learn the ignored information without self-supervised samples. Extensive experiments demonstrate the effectiveness of our method.
更多查看译文
关键词
ML: Graph-based Machine Learning,DMKM: Graph Mining, Social Network Analysis & Community,ML: Unsupervised & Self-Supervised Learning
AI 理解论文
溯源树
样例
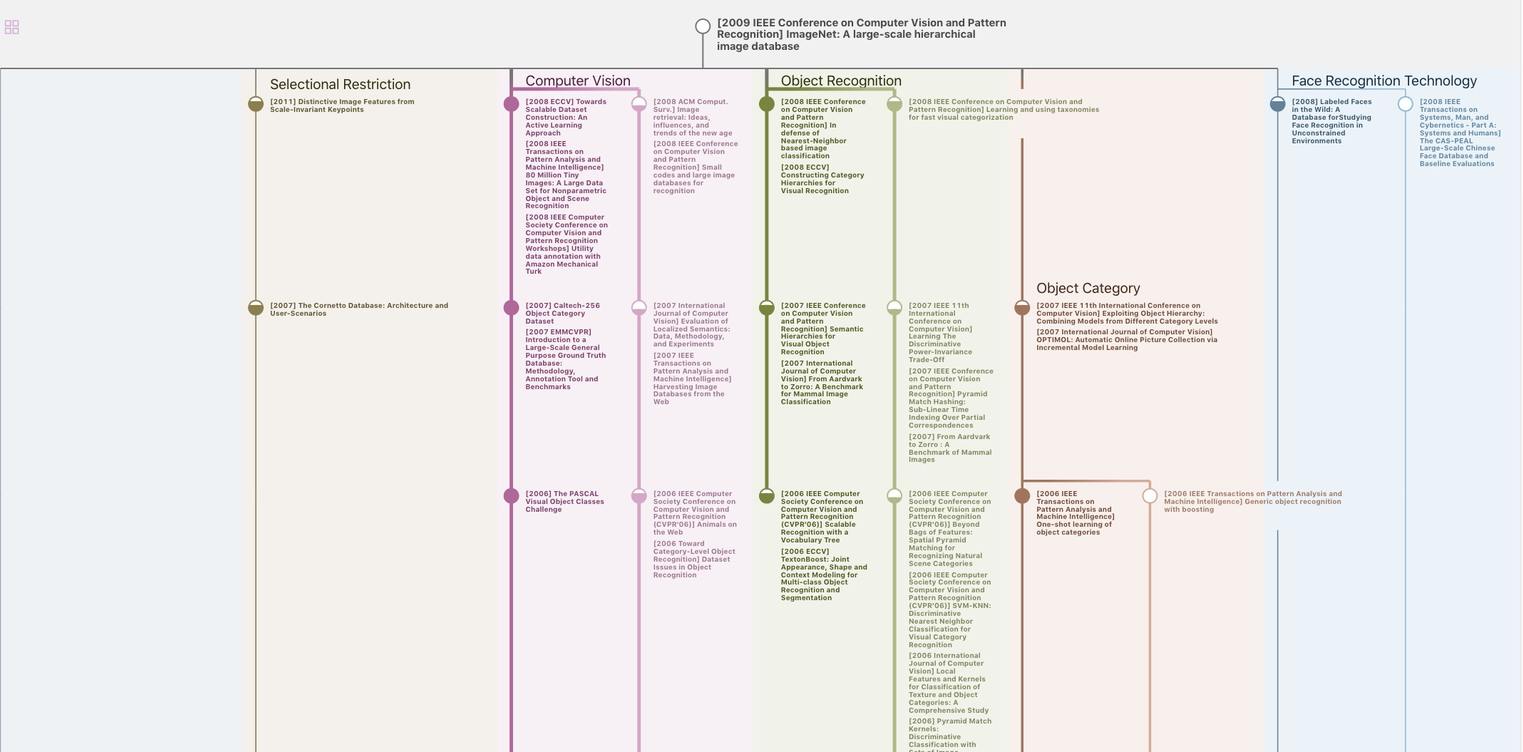
生成溯源树,研究论文发展脉络
Chat Paper
正在生成论文摘要