Random Sampling of a Continuous-time Stochastic Dynamical System
msra(2002)
摘要
We consider a dynamical system where the state equation is given by a linear stochastic dierential equation and noisy measurements occur at discrete times, in correspondence of the arrivals of a Poisson process. Such a system models a network of a large number of sensors that are not synchronized with one another, where the waiting time between two measurements is modelled by an exponential random vari- able. We formulate a Kalman Filter-based state estimation algorithm. The sequence of estimation error covariance matrices is not deterministic as for the ordinary Kalman Filter, but is a stochastic process itself: it is a homogeneous Markov process. In the one-dimensional case we compute a complete statistical description of this process: such a description depends on the Poisson sampling rate (which is proportional to the number of sensors on a network) and on the dynamics of the continuous-time system represented by the state equation. Finally, we have found a lower bound on the sam- pling rate that makes it possible to keep the estimation error variance below a given threshold with an arbitrary probability.
更多查看译文
关键词
system modeling,stochastic process,poisson process,lower bound,markov process,random sampling,discrete time,kalman filter,dynamic system
AI 理解论文
溯源树
样例
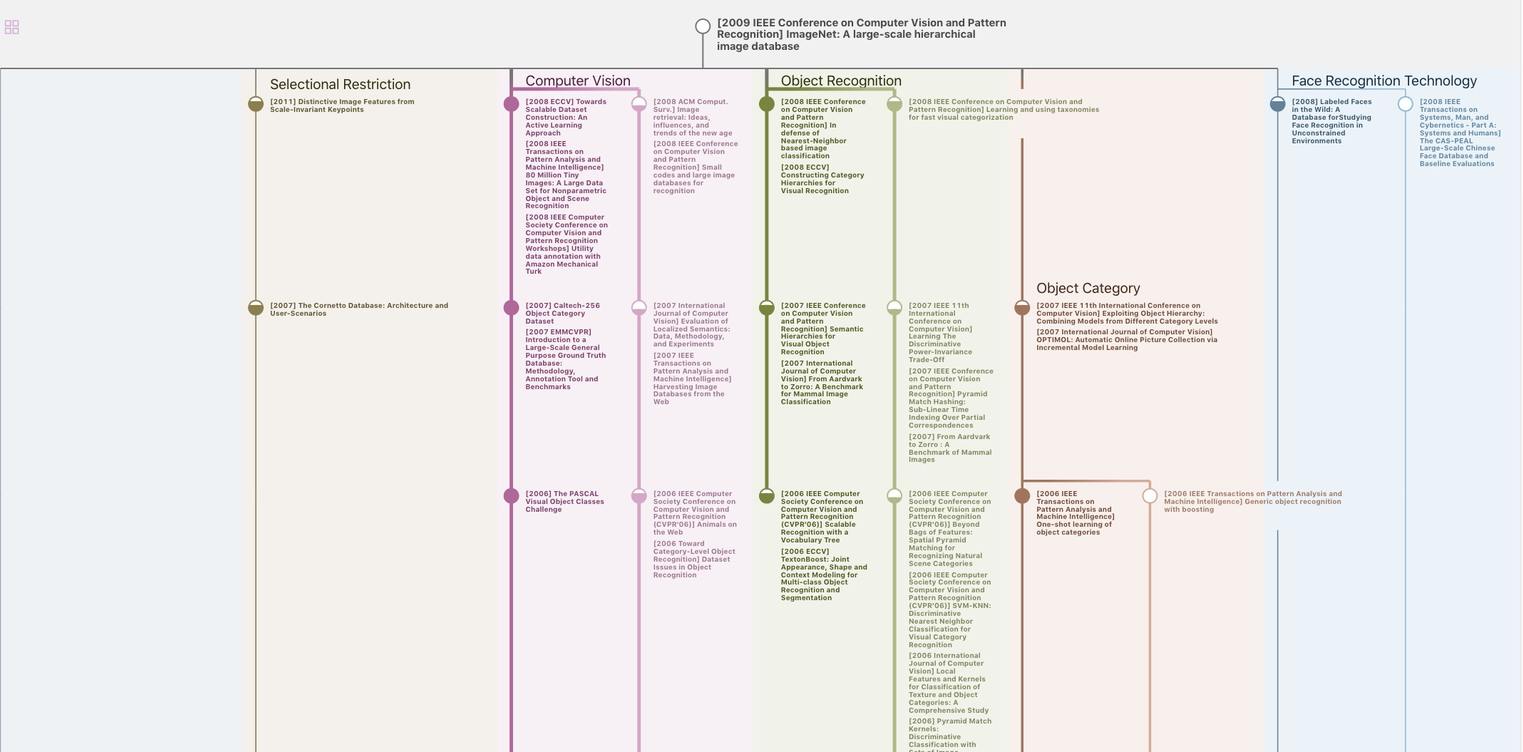
生成溯源树,研究论文发展脉络
Chat Paper
正在生成论文摘要