Model characterization curves for federated search using click-logs: predicting user engagement metrics for the span of feasible operating points.
WWW '11: 20th International World Wide Web Conference Hyderabad India March, 2011(2011)
摘要
Modern day federated search engines aggregate heterogeneous types of results from multiple vertical search engines and compose a single search engine result page (SERP). The search engine aggregates the results and produces one ranked list, constraining the vertical results to specific slots on the SERP.
The usual way to compare two ranking algorithms is to first fix their operating points (internal thresholds), and then run an online experiment that lasts multiple weeks. Online user engagement metrics are then compared to decide which algorithm is better. However, this method does not characterize and compare the behavior over the entire span of operating points. Furthermore, this time-consuming approach is not practical if we have to conduct the experiment over numerous operating points.
In this paper we propose a method of characterizing the performance of models that allows us to predict answers to "what if" questions about online user engagement using click-logs over the entire span of feasible operating points. We audition verticals at various slots on the SERP and generate click-logs. This log is then used to create operating curves between variables of interest (for example between result quality and click-through). The operating point for the system then can be chosen to achieve a specific trade-off between the variables. We apply this methodology to predict i) the online performance of two different models, ii) the impact of changing internal quality thresholds on clickthrough, iii) the behavior of introducing a new feature, iv) which machine learning loss function will give better online engagement, v) the impact of sampling distribution of head and tail queries in the training process. The results are reported on a well-known federated search engine. We validate the predictions with online experiments.
更多查看译文
AI 理解论文
溯源树
样例
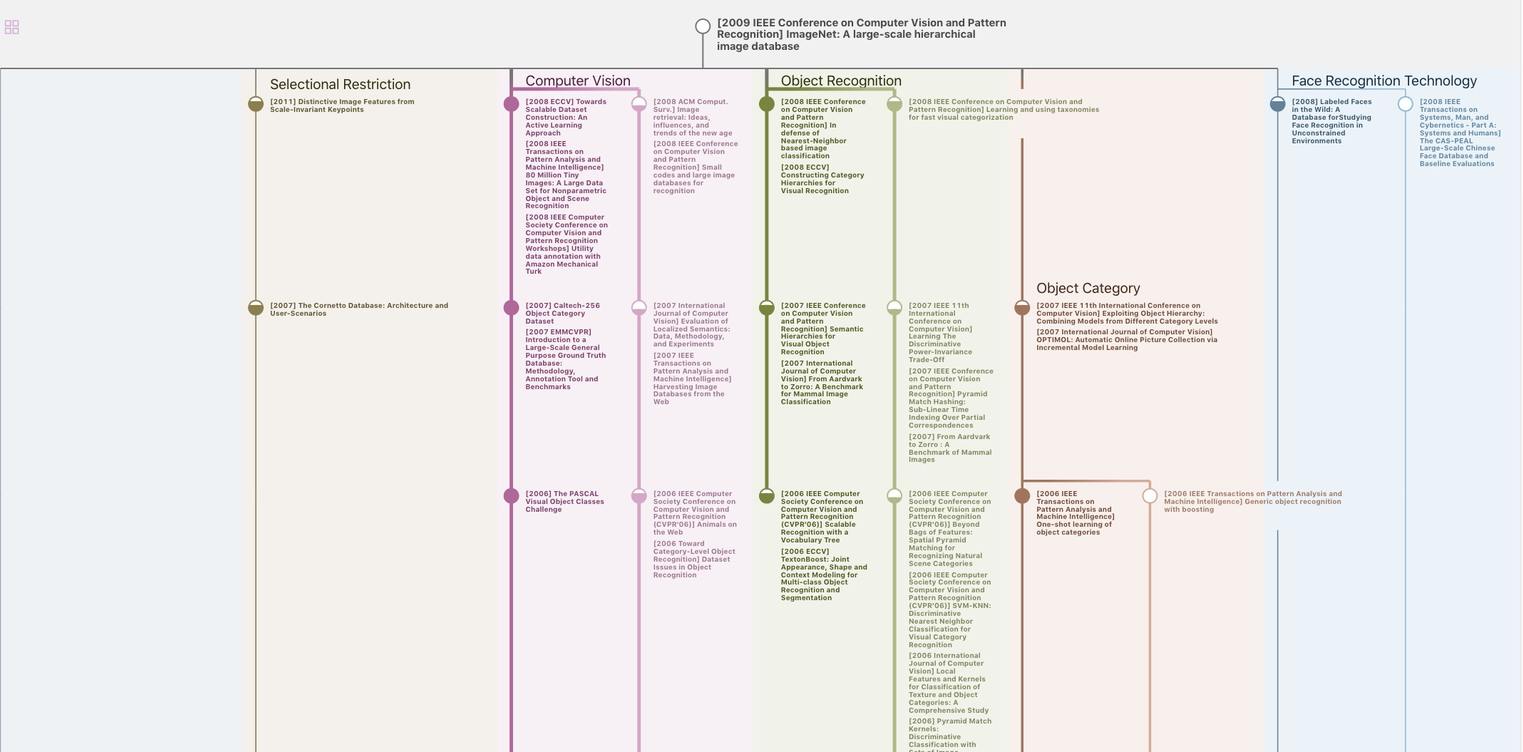
生成溯源树,研究论文发展脉络
Chat Paper
正在生成论文摘要