Part-based statistical models for visual object class recognition
Part-based statistical models for visual object class recognition(2008)
摘要
Object class recognition is a central problem of computer vision with broad potential application in image understanding, content-based retrieval, and surveillance. Unlike traditional object recognition where the goal is to detect specific objects, object class recognition aims to detect instances of broad object categories like cars, airplanes, bicycles, and people. This task is challenging because members of an object class may vary widely in appearance; for example, the “car” class includes many makes, models, styles and colors. In addition, class recognition must cope with usual sources of visual variation including viewpoint, illumination, and scale changes. In this thesis we describe a family of part-based probabilistic models for object class recognition. These models allow for efficient exact inference, unlike most other approaches which rely on feature detection and approximating heuristics to make inference tractable. The object models are hierarchical in nature, allowing evidence at multiple image scales to be combined in making recognition decisions. We show how the multiscale models can be augmented to incorporate local context from the surrounding scene. We also present learning algorithms of various degrees of supervision, including a weakly-supervised algorithm that requires only a set of images known to contain the object. Experimental results demonstrate state-of-the-art performance on challenging datasets of unconstrained consumer images.
更多查看译文
关键词
traditional object recognition,recognition decision,object model,Part-based statistical model,efficient exact inference,visual object class recognition,class recognition,specific object,broad potential application,broad object category,object class,object class recognition
AI 理解论文
溯源树
样例
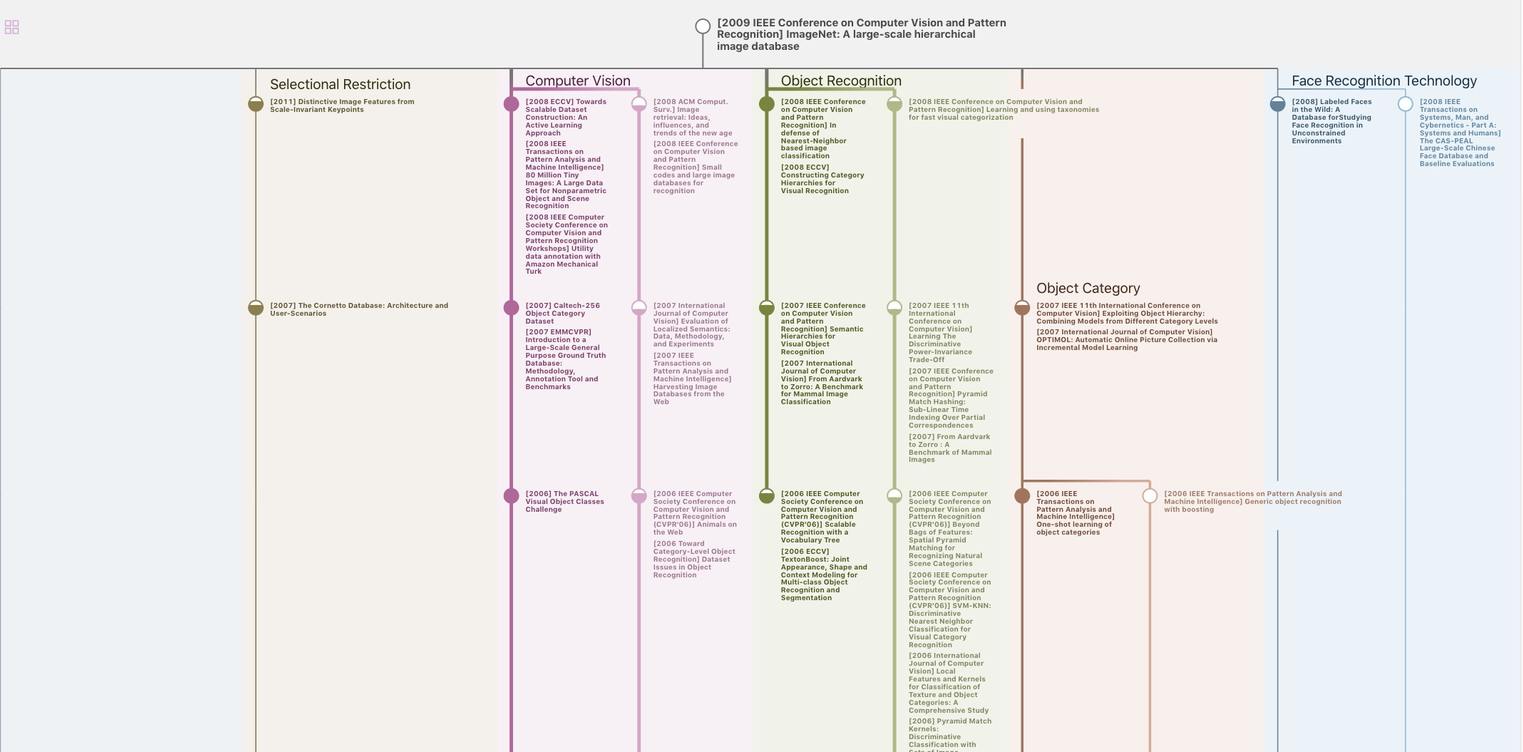
生成溯源树,研究论文发展脉络
Chat Paper
正在生成论文摘要